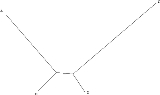
Maximum parsimony
Encyclopedia
Parsimony is a non-parametric statistical
method commonly used in computational phylogenetics
for estimating phylogenies. Under parsimony, the preferred phylogenetic tree
is the tree that requires the least evolutionary change to explain some observed data.
of discrete phylogenetic characters to infer one or more optimal phylogenetic tree
s for a set of taxa, commonly a set of species
or reproductively-isolated population
s of a single species. These methods operate by evaluating candidate phylogenetic trees according to an explicit optimality criterion
; the tree with the most favorable score is taken as the best estimate of the phylogenetic relationships of the included taxa. Maximum parsimony is used with most kinds of phylogenetic data; until recently, it was the only widely-used character-based tree estimation method used for morphological data.
Estimating phylogenies is not a trivial problem. A huge number of possible phylogenetic trees exist for any reasonably sized set of taxa; for example, a mere ten species gives over two million possible unrooted trees. These possibilities must be searched to find a tree that best fits the data according to the optimality criterion. However, the data themselves do not lead to a simple, arithmetic solution to the problem. Ideally, we would expect the distribution of whatever evolutionary characters (such as phenotypic traits
or allele
s) to directly follow the branching pattern of evolution. Thus we could say that if two organisms possess a shared character, they should be more closely related to each other than to a third organism that lacks this character (provided that character was not present in the last common ancestor of all three, in which case it would be a symplesiomorphy
). We would predict that bats and monkeys are more closely related to each other than either is to a fish, because they both possess hair—a synapomorphy
. However, we cannot say that bats and monkeys are more closely related to one another than they are to whales because they share hair, because we believe the last common ancestor of the three had hair.
However, the phenomena of convergent evolution
, parallel evolution
, and evolutionary reversals (collectively termed homoplasy) add an unpleasant wrinkle to the problem of estimating phylogeny. For a number of reasons, two organisms can possess a trait not present in their last common ancestor: If we naively took the presence of this trait as evidence of a relationship, we would reconstruct an incorrect tree. Real phylogenetic data include substantial homoplasy, with different parts of the data suggesting sometimes very different relationships. Methods used to estimate phylogenetic trees are explicitly intended to resolve the conflict within the data by picking the phylogenetic tree that is the best fit to all the data overall, accepting that some data simply will not fit. It is often mistakenly believed that parsimony assumes that convergence is rare; in fact, even convergently-derived characters have some value in maximum-parsimony-based phylogenetic analyses, and the prevalence of convergence does not systematically affect the outcome of parsimony-based methods.
Data that do not fit a tree perfectly are not simply "noise", they can contain relevant phylogenetic signal in some parts of a tree, even if they conflict with the tree overall. In the whale example given above, the lack of hair in whales is homoplastic: It reflects a return to the condition present in ancient ancestors of mammals, who lacked hair. This similarity between whales and ancient mammal ancestors is in conflict with the tree we accept, since it implies that the mammals with hair should form a group excluding whales. However, among the whales, the reversal to hairlessness actually correctly associates the various types of whales (including dolphins and porpoises) into the group Cetacea
. Still, the determination of the best-fitting tree—and thus which data do not fit the tree—is a complex process. Maximum parsimony is one method developed to do this.
. Whether it must be directly heritable, or whether indirect inheritance (e.g., learned behaviors) is acceptable, is not entirely resolved.
Each character is divided into discrete character states, into which the variations observed are classified. Character states are often formulated as descriptors, describing the condition of the character substrate. For example, the character "eye color" might have the states "blue" and "brown." Characters can have two or more states (they can have only one, but these characters lend nothing to a maximum parsimony analysis, and are often excluded).
Coding characters for phylogenetic analysis is not an exact science, and there are numerous complicating issues. Typically, taxa are scored with the same state if they are more similar to one another in that particular attribute than each is to taxa scored with a different state. This is not straightforward when character states are not clearly delineated or when they fail to capture all of the possible variation in a character. How would one score the previously mentioned character for a taxon (or individual) with hazel eyes? Or green? As noted above, character coding is generally based on similarity: Hazel and green eyes might be lumped with blue because they are more similar to that color (being light), and the character could be then recoded as "eye color: light; dark." Alternatively, there can be multi-state characters, such as "eye color: brown; hazel, blue; green."
Ambiguities in character state delineation and scoring can be a major source of confusion, dispute, and error in phylogenetic analysis using character data. Note that, in the above example, "eyes: present; absent" is also a possible character, which creates issues because "eye color" is not applicable if eyes are not present. For such situations, a "?" ("unknown") is scored, although sometimes "X" or "-" (the latter usually in sequence
data) are used to distinguish cases where a character cannot be scored from a case where the state is simply unknown. Current implementations of maximum parsimony generally treat unknown values in the same manner: the reasons the data are unknown have no particular effect on analysis. Effectively, the program treats a ? as if it held the state that would involve the fewest extra steps in the tree (see below), although this is not an explicit step in the algorithm.
