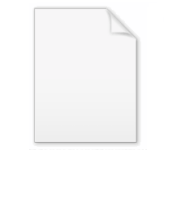
Nonlinear dimensionality reduction
Encyclopedia
High-dimensional data, meaning data that requires more than two or three dimensions to represent, can be difficult to interpret. One approach to simplification is to assume that the data of interest lies on an embedded non-linear manifold
within the higher-dimensional space. If the manifold is of low enough dimension then the data can be visualised in the low dimensional space.
Below is a summary of some of the important algorithms from the history of manifold learning and nonlinear dimensionality reduction. Many of these non-linear dimensionality reduction
methods are related to the linear methods listed below. Non-linear methods can be broadly classified into two groups: those that provide a mapping (either from the high dimensional space to the low dimensional embedding or vice versa), and those that just give a visualisation. In the context of machine learning
, mapping methods may be viewed as a preliminary feature extraction step, after which pattern recognition algorithms are applied. Typically those that just give a visualisation are based on proximity data - that is, distance
measurements.
. Reducing data into fewer dimensions often makes analysis algorithms more efficient, and can help machine learning algorithms make more accurate predictions.
Humans often have difficulty comprehending data in many dimensions. Thus, reducing data to a small number of dimensions is useful for visualization purposes.
The reduced-dimensional representations of data are often referred to as "intrinsic variables". This description implies that these are the values from which the data was produced. For example, consider a dataset that contains images of a letter 'A', which has been scaled and rotated by varying amounts. Each image has 32x32 pixels. Each image can be represented as a vector of 1024 pixel values. Each row is a sample on a two-dimensional manifold in 1024-dimensional space (a Hamming space
). The intrinsic dimensionality is two, because two variables (rotation and scale) were varied in order to produce the data. Information about the shape or look of a letter 'A' is not part of the intrinsic variables because it is the same in every instance. Nonlinear dimensionality reduction will discard the correlated information (the letter 'A') and recover only the varying information (rotation and scale). The image to the left shows sample images from this dataset (to save space, not all input images are shown), and a plot of the two-dimensional points that results from using a NLDR algorithm (in this case, Manifold Sculpting was used) to reduce the data into just two dimensions.
By comparison, if PCA (a linear dimensionality reduction algorithm) is used to reduce this same dataset into two dimensions, the resulting values are not so well organized. This demonstrates that the high-dimensional vectors (each representing a letter 'A') that sample this manifold vary in a non-linear manner.
It should be apparent, therefore, that NLDR has several applications in the field of computer-vision. For example, consider a robot that uses a camera to navigate in a closed static environment. The images obtained by that camera can be considered to be samples on a manifold in high-dimensional space, and the intrinsic variables of that manifold will represent the robot's position and orientation. This utility is not limited to robots. Dynamical systems, a more general class of systems, which includes robots, are defined in terms of a manifold. Active research in NLDR seeks to unfold the observation manifolds associated dynamical systems to develop techniques for modeling such systems and enable them to operate autonomously.
How to define the "simplicity" of the manifold is problem-dependent, however, it is commonly measured by the intrinsic dimensionality and/or the smoothness of the manifold. Usually, the principal manifold is defined as a solution to an optimization problem. The objective function includes a quality of data approximation and some penalty terms for the bending of the manifold. The popular initial approximations are generated by linear PCA, Kohonen's SOM or autoencoders. The elastic map
method provides the expectation-maximization algorithm
for principal manifold learning with minimization of quadratic energy functional at the "maximization" step.
is a feed-forward neural network
which is trained to approximate the identity function. That is, it is trained to map from a vector of values to the same vector. One of the hidden layers in the network, however, is limited to contain only a small number of network units. Thus, the network must learn to encode the vector into a small number of dimensions and then decode it back into the original space. Thus, the first half of the network is a model which maps from high to low-dimensional space, and the second half maps from low to high-dimensional space. Although the idea of autoencoders is quite old, training of the encoders has only recently become possible through the use of Restricted Boltzmann machine
s. Related to autoencoders is the NeuroScale algorithm, which uses stress functions inspired by multidimensional scaling
and Sammon mappings (see below) to learn a non-linear mapping from the high dimensional to the embedded space. The mappings in NeuroScale are based on radial basis function network
s.
). However in the GPLVM the mapping is from the embedded space to the data space (like density networks and GTM) whereas in kernel PCA it is in the opposite direction.
It should be noticed that CCA, as an iterative learning algorithm, actually starts with focus on large distances (like the Sammon algorithm), then gradually change focus to small distances. The small distance information will overwrite the large distance information, if compromises between the two have to be made.
The stress function of CCA has been proved as a sum of right Bregman divergence
. PCA begins by computing the covariance matrix of the
matrix 

It then projects the data onto the first k eigenvectors of that matrix. By comparison, KPCA begins by computing the covariance matrix of the data after being transformed into a higher-dimensional space,