Genetic data are particularly amenable to character-based phylogenetic methods such as maximum parsimony because protein and nucleotide sequences are naturally discrete: A particular position in a nucleotide sequence can be either adenine
, cytosine
, guanine
, or thymine
/ uracil
, or a sequence gap; a position (residue
) in a protein sequence will be one of the basic amino acids or a sequence gap. Thus, character scoring is rarely ambiguous, except in cases where sequencing
methods fail to produce a definitive assignment for a particular sequence position. Sequence gaps are sometimes treated as characters, although there is no consensus on how they should be coded.
Characters can be treated as unordered or ordered. For a binary (two-state) character, this makes little difference. For a multi-state character, unordered characters can be thought of as having an equal "cost" (in terms of number of "evolutionary events") to change from any one state to any other; complementarily, they do not require passing through intermediate states. Ordered characters have a particular sequence in which the states must occur through evolution, such that going between some states requires passing through an intermediate. This can be thought of complementarily as having different costs to pass between different pairs of states. In the eye-color example above, it is possible to leave it unordered, which imposes the same evolutionary "cost" to go from brown-blue, green-blue, green-hazel, etc. Alternatively, it could be ordered brown-hazel-green-blue; this would normally imply that it would cost two evolutionary events to go from brown-green, three from brown-blue, but only one from brown-hazel. This can also be thought of as requiring eyes to evolve through a "hazel stage" to get from brown to green, and a "green stage" to get from hazel to blue, etc.
There is a lively debate on the utility and appropriateness of character ordering, but no consensus. Some authorities order characters when there is a clear logical, ontogenetic, or evolutionary transition among the states (for example, "legs: short; medium; long"). Some accept only some of these criteria. Some run an unordered analysis, and order characters that show a clear order of transition in the resulting tree (which practice might be accused of circular reasoning
). Some authorities refuse to order characters at all, suggesting that it biases an analysis to require evolutionary transitions to follow a particular path.
It is also possible to apply differential weighting to individual characters. This is usually done relative to a "cost" of 1. Thus, some characters might be seen as more likely to reflect the true evolutionary relationships among taxa, and thus they might be weighted at a value 2 or more; changes in these characters would then count as two evolutionary "steps" rather than one when calculating tree scores (see below). There has been much discussion in the past about character weighting. Most authorities now weight all characters equally, although exceptions are common. For example, allele frequency
data is sometimes pooled in bins and scored as an ordered character. In these cases, the character itself is often downweighted so that small changes in allele frequencies count less than major changes in other characters. Also, the third codon position in a coding nucleotide sequence is particularly labile, and is sometimes downweighted, or given a weight of 0, on the assumption that it is more likely to exhibit homoplasy. In some cases, repeated analyses are run, with characters reweighted in inverse proportion to the degree of homoplasy discovered in the previous analysis (termed successive weighting); this is another technique that might be considered circular reasoning
.
Character state changes can also be weighted individually. This is often done for nucleotide sequence data; it has been empirically determined that certain base changes (A-C, A-T, G-C, G-T, and the reverse changes) occur much less often than others. These changes are therefore often weighted more. As shown above in the discussion of character ordering, ordered characters can be thought of as a form of character state weighting.
Some systematists prefer to exclude characters known to be, or suspected to be, highly homoplastic or that have a large number of unknown entries ("?"). As noted below, theoretical and simulation work has demonstrated that this is likely to sacrifice accuracy rather than improve it. This is also the case with characters that are variable in the terminal taxa: theoretical, congruence, and simulation studies have all demonstrated that such polymorphic characters contain significant phylogenetic information.
Empirical, theoretical, and simulation studies have led to a number of dramatic demonstrations of the importance of adequate taxon sampling. Most of these can be summarized by a simple observation: a phylogenetic data matrix has dimensions of characters times taxa. Doubling the number of taxa doubles the amount of information in a matrix just as surely as doubling the number of characters. Each taxon represents a new sample for every character, but, more importantly, it (usually) represents a new combination of character states. These character states can not only determine where that taxon is placed on the tree, they can inform the entire analysis, possibly causing different relationships among the remaining taxa to be favored by changing estimates of the pattern of character changes.