It then projects the transformed data onto the first k eigenvectors of that matrix, just like PCA. It uses the kernel trick to factor away much of the computation, such that the entire process can be performed without actually computing
. Of course
must be chosen such that it has a known corresponding kernel. Unfortunately, it is not trivial to find a good kernel for a given problem, so KPCA does not yield good results with some problems. For example, it is known to perform poorly with the swiss roll
manifold.
KPCA has an internal model, so it can be used to map points onto its embedding that were not available at training time.
is a combination of the Floyd-Warshall algorithm
with classic Multidimensional Scaling
. Classic Multidimensional Scaling (MDS) takes a matrix of pair-wise distances between all points, and computes a position for each point. With NLDR algorithms like Isomap, however, the pair-wise distances are only known between neighboring points. So Isomap uses the Floyd-Warshall algorithm to compute the pair-wise distances between all of the other points. This effectively estimates the full matrix of pair-wise geodesic distances between all of the points. Isomap then uses classic MDS to compute the reduced-dimensional positions of all the points.
Landmark-Isomap is a variant of this algorithm that uses landmarks to increase speed, at the cost of some accuracy.
LLE computes the barycentric coordinates of a point Xi based on its neighbors Xj. The original point is reconstructed by a linear combination, given by the weight matrix Wij, of its neighbors. The reconstruction error is given by the cost function E(W).

The weights Wij refer to the amount of contribution the point Xj has while reconstructing the point Xi. The cost function is minimized under 2 constraints:
(a) Each data point Xi is reconstructed only from its neighbors, thus enforcing Wij to be zero if point Xj is not a neighbor of the point Xi and
(b) The sum of every row of the weight matrix equals 1.

The original data points are collected in a D dimensional space and the goal of the algorithm is to reduce the dimensionality to d such that D >> d. The same weights Wij that reconstructs the i th data point in the D dimensional space will be used to reconstruct the same point in the lower d dimensional space. A neighborhood preserving map is created based on this idea. Each point Xi in the D dimensional space is mapped onto a point Yi in the d dimensional space by minimizing the cost function

In this cost function unlike the previous one the weights Wij are kept fixed and the minimization is done on the points Yi to optimize the coordinates. This minimization problem can be solved by solving a sparse N X N eigen value problem, whose bottom d nonzero eigen vectors provide an orthogonal set of coordinates. Generally the data points are reconstructed from K nearest neighbors, as measured by Euclidean distance
. For such an implementation the algorithm has only one free parameter K, which can be chosen by cross validation.
regularization exist for adding this capability. Such techniques can be applied to other nonlinear dimensionality reduction algorithms as well.
Traditional techniques like Principal Component Analysis do not consider the intrinsic geometry of the data. Laplacian Eigenmaps builds a graph from neighborhood information of the data set. Each data point serves as a node on the graph and connectivity between nodes is governed by the proximity of neighboring points (using e.g. the k-nearest neighbor algorithm
). The graph thus generated can be considered as a discrete approximation of the low dimensional manifold in the high dimensional space. Minimization of a cost function based on the graph ensures that points close to each other on the manifold are mapped close to each other in the low dimensional space, preserving local distances. The eigenfunctions of the Laplace-Beltrami operator
on the manifold serve as the embedding dimensions, since under mild conditions this operator has a countable spectrum that is a basis for square integrable functions on the manifold (compare to Fourier series
on the unit circle manifold). Attempts to place Laplacian eigenmaps on solid theoretical ground have met with some success, as under certain nonrestrictive assumptions, the graph Laplacian matrix has been shown to converge to the Laplace-Beltrami operator as the number of points goes to infinity. Matlab code for Laplacian Eigenmaps can be found in algorithms and the PhD thesis of Belkin can be found at the Ohio State University
.
); an analogy is drawn between the diffusion operator on a manifold and a Markov transition matrix operating on functions defined on the graph whose nodes were sampled from the manifold.. In particular let a data set be represented by
. The underlying assumption of diffusion map is that the data although high dimensional lies on a low dimensional manifold of dimensions
.X represents the data set and let
represent the distribution of the data points on X. In addition to this lets define a kernel which represents some notion of affinity of the points in X. The kernel
has the following properties
, k is symmetric
x,y.
is positivity preserving
Thus one can think of the individual data points as the nodes of a graph and the kernel k defining some sort of affinity on that graph. The graph is symmetric by construction since the kernel is symmetric. It is easy to see here that from the tuple {X,k} one can construct a reversible Markov Chain
. This technique is fairly popular in a variety of fields and is known as the graph laplacian.
The graph K = (X,E) can be constructed for example using a Gaussian kernel.

In this above equation
denotes that
is a nearest neighbor of
. In reality Geodesic
distance should be used to actually measure distances on the manifold
. Since the exact structure of the manifold is not available, the geodesic distance is approximated by euclidean distances with only nearest neighbors. The choice
modulates our notion of proximity in the sense that if
then
and if
then
. The former means that very little diffusion has taken place while the latter implies that the diffusion process is nearly complete. Different strategies to choose
can be found in . If
has to faithfully represent a Markov Matrix, then it has to be normalized by the corresponding degree matrix
.

now represents a Markov chain.
is the probability of transitioning from
to
in one a time step. Similarly the probability of transitioning from
to
in t time steps is given by
. Here
is the matrix K multiplied to itself t times. Now the kernel markov matrix K constitutes some notion of local geometry of the data set X. The major difference between diffusion maps and principal component analysis is that only local features of the data is considered in diffusion maps as opposed to taking correlations of the entire data set.
defines a random walk on the data set which means that the kernel captures some local geometry of data set. The markov chain defines fast and slow directions of propagation , based on the values taken by the kernel, and as one propagates the walk forward in time, the local geometry information is being aggregated in the same way as local transitions (defined by differential equations) of the dynamical system . The concept of diffusion arises from the definition of a family diffusion distance {
}