The most disturbing weakness of parsimony analysis, that of long-branch attraction (see below) is particularly pronounced with poor taxon sampling, especially in the four-taxon case. This is a well-understood case in which additional character sampling may not improve the quality of the estimate. As taxa are added, they often break up long branches (especially in the case of fossils), effectively improving the estimation of character state changes along them. Because of the richness of information added by taxon sampling, it is even possible to produce highly accurate estimates of phylogenies with hundreds of taxa using only a few thousand characters.
Although many studies have been performed, there is still much work to be done on taxon sampling strategies. Because of advances in computer performance, and the reduced cost and increased automation of molecular sequencing, sample sizes overall are on the rise, and studies addressing the relationships of hundreds of taxa (or other terminal entities, such as genes) are becoming common. Of course, this is not to say that adding characters is not also useful; the number of characters is increasing as well.
Some systematists prefer to exclude taxa based on the number of unknown character entries ("?") they exhibit, or because they tend to "jump around" the tree in analyses (i.e., they are "wildcards"). As noted below, theoretical and simulation work has demonstrated that this is likely to sacrifice accuracy rather than improve it. Although these taxa may generate more most-parsimonious trees (see below), methods such as agreement subtrees and reduced consensus can still extract information on the relationships of interest.
It has been observed that inclusion of more taxa tends to lower overall support values (bootstrap
percentages or decay indices, see below). The cause of this is clear: as additional taxa are added to a tree, they subdivide the branches to which they attach, and thus dilute the information that supports that branch. While support for individual branches is reduced, support for the overall relationships is actually increased. Consider analysis that produces the following tree: (fish ,(lizard ,(whale, (cat, monkey)))). Adding a rat and a walrus will probably reduce the support for the (whale, (cat, monkey)) clade, because the rat and the walrus may fall within this clade, or outside of the clade, and since these five animals are all relatively closely related, there should be more uncertainty about their relationships. Within error, it may be impossible to determine any of these animals' relationships relative to one another. However, the rat and the walrus will probably add character data that cements the grouping any two of these mammals exclusive of the fish or the lizard; where the initial analysis might have been misled, say, by the presence of fins in the fish and the whale, the presence of the walrus, with blubber and fins like a whale but whiskers like a cat and a rat, firmly ties the whale to the mammals.
To cope with this problem, agreement subtrees, reduced consensus, and double-decay analysis seek to identify supported relationships (in the form of "n-taxon statements," such as the four-taxon statement "(fish, (lizard, (cat, whale)))") rather than whole trees. If the goal of an analysis is a resolved tree, as is the case for comparative phylogenetics, these methods cannot solve the problem. However, if the tree estimate is so poorly supported, the results of any analysis derived from the tree will probably be too suspect to use anyway.
Trees are scored (evaluated) by using a simple algorithm to determine how many "steps" (evolutionary transitions) are required to explain the distribution of each character. A step is, in essence, a change from one character state to another, although with ordered characters some transitions require more than one step. Contrary to popular belief, the algorithm does not explicitly assign particular character states to nodes (branch junctions) on a tree: the least number of steps can involve multiple, equally costly assignments and distributions of evolutionary transitions. What is optimized is the total number of changes.
There are many more possible phylogenetic tree
s than can be searched exhaustively for more than eight taxa or so. A number of algorithms are therefore used to searching among the possible trees. Many of these involve taking an initial tree (usually the favored tree from the last iteration of the algorithm), and perturbing it to see if the change produces a higher score.
The trees resulting from parsimony search are unrooted: They show all the possible relationships of the included taxa, but they lack any statement on relative times of divergence. A particular branch is chosen to root the tree by the user. This branch is then taken to be outside all the other branches of the tree, which together form a monophyletic group. This imparts a sense of relative time to the tree. Incorrect choice of a root can result in incorrect relationships on the tree, even if the tree is itself correct in its unrooted form.
Parsimony analysis often returns a number of equally most-parsimonious trees (MPTs). A large number of MPTs is often seen as an analytical failure, and is widely believed to be related to the number of missing entries ("?") in the dataset, characters showing too much homoplasy, or the presence of topologically labile "wildcard" taxa (which may have many missing entries). Numerous methods have been proposed to reduce the number of MPTs, including removing characters or taxa with large amounts of missing data before analysis, removing or downweighting highly homoplastic characters (successive weighting) or removing wildcard taxa (the phylogenetic trunk method) a posteriori
and then reanalyzing the data.
Numerous theoretical and simulation studies have demonstrated that highly homoplastic characters, characters and taxa with abundant missing data, and "wildcard" taxa contribute to the analysis. Although excluding characters or taxa may appear to improve resolution, the resulting tree is based on less data, and is therefore a less reliable estimate of the phylogeny (unless the characters or taxa are non informative, see safe taxonomic reduction). Today's general consensus is that having multiple MPTs is a valid analytical result; it simply indicates that there is insufficient data to resolve the tree completely. In many cases, there is substantial common structure in the MPTs, and differences are slight and involve uncertainty in the placement of a few taxa. There are a number of methods for summarizing the relationships within this set, including consensus trees, which show common relationships among all the taxa, and pruned agreement subtrees, which show common structure by temporarily pruning "wildcard" taxa from every tree until they all agree. Reduced consensus takes this one step further, but showing all subtrees (and therefore all relationships) supported by the input trees.