For a given value of t
defines a distance between any two points of the data set. This means that the value of
will be small if there are many paths that connect x to y and vice versa. The quantity
involves summing over of all paths of length t, as a result of which
is extremely robust to noise in the data as opposed to geodesic distance.
takes into account all the relation between points x and y while calculating the distance and serves as a better notion of proximity than just Euclidean distance
or even geodesic distance.
is based on the intuition that when a manifold is correctly unfolded, all of the tangent hyperplanes to the manifold will become aligned. It begins by computing the k-nearest neighbors of every point. It computes the tangent space at every point by computing the d-first principal components in each local neighborhood. It then optimizes to find an embedding that aligns the tangent spaces.
to train a multi-layer perceptron to fit to a manifold. Unlike typical MLP training, which only updates the weights, NLPCA updates both the weights and the inputs. That is, both the weights and inputs are treated as latent values. After training, the latent inputs are a low-dimensional representation of the observed vectors, and the MLP maps from that low-dimensional representation to the high-dimensional observation space.
phenomenon by adapting the weighting function to the distance distribution.
Data-Driven High Dimensional Scaling (DD-HDS) is closely related to Sammon's mapping and curvilinear component analysis except that (1) it simultaneously penalizes false neighborhoods and tears by focusing on small distances in both original and output space, and that (2) it accounts for concentration of measure
phenomenon by adapting the weighting function to the distance distribution.
to find an embedding. Like other algorithms, it computes the k-nearest neighbors and tries to seek an embedding that preserves relationships in local neighborhoods. It slowly scales variance out of higher dimensions, while simultaneously adjusting points in lower dimensions to preserve those relationships. If the rate of scaling is small, it can find very precise embeddings. It boasts higher empirical accuracy than other algorithms with several problems. It can also be used to refine the results from other manifold learning algorithms. It struggles to unfold some manifolds, however, unless a very slow scaling rate is used. It has no model.
that follows.
algorithm. The algorithm finds a configuration of data points on a manifold by simulating a multi-particle dynamic system on a closed manifold, where data points are mapped to particles and distances (or dissimilarity) between data points are used as strength of a kind of repulsive force. As the manifold gradually grows in size the multi-particale system cools down gradually and converges to configuration that reflects the distance information of the data points.
Relational perspective map is initially inspired by the physic system in which positively charged particles freely move on the surface of a ball. Guided by Coulumbian repulsive force between particles, the minimal energy configuration of the particles will reflect the strength of repulsive forces between the particles.
Relational perspective map is introduced in.
The algorithm firstly used the flat torus
as the image manifold, then it has been extended (in the software VisuMap to use other types of closed manifolds, like sphere
, projective space
and klein-bottle, as image manifolds.
or a distance matrix
. These methods all fall under the broader class of metric multidimensional scaling. The variations tend to be differences in how the proximity data is computed; for example, Isomap
, locally linear embeddings, maximum variance unfolding, and Sammon mapping
(which is not in fact a mapping) are examples of metric multidimensional scaling methods.
Manifold
In mathematics , a manifold is a topological space that on a small enough scale resembles the Euclidean space of a specific dimension, called the dimension of the manifold....
within the higher-dimensional space. If the manifold is of low enough dimension then the data can be visualised in the low dimensional space.
Below is a summary of some of the important algorithms from the history of manifold learning and nonlinear dimensionality reduction. Many of these non-linear dimensionality reduction
Dimensionality reduction
In machine learning, dimension reduction is the process of reducing the number of random variables under consideration, and can be divided into feature selection and feature extraction.-Feature selection:...
methods are related to the linear methods listed below. Non-linear methods can be broadly classified into two groups: those that provide a mapping (either from the high dimensional space to the low dimensional embedding or vice versa), and those that just give a visualisation. In the context of machine learning
Machine learning
Machine learning, a branch of artificial intelligence, is a scientific discipline concerned with the design and development of algorithms that allow computers to evolve behaviors based on empirical data, such as from sensor data or databases...
, mapping methods may be viewed as a preliminary feature extraction step, after which pattern recognition algorithms are applied. Typically those that just give a visualisation are based on proximity data - that is, distance
Distance
Distance is a numerical description of how far apart objects are. In physics or everyday discussion, distance may refer to a physical length, or an estimation based on other criteria . In mathematics, a distance function or metric is a generalization of the concept of physical distance...
measurements.
Linear methods
- Independent component analysisIndependent component analysisIndependent component analysis is a computational method for separating a multivariate signal into additive subcomponents supposing the mutual statistical independence of the non-Gaussian source signals...
(ICA). - Principal component analysis (PCA) (also called Karhunen–Loève transform — KLT).
- Singular value decompositionSingular value decompositionIn linear algebra, the singular value decomposition is a factorization of a real or complex matrix, with many useful applications in signal processing and statistics....
(SVD). - Factor analysisFactor analysisFactor analysis is a statistical method used to describe variability among observed, correlated variables in terms of a potentially lower number of unobserved, uncorrelated variables called factors. In other words, it is possible, for example, that variations in three or four observed variables...
.
Uses for NLDR
Consider a dataset represented as a matrix (or a database table), such that each row represents a set of attributes (or features or dimensions) that describe a particular instance of something. If the number of attributes is large, then the space of unique possible rows is exponentially large. Thus, the larger the dimensionality, the more difficult it becomes to sample the space. This causes many problems. Algorithms that operate on high-dimensional data tend to have a very high time complexity. Many machine learning algorithms, for example, struggle with high-dimensional data. This has become known as the curse of dimensionalityCurse of dimensionality
The curse of dimensionality refers to various phenomena that arise when analyzing and organizing high-dimensional spaces that do not occur in low-dimensional settings such as the physical space commonly modeled with just three dimensions.There are multiple phenomena referred to by this name in...
. Reducing data into fewer dimensions often makes analysis algorithms more efficient, and can help machine learning algorithms make more accurate predictions.
Humans often have difficulty comprehending data in many dimensions. Thus, reducing data to a small number of dimensions is useful for visualization purposes.
The reduced-dimensional representations of data are often referred to as "intrinsic variables". This description implies that these are the values from which the data was produced. For example, consider a dataset that contains images of a letter 'A', which has been scaled and rotated by varying amounts. Each image has 32x32 pixels. Each image can be represented as a vector of 1024 pixel values. Each row is a sample on a two-dimensional manifold in 1024-dimensional space (a Hamming space
Hamming space
In statistics and coding theory, a Hamming space is the set of all 2^N binary strings of length N. It is used in the theory of coding signals and transmission. Hamming codes and Hamming distance are related concepts....