Even if multiple MPTs are returned, parsimony analysis still basically produces a point-estimate, lacking confidence interval
s of any sort. This has often been levelled as a criticism, since there is certainly error in estimating the most-parsimonious tree, and the method does not inherently include any means of establishing how sensitive its conclusions are to this error. Several methods have been used to assess support.
Jackknifing and bootstrapping
, well-known statistical resampling
procedures, have been employed with parsimony analysis. The jackknife, which involves resampling without replacement ("leave-one-out") can be employed on characters or taxa; interpretation may become complicated in the latter case, because the variable of interest is the tree, and comparison of trees with different taxa is not straightforward. The bootstrap, resampling with replacement (sample x items randomly out of a sample of size x, but items can be picked multiple times), is only used on characters, because adding duplicate taxa does not change the result of a parsimony analysis. The bootstrap is much more commonly employed in phylogenetics (as elsewhere); both methods involve an arbitrary but large number of repeated iterations involving perturbation of the original data followed by analysis. The resulting MPTs from each analysis are pooled, and the results are usually presented on a 50% Majority Rule Consensus tree, with individual branches (or nodes) labelled with the percentage of bootstrap MPTs in which they appear. This "bootstrap percentage" (which is not a P-value
, as is sometimes claimed) is used as a measure of support. Technically, it is supposed to be a measure of repeatability, the probability that that branch (node, clade) would be recovered if the taxa were sampled again. Experimental tests with viral phylogenies suggest that the bootstrap percentage is not a good estimator of repeatability for phylogenetics, but it is a reasonable estimator of accuracy. In fact, it has been shown that the bootstrap percentage, as an estimator of accuracy, is biased, and that this bias results on average in an underestimate of confidence (such that as little as 70% support might really indicate up to 95% confidence). However, the direction of bias cannot be ascertained in individual cases, so assuming that high values bootstrap support indicate even higher confidence is unwarranted.
Another means of assessing support is Bremer support, or the decay index (which is technically not an index). This is simply the difference in number of steps between the score of the MPT(s), and the score of the most parsimonious tree that does NOT contain a particular clade (node, branch). It can be thought of as the number of steps you have to add to lose that clade; implicitly, it is meant to suggest how great the error in the estimate of the score of the MPT must be for the clade to no longer be supported by the analysis, although this is not necessarily what it does. Decay index values are often fairly low (one or two steps being typical), but they often appear to be proportional to bootstrap percentages. However, interpretation of decay values is not straightforward, and they seem to be preferred by authors with philosophical objections to the bootstrap (although many morphological systematists, especially paleontologists, report both). Double-decay analysis is a decay counterpart to reduced consensus that evaluates the decay index for all possible subtree relationships (n-taxon statements) within a tree.
Maximum parsimony is a very simple approach, and is popular for this reason. However, it is not statistically consistent. That is, it is not guaranteed to produce the true tree with high probability, given sufficient data. Consistency, here meaning the monotonic convergence on the correct answer with the addition of more data, is a desirable property of any statistical method
. As demonstrated in 1978 by Joe Felsenstein
, maximum parsimony can be inconsistent under certain conditions. The category of situations in which this is known to occur is called long branch attraction
, and occurs, for example, where there are long branches (a high level of substitutions) for two characters (A & C), but short branches for another two (B & D). A and B diverged from a common ancestor, as did C and D.
Assume for simplicity that we are considering a single binary character (it can either be + or -). Because the distance from B to D is small, in the vast majority of all cases, B and D will be the same. Here, we will assume that they are both + (+ and - are assigned arbitrarily and swapping them is only a matter of definition). If this is the case, there are four remaining possibilities. A and C can both be +, in which case all taxa are the same and all the trees have the same length. A can be + and C can be -, in which case only one character is different, and we cannot learn anything, as all trees have the same length. Similarly, A can be - and C can be +. The only remaining possibility is that A and C are both -. In this case, however, we group A and C together, and B and D together. As a consequence, when we have a tree of this type, the more data we collect (i.e. the more characters we study), the more we tend towards the wrong tree.