). The intrinsic dimensionality is two, because two variables (rotation and scale) were varied in order to produce the data. Information about the shape or look of a letter 'A' is not part of the intrinsic variables because it is the same in every instance. Nonlinear dimensionality reduction will discard the correlated information (the letter 'A') and recover only the varying information (rotation and scale). The image to the left shows sample images from this dataset (to save space, not all input images are shown), and a plot of the two-dimensional points that results from using a NLDR algorithm (in this case, Manifold Sculpting was used) to reduce the data into just two dimensions.
By comparison, if PCA (a linear dimensionality reduction algorithm) is used to reduce this same dataset into two dimensions, the resulting values are not so well organized. This demonstrates that the high-dimensional vectors (each representing a letter 'A') that sample this manifold vary in a non-linear manner.
It should be apparent, therefore, that NLDR has several applications in the field of computer-vision. For example, consider a robot that uses a camera to navigate in a closed static environment. The images obtained by that camera can be considered to be samples on a manifold in high-dimensional space, and the intrinsic variables of that manifold will represent the robot's position and orientation. This utility is not limited to robots. Dynamical systems, a more general class of systems, which includes robots, are defined in terms of a manifold. Active research in NLDR seeks to unfold the observation manifolds associated dynamical systems to develop techniques for modeling such systems and enable them to operate autonomously.
Manifold learning algorithms
Some of the more prominent manifold learning algorithms are listed below (in approximately chronological order). An algorithm may learn an internal model of the data, which can be used to map points unavailable at training time into the embedding in a process often called out-of-sample extension.Kohonen Maps
Kohonen maps (also called self-organizing maps or SOM) and its probabilistic variant generative topographic mapping (GTM) use a point representation in the embedded space to form a latent variable model based on a non-linear mapping from the embedded space to the high dimensional space. These techniques are related to work on density networks, which also are based around the same probabilistic model.Principal curves and manifolds
Principal curves and manifolds give the natural geometric framework for nonlinear dimensionality reduction and extend the geometric interpretation of PCA by explicitly constructing an embedded manifold, and by encoding using standard geometric projection onto the manifold. This approach was proposed by Trevor Hastie in his thesis (1984) and developed further by many authors.How to define the "simplicity" of the manifold is problem-dependent, however, it is commonly measured by the intrinsic dimensionality and/or the smoothness of the manifold. Usually, the principal manifold is defined as a solution to an optimization problem. The objective function includes a quality of data approximation and some penalty terms for the bending of the manifold. The popular initial approximations are generated by linear PCA, Kohonen's SOM or autoencoders. The elastic map
Elastic map
Elastic maps provide a tool for nonlinear dimensionality reduction. By their construction, they are system of elastic springs embedded in the dataspace. This system approximates a low-dimensional manifold...
method provides the expectation-maximization algorithm
Expectation-maximization algorithm
In statistics, an expectation–maximization algorithm is an iterative method for finding maximum likelihood or maximum a posteriori estimates of parameters in statistical models, where the model depends on unobserved latent variables...
for principal manifold learning with minimization of quadratic energy functional at the "maximization" step.
Autoencoders
An autoencoderAutoencoder
An auto-encoder is an artificial neural network used for learning efficient codings.The aim of an auto-encoder is to learn a compressed representation for a set of data....
is a feed-forward neural network
Neural network
The term neural network was traditionally used to refer to a network or circuit of biological neurons. The modern usage of the term often refers to artificial neural networks, which are composed of artificial neurons or nodes...
which is trained to approximate the identity function. That is, it is trained to map from a vector of values to the same vector. One of the hidden layers in the network, however, is limited to contain only a small number of network units. Thus, the network must learn to encode the vector into a small number of dimensions and then decode it back into the original space. Thus, the first half of the network is a model which maps from high to low-dimensional space, and the second half maps from low to high-dimensional space. Although the idea of autoencoders is quite old, training of the encoders has only recently become possible through the use of Restricted Boltzmann machine
Boltzmann machine
A Boltzmann machine is a type of stochastic recurrent neural network invented by Geoffrey Hinton and Terry Sejnowski. Boltzmann machines can be seen as the stochastic, generative counterpart of Hopfield nets...
s. Related to autoencoders is the NeuroScale algorithm, which uses stress functions inspired by multidimensional scaling
Multidimensional scaling
Multidimensional scaling is a set of related statistical techniques often used in information visualization for exploring similarities or dissimilarities in data. MDS is a special case of ordination. An MDS algorithm starts with a matrix of item–item similarities, then assigns a location to each...
and Sammon mappings (see below) to learn a non-linear mapping from the high dimensional to the embedded space. The mappings in NeuroScale are based on radial basis function network
Radial basis function network
A radial basis function network is an artificial neural network that uses radial basis functions as activation functions. It is a linear combination of radial basis functions...
s.
Gaussian process latent variable models
Gaussian process latent variable models (GPLVM) are a probabilistic non-linear PCA. Like kernel PCA they use a kernel function to form the mapping (in the form of a Gaussian processGaussian process
In probability theory and statistics, a Gaussian process is a stochastic process whose realisations consist of random values associated with every point in a range of times such that each such random variable has a normal distribution...
). However in the GPLVM the mapping is from the embedded space to the data space (like density networks and GTM) whereas in kernel PCA it is in the opposite direction.
Curvilinear component analysis
Curvilinear component analysis (CCA) looks for the configuration of points in the output space that preserves original distances as much as possible while focusing on small distances in the output space (conversely to Sammon's mapping which focus on small distances in original space).It should be noticed that CCA, as an iterative learning algorithm, actually starts with focus on large distances (like the Sammon algorithm), then gradually change focus to small distances. The small distance information will overwrite the large distance information, if compromises between the two have to be made.
The stress function of CCA has been proved as a sum of right Bregman divergence
Curvilinear Distance Analysis
CDA trains a self-organizing neural network to fit the manifold and seeks to preserve geodesic distances in its embedding. It is based on Curvilinear Component Analysis (which extended Sammon's mapping), but uses geodesic distances instead.Diffeomorphic Dimensionality Reduction
Diffeomorphic Dimensionality Reduction or Diffeomap learns a smooth diffeomorphic mapping which transports the data onto a lower dimensional linear subspace. The methods solves for a smooth time indexed vector field such that path integrals along the field which start at the data points will end at a lower dimensional linear subspace, thereby attempting to preserve pairwise differences under both the forward and inverse mapping.Kernel Principal Component Analysis
Perhaps the most widely used algorithm for manifold learning is kernel PCA. It is a combination of Principal component analysis and the kernel trickKernel trick
For machine learning algorithms, the kernel trick is a way of mapping observations from a general set S into an inner product space V , without ever having to compute the mapping explicitly, in the hope that the observations will gain meaningful linear structure in V...
. PCA begins by computing the covariance matrix of the