A simple and effective method for determining whether or not long branch attraction is affecting tree topology is the SAW method, named for Siddal and Whiting. If long branch attraction is suspected in a pair of taxa (A and B), simply remove taxon A ("saw" off the branch) and re-run the analysis. Then remove A and replace B, running the analysis again. If either of the taxa appear at different branch points in the absence of the other, there is evidence of long branch attraction. Since long branches can't possibly attract one another when only one is in the analysis, consistent taxon placement between treatments would indicate long branch attraction is not a problem.
Several other methods of phylogeny estimation are available, including maximum likelihood
, Bayesian phylogeny inference
, neighbour joining, and quartet methods. Of these, the first two both use a likelihood function
, and, if used properly, are theoretically immune to long-branch attraction. These methods are both parametric
, meaning that they rely on an explicit model of character evolution. It has been shown that, for some suboptimal models, these methods can also be inconsistent.
Another complication with maximum parsimony is that finding the most parsimonious tree is an NP-Hard
problem. The only currently available, efficient way of obtaining a solution, given an arbitrarily large set of taxa, is by using heuristic methods which do not guarantee that the most parsimonious tree will be recovered. These methods employ hill-climbing algorithms to progressively approach the best tree. However, it has been shown that there can be "tree islands" of suboptimal solutions, and the analysis can become trapped in these local optima. Thus, complex, flexible heuristics are required to ensure that tree space has been adequately explored. Several heuristics are available, including nearest neighbor interchange (NNI), tree bisection / reconnection (TBR), and the phylogenetic ratchet. This problem is certainly not unique to MP; any method that uses an optimality criterion faces the same problem, and none offer easy solutions.
, is that maximum parsimony assumes that the only way two species can share the same nucleotide at the same position is if they are genetically related. This asserts that phylogenetic applications of parsimony assume that all similarity is homologous (other interpretations, such as the assertion that two organisms might NOT be related at all, are nonsensical). This is emphatically not the case: as with any form of character-based phylogeny estimation, parsimony is used to test the homologous nature of similarities by finding the phylogenetic tree which best accounts for all of the similarities.
For example, birds and bats have wings, while crocodiles and humans do not. If these were the only data available, maximum parsimony would tend to group crocodiles with humans, and birds with bats (as would any other method of phylogenetic inference). We believe that humans are actually more closely related to bats than to crocodiles or birds. Our belief is founded on additional data that were not considered in the one-character example (using wings). If even a tiny fraction of these additional data, including information on skeletal structure, soft-tissue
morphology, integument, behaviour, genetics, etc., were included in the analysis, the faint phylogenetic signal produced by the presence of wings in birds and bats would be overwhelmed by the preponderance of data supporting the (human, bat)(bird, crocodile) tree.
It is often stated that parsimony is not relevant to phylogenetic inference because "evolution is not parsimonious." In most cases, there is no explicit alternative proposed; if no alternative is available, any statistical method is preferable to none at all. Additionally, it is not clear what would be meant if the statement "evolution is parsimonious" were in fact true. This could be taken to mean that more character changes may have occurred historically than are predicted using the parsimony criterion. Because parsimony phylogeny estimation reconstructs the minimum number of changes necessary to explain a tree, this is quite possible. However, it has been shown through simulation studies, testing with known in vitro
viral phylogenies, and congruence with other methods, that the accuracy of parsimony is in most cases not compromised by this. Parsimony analysis uses the number of character changes on trees to choose the best tree, but it does not require that exactly that many changes, and no more, produced the tree. As long as the changes that have not been accounted for are randomly distributed over the tree (a reasonable null expectation), the result should not be biased. In practice, the technique is robust: maximum parsimony exhibits minimal bias as a result of choosing the tree with the fewest changes.
An analogy can be drawn with choosing among contractors based on their initial (nonbinding) estimate of the cost of a job. The actual finished cost is very likely to be higher than the estimate. Despite this, choosing the contractor who furnished the lowest estimate should theoretically result in the lowest final project cost. This is because, in the absence of other data, we would assume that all of the relevant contractors have the same risk of cost overruns. In practice, of course, unscrupulous business practices may bias this result; in phylogenetics, too, some particular phylogenetic problems (for example, long branch attraction
, described above) may potentially bias results. In both cases, however, there is no way to tell if the result is going to be biased, or the degree to which it will be biased, based on the estimate itself. With parsimony too, there is no way to tell that the data are positively misleading, without comparison to other evidence.
Along the same lines, parsimony is often characterized as implicitly adopting the philosophical position that evolutionary change is rare, or that homoplasy (convergence and reversal) is minimal in evolution. This is not entirely true: parsimony minimizes the number of convergences and reversals that are assumed by the preferred tree, but this may result in a relatively large number of such homoplastic events. It would be more appropriate to say that parsimony assumes only the minimum amount of change implied by the data. As above, this does not require that these were the only changes that occurred; it simply does not infer changes for which there is no evidence. The shorthand for describing this is that "parsimony minimizes assumed homoplasies, it does not assume that homoplasy is minimal."