It then projects the data onto the first k eigenvectors of that matrix. By comparison, KPCA begins by computing the covariance matrix of the data after being transformed into a higher-dimensional space,

It then projects the transformed data onto the first k eigenvectors of that matrix, just like PCA. It uses the kernel trick to factor away much of the computation, such that the entire process can be performed without actually computing


Swiss roll
A Swiss roll or jelly roll is a type of sponge cake roll. The thin cake is made of eggs, flour and sugar and baked in a very shallow rectangular baking tray, called a sheet pan. The cake is removed from the pan and spread with jam or buttercream, rolled up, and served in circular slices.The...
manifold.
KPCA has an internal model, so it can be used to map points onto its embedding that were not available at training time.
Isomap
IsomapIsomap
In statistics, Isomap is one of several widely used low-dimensional embedding methods, where geodesic distances on a weighted graph are incorporated with the classical scaling . Isomap is used for computing a quasi-isometric, low-dimensional embedding of a set of high-dimensional data points...
is a combination of the Floyd-Warshall algorithm
Floyd-Warshall algorithm
In computer science, the Floyd–Warshall algorithm is a graph analysis algorithm for finding shortest paths in a weighted graph and also for finding transitive closure of a relation R...
with classic Multidimensional Scaling
Multidimensional scaling
Multidimensional scaling is a set of related statistical techniques often used in information visualization for exploring similarities or dissimilarities in data. MDS is a special case of ordination. An MDS algorithm starts with a matrix of item–item similarities, then assigns a location to each...
. Classic Multidimensional Scaling (MDS) takes a matrix of pair-wise distances between all points, and computes a position for each point. With NLDR algorithms like Isomap, however, the pair-wise distances are only known between neighboring points. So Isomap uses the Floyd-Warshall algorithm to compute the pair-wise distances between all of the other points. This effectively estimates the full matrix of pair-wise geodesic distances between all of the points. Isomap then uses classic MDS to compute the reduced-dimensional positions of all the points.
Landmark-Isomap is a variant of this algorithm that uses landmarks to increase speed, at the cost of some accuracy.
Locally-Linear Embedding
Locally-Linear Embedding (LLE) was presented at approximately the same time as Isomap. It has several advantages over Isomap, including faster optimization when implemented to take advantage of sparse matrix algorithms, and better results with many problems. LLE also begins by finding a set of the nearest neighbors of each point. It then computes a set of weights for each point that best describe the point as a linear combination of its neighbors. Finally, it uses an eigenvector-based optimization technique to find the low-dimensional embedding of points, such that each point is still described with the same linear combination of its neighbors. LLE tends to handle non-uniform sample densities poorly because there is no fixed unit to prevent the weights from drifting as various regions differ in sample densities. LLE has no internal model.LLE computes the barycentric coordinates of a point Xi based on its neighbors Xj. The original point is reconstructed by a linear combination, given by the weight matrix Wij, of its neighbors. The reconstruction error is given by the cost function E(W).