Parsimony is also sometimes associated with the notion that "the simplest possible explanation is the best," a generalisation of Occam's Razor
. Parsimony does prefer the solution that requires the least number of unsubstantiated assumptions and unsupportable conclusions, the solution that goes the least theoretical distance beyond the data. This is a very common approach to science, especially when dealing with systems that are so complex as to defy simple models. Parsimony does not by any means necessarily produce a "simple" assumption. Indeed, as a general rule, most character datasets are so "noisy" that no truly "simple" solution is possible.
, in that it employs an explicit model of character evolution. Such methods are potentially much more powerful than non-parametric statistical methods
like parsimony, but only if the model used is a reasonable approximation of the processes that produced the data. Maximum likelihood has probably surpassed parsimony in popularity with nucleotide sequence data, and Bayesian phylogenetic inference
, which uses the likelihood function, is becoming almost as prevalent.
Likelihood
is the relative counterpart to absolute probability
. If we know the number of possible outcomes of a test (N), and we know the number of those outcomes that fit a particular criterion (n), we can say that the probability of that criterion being met by an execution of that test is n/N. Thus, the probability of heads in the toss of a fair coin
is 50% (1/2). What if we don't know the number of possible outcomes? Obviously, we cannot then calculate probabilities. However, if we observe that one outcome happens twice as often as the other over an arbitrarily large number of tests, we can say that that outcome is twice as likely. Likelihoods are proportional to the true probabilities: if an outcome is twice as likely, we can say that it is twice as probable, even though we cannot say how probable it is.
Practically, the probability of a tree cannot be calculated directly. The probability of the data given a tree can be calculated if you assume a specific set of probabilities of character change (a model). The critical part of likelihood analysis is that the probability of the data given the tree is the likelihood of the tree given the data. Thus, the tree that has the highest probability of producing the observed data is the most likely tree.
Maximum likelihood, as implemented in phylogenetics, uses a stochastic model that gives the probability of a particular character changing at any given point on a tree. This model can have a potentially large number of parameters, which can account for differences in the probabilities of particular states, the probabilities of particular changes, and differences in the probabilities of change among characters.
A likelihood tree has meaningful branch lengths (i.e. it is a phylogram); these lengths are usually interpreted as being proportional to the average probability of change for characters on that branch (thus, on a branch of length 1, we would expect an average of one change per character, which is a lot). The state of each character is plotted on the tree, and the probability of that distribution of character states is calculated using the model and the branch lengths (which can be altered to maximize the probability of the data). This is the probability of that character, given the tree. The probabilities of all of the characters is multiplied together; they are usually negative log-transformed and added (producing the same effect), because the numbers become very small very quickly. This sum is the probability of the data, given the tree, or the likelihood of the tree. The tree with the highest likelihood (lowest negative log-transformed likelihood) given the data is preferred.
In the above analogy regarding choosing a contractor, maximum likelihood would be analogous to gathering data on the final cost of broadly comparable jobs performed by each contractor over the past year, and selecting the contractor with the lowest average cost for those comparable jobs. This method would be highly dependent on how comparable the jobs are, but, if they are properly chosen, it will produce a better estimate of the actual cost of the job. Further, it would not be misled by bias in contractor estimates, because it is based on the final cost, not on the (potentially biased) estimates.
In practice, maximum likelihood tends to favor trees that are very similar to the most parsimonious tree(s) for the same dataset. It has been shown to outperform parsimony in certain situations where the latter is known to be biased, including long-branch attraction. Note, however, that the performance of likelihood is dependent on the quality of the model employed; an incorrect model can produce a biased result. Studies have shown that incorporating a parameter to account for differences in rate of evolution among characters is often critical to accurate estimation of phylogenies; failure to model this or other crucial parameters may produce incorrect or biased results. Model parameters are usually estimated from the data, and the number (and type) of parameters is often determined using the hierarchical likelihood ratio test. The consequences of mis-specified models are just beginning to be explored in detail.
Likelihood is generally regarded as a more desirable method than parsimony, in that it is statistically consistent
, and has a better statistical foundation, and because it allows complex modeling of evolutionary processes. A major drawback is that ML is still quite slow relative to parsimony methods, sometimes requiring days to run large datasets. Maximum likelihood phylogenetic inference was proposed in the mid-Twentieth Century, but it has only been a popular method for phylogenetic inference since the 1990s, when computational power caught up with tremendous demands of ML analysis. Newer algorithms and implementations are bringing analysis times for large datasets into acceptable ranges. Until these methods gain widespread acceptance, parsimony will probably be preferred for extremely large datasets, especially when bootstrapping is used to assess confidence in the results.