The weights Wij refer to the amount of contribution the point Xj has while reconstructing the point Xi. The cost function is minimized under 2 constraints:
(a) Each data point Xi is reconstructed only from its neighbors, thus enforcing Wij to be zero if point Xj is not a neighbor of the point Xi and
(b) The sum of every row of the weight matrix equals 1.

The original data points are collected in a D dimensional space and the goal of the algorithm is to reduce the dimensionality to d such that D >> d. The same weights Wij that reconstructs the i th data point in the D dimensional space will be used to reconstruct the same point in the lower d dimensional space. A neighborhood preserving map is created based on this idea. Each point Xi in the D dimensional space is mapped onto a point Yi in the d dimensional space by minimizing the cost function

In this cost function unlike the previous one the weights Wij are kept fixed and the minimization is done on the points Yi to optimize the coordinates. This minimization problem can be solved by solving a sparse N X N eigen value problem, whose bottom d nonzero eigen vectors provide an orthogonal set of coordinates. Generally the data points are reconstructed from K nearest neighbors, as measured by Euclidean distance
Euclidean distance
In mathematics, the Euclidean distance or Euclidean metric is the "ordinary" distance between two points that one would measure with a ruler, and is given by the Pythagorean formula. By using this formula as distance, Euclidean space becomes a metric space...
. For such an implementation the algorithm has only one free parameter K, which can be chosen by cross validation.
Laplacian Eigenmaps
Laplacian Eigenmaps uses spectral techniques to perform dimensionality reduction. This technique relies on the basic assumption that the data lies in a low dimensional manifold in a high dimensional space. This algorithm cannot embed out of sample points, but techniques based on Reproducing kernel Hilbert spaceReproducing kernel Hilbert space
In functional analysis , a reproducing kernel Hilbert space is a Hilbert space of functions in which pointwise evaluation is a continuous linear functional. Equivalently, they are spaces that can be defined by reproducing kernels...
regularization exist for adding this capability. Such techniques can be applied to other nonlinear dimensionality reduction algorithms as well.
Traditional techniques like Principal Component Analysis do not consider the intrinsic geometry of the data. Laplacian Eigenmaps builds a graph from neighborhood information of the data set. Each data point serves as a node on the graph and connectivity between nodes is governed by the proximity of neighboring points (using e.g. the k-nearest neighbor algorithm
K-nearest neighbor algorithm
In pattern recognition, the k-nearest neighbor algorithm is a method for classifying objects based on closest training examples in the feature space. k-NN is a type of instance-based learning, or lazy learning where the function is only approximated locally and all computation is deferred until...
). The graph thus generated can be considered as a discrete approximation of the low dimensional manifold in the high dimensional space. Minimization of a cost function based on the graph ensures that points close to each other on the manifold are mapped close to each other in the low dimensional space, preserving local distances. The eigenfunctions of the Laplace-Beltrami operator
Laplace-Beltrami operator
In differential geometry, the Laplace operator, named after Pierre-Simon Laplace, can be generalized to operate on functions defined on surfaces in Euclidean space and, more generally, on Riemannian and pseudo-Riemannian manifolds. This more general operator goes by the name Laplace–Beltrami...
on the manifold serve as the embedding dimensions, since under mild conditions this operator has a countable spectrum that is a basis for square integrable functions on the manifold (compare to Fourier series
Fourier series
In mathematics, a Fourier series decomposes periodic functions or periodic signals into the sum of a set of simple oscillating functions, namely sines and cosines...
on the unit circle manifold). Attempts to place Laplacian eigenmaps on solid theoretical ground have met with some success, as under certain nonrestrictive assumptions, the graph Laplacian matrix has been shown to converge to the Laplace-Beltrami operator as the number of points goes to infinity. Matlab code for Laplacian Eigenmaps can be found in algorithms and the PhD thesis of Belkin can be found at the Ohio State University
Ohio State University
The Ohio State University, commonly referred to as Ohio State, is a public research university located in Columbus, Ohio. It was originally founded in 1870 as a land-grant university and is currently the third largest university campus in the United States...
.
Diffusion Maps
Diffusion maps leverages the relationship between heat diffusion and a random walk (Markov ChainMarkov chain
A Markov chain, named after Andrey Markov, is a mathematical system that undergoes transitions from one state to another, between a finite or countable number of possible states. It is a random process characterized as memoryless: the next state depends only on the current state and not on the...
); an analogy is drawn between the diffusion operator on a manifold and a Markov transition matrix operating on functions defined on the graph whose nodes were sampled from the manifold.. In particular let a data set be represented by








Thus one can think of the individual data points as the nodes of a graph and the kernel k defining some sort of affinity on that graph. The graph is symmetric by construction since the kernel is symmetric. It is easy to see here that from the tuple {X,k} one can construct a reversible Markov Chain
Markov chain
A Markov chain, named after Andrey Markov, is a mathematical system that undergoes transitions from one state to another, between a finite or countable number of possible states. It is a random process characterized as memoryless: the next state depends only on the current state and not on the...
. This technique is fairly popular in a variety of fields and is known as the graph laplacian.
The graph K = (X,E) can be constructed for example using a Gaussian kernel.