One area where parsimony still holds much sway is in the analysis of morphological data. Until recently, stochastic models of character change were not available for non-molecular data. New methods, proposed by Paul Lewis, make essentially the same assumptions that parsimony analysis does, but do so within a likelihood framework. These models are not, however, widely implemented, and, unless modified, they require the modification of existing datasets (to deal with ordered characters, and the tendency to not record autapomorphies in morphological datasets.
Maximum likelihood has been criticised as assuming neutral evolution implicitly in its adoption of a stochastic model of evolution. This is not necessarily the case: as with parsimony, assuming a stochastic model does not presume that all evolution is stochastic. In practice, likelihood is robust to deviations from stochasticity. It performs well even on coding sequences that include sites believed to be under selection.
A related objection (often brought up by parsimony-only advocates) is the idea that evolution is too complex or too poorly understood to be modeled. This objection probably rests on a misunderstanding of the term "model." While it is customary to think of models as representing the mechanics of a process, this is not necessarily literally the case. In fact, a model is often selected not so much for its faithful reproduction of the phenomenon as its ability to make predictions. In practice, it is best not to try and exactly fit a model to a process, because there is a trade-off between number of parameters in a model and its statistical power
. Stochasticity may be a reasonably good fit to evolutionary data at a broad level, even if it does not accurately mirror the process at finer scales.
By analogy, no one claims that the human foot varies only in length and width, but differing combinations of length and width values can be combined to fit a wide variety of feet. In some cases, a slightly wider overall foot may be better fitted by increasing overall size rather than instep width, while a foot with a narrower heel might be better fit by a wider instep and a smaller shoe. Adding several more measurements would probably improve shoe fit somewhat, but would be impractical from a business standpoint. With increasingly precise fitting, differences between feet would make selling matched pairs of shoes impossible, and differences through time would mean that a proper fit at purchase might not be a proper fit when worn.
Parsimony has recently been shown to be more likely to recover the true tree in the face of profound changes in evolutionary ("model") parameters (e.g., the rate of evolutionary change) within a tree . This is particularly troublesome, since it is generally agreed that such changes may be a significant feature of deep divergences. Likelihood has had substantial success recovering known in vitro viral phylogenies, simulated phylogenies, and phylogenies confirmed by other method. It seems likely therefore that this potential complication does not strongly bias results for more shallow divergences. Several research groups are currently exploring ways to incorporate profound shifts in evolutionary parameters into likelihood analysis.
, which relates the posterior probability
of a tree to the likelihood of data, and the prior probability
of the tree and model of evolution. However, unlike parsimony and likelihood methods, Bayesian analysis
does not produce a single tree or set of equally optimal trees. Bayesian analysis uses the likelihood of trees in a Markov chain Monte Carlo
(MCMC) simulation to sample trees in proportion to their likelihood, thereby producing a credible sample of trees.
One commonly cited drawback of Bayesian analysis is the need to explicitly set out a set of prior probabilities for the range of potential outcomes. The idea of incorporating prior probabilities into an analysis has been suggested as a potential source of bias. Bayesian methods involve other potential issues, such as the evaluation of "convergence," the point at which the MCMC process stops searching for the "space" of credible solutions and begins to build the credible sample.
can come from a number of different sources, including measured distance (for example from immunological studies
) or morphometric analysis, various pairwise distance formulae (such as euclidean distance
) applied to discrete morphological characters, or genetic distance
from sequence, restriction fragment
, or allozyme
data. For phylogenetic character data, raw distance values can be calculated by simply counting the number of pairwise differences in character states (Manhattan distance).
Several simple algorithms exist to construct a tree directly from pairwise distances, including UPGMA
and neighbor joining (NJ), but these will not necessarily produce the best tree for the data. UPGMA assumes an ultrametric tree (a tree where all the path-lengths from the root to the tips are equal). Neighbor-joining
is a form of star decomposition, and can very quickly produce reasonable trees. It is very often used on its own, and in fact quite frequently produces reasonable trees.
Phylogeny estimation using distance methods has produced a number of controversies. The relationship between individual characters and the tree is lost in the process of reducing characters to distances. Since these methods do not use character data directly, and information locked in the distribution of character states can be lost in the pairwise comparison
s. Also, some complex phylogenetic relationships may produce biased distances. Despite these potential problems, distance methods are extremely fast, and they often produce a reasonable estimate of phylogeny. They also have certain benefits over the methods that use characters directly. Notably, distance methods allow use of data that may not be easily converted to character data, such as DNA-DNA hybridization assays.