In this above equation



Geodesic
In mathematics, a geodesic is a generalization of the notion of a "straight line" to "curved spaces". In the presence of a Riemannian metric, geodesics are defined to be the shortest path between points in the space...
distance should be used to actually measure distances on the manifold
Manifold
In mathematics , a manifold is a topological space that on a small enough scale resembles the Euclidean space of a specific dimension, called the dimension of the manifold....
. Since the exact structure of the manifold is not available, the geodesic distance is approximated by euclidean distances with only nearest neighbors. The choice





















For a given value of t





Euclidean distance
In mathematics, the Euclidean distance or Euclidean metric is the "ordinary" distance between two points that one would measure with a ruler, and is given by the Pythagorean formula. By using this formula as distance, Euclidean space becomes a metric space...
or even geodesic distance.
Hessian LLE
Like LLE, Hessian LLE is also based on sparse matrix techniques. It tends to yield results of a much higher quality than LLE. Unfortunately, it has a very costly computational complexity, so it is not well-suited for heavily-sampled manifolds. It has no internal model.Modified LLE
Modified LLE (MLLE) is another LLE variant which uses multiple weights in each neighborhood to address the local weight matrix conditioning problem which leads to distortions in LLE maps. MLLE produces robust projections similar to Hessian LLE, but without the significant additional computational cost.Local tangent space alignment
LTSALocal tangent space alignment
Local tangent space alignment is a method for manifold learning, which can efficiently learn a nonlinear embedding into low-dimensional coordinates from high-dimensional data, and can also reconstruct high-dimensional coordinates from embedding coordinates...
is based on the intuition that when a manifold is correctly unfolded, all of the tangent hyperplanes to the manifold will become aligned. It begins by computing the k-nearest neighbors of every point. It computes the tangent space at every point by computing the d-first principal components in each local neighborhood. It then optimizes to find an embedding that aligns the tangent spaces.
Local Multidimensional Scaling
Local Multidimensional Scaling performs multidimensional scaling in local regions, and then uses convex optimization to fit all the pieces together.Maximum Variance Unfolding
Maximum Variance Unfolding was formerly known as Semidefinite Embedding. The intuition for this algorithm is that when a manifold is properly unfolded, the variance over the points is maximized. This algorithm also begins by finding the k-nearest neighbors of every point. It then seeks to solve the problem of maximizing the distance between all non-neighboring points, constrained such that the distances between neighboring points are preserved. The primary contribution of this algorithm is a technique for casting this problem as a semidefinite programming problem. Unfortunately, semidefinite programming solvers have a high computational cost. The Landmark-MVU variant of this algorithm uses landmarks to increase speed with some cost to accuracy. It has no model.Nonlinear PCA
Nonlinear PCA (NLPCA) uses backpropagationBackpropagation
Backpropagation is a common method of teaching artificial neural networks how to perform a given task. Arthur E. Bryson and Yu-Chi Ho described it as a multi-stage dynamic system optimization method in 1969 . It wasn't until 1974 and later, when applied in the context of neural networks and...
to train a multi-layer perceptron to fit to a manifold. Unlike typical MLP training, which only updates the weights, NLPCA updates both the weights and the inputs. That is, both the weights and inputs are treated as latent values. After training, the latent inputs are a low-dimensional representation of the observed vectors, and the MLP maps from that low-dimensional representation to the high-dimensional observation space.
Data-Driven High Dimensional Scaling
Data-Driven High Dimensional Scaling (DD-HDS) is closely related to Sammon's mapping and curvilinear component analysis except that (1) it simultaneously penalizes false neighborhoods and tears by focusing on small distances in both original and output space, and that (2) it accounts for concentration of measureConcentration of measure
In mathematics, concentration of measure is a principle that is applied in measure theory, probability and combinatorics, and has consequences for other fields such as Banach space theory. Informally, it states that "A random variable that depends in a Lipschitz way on many independent variables ...
phenomenon by adapting the weighting function to the distance distribution.
Data-Driven High Dimensional Scaling (DD-HDS) is closely related to Sammon's mapping and curvilinear component analysis except that (1) it simultaneously penalizes false neighborhoods and tears by focusing on small distances in both original and output space, and that (2) it accounts for concentration of measure
Concentration of measure
In mathematics, concentration of measure is a principle that is applied in measure theory, probability and combinatorics, and has consequences for other fields such as Banach space theory. Informally, it states that "A random variable that depends in a Lipschitz way on many independent variables ...
phenomenon by adapting the weighting function to the distance distribution.
Manifold Sculpting
Manifold Sculpting uses graduated optimizationGraduated optimization
Graduated optimization is a global optimization technique that attempts to solve a difficult optimization problem by initially solving a greatly simplified problem, and progressively transforming that problem until it is equivalent to the difficult optimization problem.-Technique...
to find an embedding. Like other algorithms, it computes the k-nearest neighbors and tries to seek an embedding that preserves relationships in local neighborhoods. It slowly scales variance out of higher dimensions, while simultaneously adjusting points in lower dimensions to preserve those relationships. If the rate of scaling is small, it can find very precise embeddings. It boasts higher empirical accuracy than other algorithms with several problems. It can also be used to refine the results from other manifold learning algorithms. It struggles to unfold some manifolds, however, unless a very slow scaling rate is used. It has no model.
RankVisu
RankVisu is designed to preserve rank of neighborhood rather than distance. RankVisu is especially useful on difficult tasks (when the preservation of distance cannot be achieved satisfyingly). Indeed, the rank of neighborhood is less informative than distance (ranks can be deduced from distances but distances cannot be deduced from ranks) and its preservation is thus easier.Topologically Constrained Isometric Embedding
Topologically Constrained Isometric Embedding (TCIE) is an algorithm based approximating geodesic distances after filtering geodesics inconsistent with the Euclidean metric. Aimed at correcting the distortions caused when Isomap is used to map intrinsically non-convex data, TCIE uses weight least-squares MDS in order to obtain a more accurate mapping. The TCIE algorithm first detects possible boundary points in the data, and during computation of the geodesic length marks inconsistent geodesics, to be given a small weight in the weighted Stress majorizationStress majorization
Stress majorization is an optimization strategy used in multidimensional scaling where, for a set of n, m-dimensional data items, a configuration X of n points in rStress majorization is an optimization strategy used in multidimensional scaling (MDS) where, for a set of n, m-dimensional data...
that follows.
Relational Perspective Map
Relational perspective map is a special class of multidimensional scalingMultidimensional scaling
Multidimensional scaling is a set of related statistical techniques often used in information visualization for exploring similarities or dissimilarities in data. MDS is a special case of ordination. An MDS algorithm starts with a matrix of item–item similarities, then assigns a location to each...
algorithm. The algorithm finds a configuration of data points on a manifold by simulating a multi-particle dynamic system on a closed manifold, where data points are mapped to particles and distances (or dissimilarity) between data points are used as strength of a kind of repulsive force. As the manifold gradually grows in size the multi-particale system cools down gradually and converges to configuration that reflects the distance information of the data points.
Relational perspective map is initially inspired by the physic system in which positively charged particles freely move on the surface of a ball. Guided by Coulumbian repulsive force between particles, the minimal energy configuration of the particles will reflect the strength of repulsive forces between the particles.
Relational perspective map is introduced in.
The algorithm firstly used the flat torus
Torus
In geometry, a torus is a surface of revolution generated by revolving a circle in three dimensional space about an axis coplanar with the circle...
as the image manifold, then it has been extended (in the software VisuMap to use other types of closed manifolds, like sphere
Sphere
A sphere is a perfectly round geometrical object in three-dimensional space, such as the shape of a round ball. Like a circle in two dimensions, a perfect sphere is completely symmetrical around its center, with all points on the surface lying the same distance r from the center point...
, projective space
Projective space
In mathematics a projective space is a set of elements similar to the set P of lines through the origin of a vector space V. The cases when V=R2 or V=R3 are the projective line and the projective plane, respectively....
and klein-bottle, as image manifolds.
Methods based on proximity matrices
A method based on proximity matrices is one where the data is presented to the algorithm in the form of a similarity matrixSimilarity matrix
A similarity matrix is a matrix of scores which express the similarity between two data points. Similarity matrices are strongly related to their counterparts, distance matrices and substitution matrices.-Use in sequence alignment:...
or a distance matrix
Distance matrix
In mathematics, computer science and graph theory, a distance matrix is a matrix containing the distances, taken pairwise, of a set of points...
. These methods all fall under the broader class of metric multidimensional scaling. The variations tend to be differences in how the proximity data is computed; for example, Isomap
Isomap
In statistics, Isomap is one of several widely used low-dimensional embedding methods, where geodesic distances on a weighted graph are incorporated with the classical scaling . Isomap is used for computing a quasi-isometric, low-dimensional embedding of a set of high-dimensional data points...
, locally linear embeddings, maximum variance unfolding, and Sammon mapping
Sammon's projection
Sammon projection or Sammon mapping is an algorithm that maps a high-dimensional space to a space of lower dimensionality by trying to preserve the structure of inter-point distances in high-dimensional space in the lower-dimension projection. It is particularly suited for use in exploratory data...
(which is not in fact a mapping) are examples of metric multidimensional scaling methods.
See also
- Discriminant analysis
- Elastic mapElastic mapElastic maps provide a tool for nonlinear dimensionality reduction. By their construction, they are system of elastic springs embedded in the dataspace. This system approximates a low-dimensional manifold...
- Pairwise distance methods
- Self-organizing mapSelf-organizing mapA self-organizing map or self-organizing feature map is a type of artificial neural network that is trained using unsupervised learning to produce a low-dimensional , discretized representation of the input space of the training samples, called a map...
(SOM) - Topographic mapTopographic mapA topographic map is a type of map characterized by large-scale detail and quantitative representation of relief, usually using contour lines in modern mapping, but historically using a variety of methods. Traditional definitions require a topographic map to show both natural and man-made features...
External links
- Isomap
- Generative Topographic Mapping
- Mike Tipping's Thesis
- Gaussian Process Latent Variable Model
- Locally Linear Embedding
- Relational Perspective Map
- Waffles is an open source C++ library containing implementations of LLE, Manifold Sculpting, and some other manifold learning algorithms.
- DD-HDS homepage
- RankVisu homepage
- Short review of Diffusion Maps