Non-parametric statistics
In statistics, the term non-parametric statistics has at least two different meanings:The first meaning of non-parametric covers techniques that do not rely on data belonging to any particular distribution. These include, among others:...
method commonly used in computational phylogenetics
Computational phylogenetics
Computational phylogenetics is the application of computational algorithms, methods and programs to phylogenetic analyses. The goal is to assemble a phylogenetic tree representing a hypothesis about the evolutionary ancestry of a set of genes, species, or other taxa...
for estimating phylogenies. Under parsimony, the preferred phylogenetic tree
Phylogenetic tree
A phylogenetic tree or evolutionary tree is a branching diagram or "tree" showing the inferred evolutionary relationships among various biological species or other entities based upon similarities and differences in their physical and/or genetic characteristics...
is the tree that requires the least evolutionary change to explain some observed data.
In detail
Parsimony is part of a class of character-based tree estimation methods which use a matrixMatrix (mathematics)
In mathematics, a matrix is a rectangular array of numbers, symbols, or expressions. The individual items in a matrix are called its elements or entries. An example of a matrix with six elements isMatrices of the same size can be added or subtracted element by element...
of discrete phylogenetic characters to infer one or more optimal phylogenetic tree
Phylogenetic tree
A phylogenetic tree or evolutionary tree is a branching diagram or "tree" showing the inferred evolutionary relationships among various biological species or other entities based upon similarities and differences in their physical and/or genetic characteristics...
s for a set of taxa, commonly a set of species
Species
In biology, a species is one of the basic units of biological classification and a taxonomic rank. A species is often defined as a group of organisms capable of interbreeding and producing fertile offspring. While in many cases this definition is adequate, more precise or differing measures are...
or reproductively-isolated population
Population
A population is all the organisms that both belong to the same group or species and live in the same geographical area. The area that is used to define a sexual population is such that inter-breeding is possible between any pair within the area and more probable than cross-breeding with individuals...
s of a single species. These methods operate by evaluating candidate phylogenetic trees according to an explicit optimality criterion
Optimality criterion
In statistics, an optimality criterion provides a measure of the fit of the data to a given hypothesis. The selection process is determined by the solution that optimizes the criteria used to evaluate the alternative hypotheses...
; the tree with the most favorable score is taken as the best estimate of the phylogenetic relationships of the included taxa. Maximum parsimony is used with most kinds of phylogenetic data; until recently, it was the only widely-used character-based tree estimation method used for morphological data.
Estimating phylogenies is not a trivial problem. A huge number of possible phylogenetic trees exist for any reasonably sized set of taxa; for example, a mere ten species gives over two million possible unrooted trees. These possibilities must be searched to find a tree that best fits the data according to the optimality criterion. However, the data themselves do not lead to a simple, arithmetic solution to the problem. Ideally, we would expect the distribution of whatever evolutionary characters (such as phenotypic traits
Phenotype
A phenotype is an organism's observable characteristics or traits: such as its morphology, development, biochemical or physiological properties, behavior, and products of behavior...
or allele
Allele
An allele is one of two or more forms of a gene or a genetic locus . "Allel" is an abbreviation of allelomorph. Sometimes, different alleles can result in different observable phenotypic traits, such as different pigmentation...
s) to directly follow the branching pattern of evolution. Thus we could say that if two organisms possess a shared character, they should be more closely related to each other than to a third organism that lacks this character (provided that character was not present in the last common ancestor of all three, in which case it would be a symplesiomorphy
Symplesiomorphy
In cladistics, a symplesiomorphy or symplesiomorphic character is a trait which is shared between two or more taxa, but which is also shared with other taxa which have an earlier last common ancestor with the taxa under consideration...
). We would predict that bats and monkeys are more closely related to each other than either is to a fish, because they both possess hair—a synapomorphy
Synapomorphy
In cladistics, a synapomorphy or synapomorphic character is a trait that is shared by two or more taxa and their most recent common ancestor, whose ancestor in turn does not possess the trait. A synapomorphy is thus an apomorphy visible in multiple taxa, where the trait in question originates in...
. However, we cannot say that bats and monkeys are more closely related to one another than they are to whales because they share hair, because we believe the last common ancestor of the three had hair.
However, the phenomena of convergent evolution
Convergent evolution
Convergent evolution describes the acquisition of the same biological trait in unrelated lineages.The wing is a classic example of convergent evolution in action. Although their last common ancestor did not have wings, both birds and bats do, and are capable of powered flight. The wings are...
, parallel evolution
Parallel evolution
Parallel evolution is the development of a similar trait in related, but distinct, species descending from the same ancestor, but from different clades.-Parallel vs...
, and evolutionary reversals (collectively termed homoplasy) add an unpleasant wrinkle to the problem of estimating phylogeny. For a number of reasons, two organisms can possess a trait not present in their last common ancestor: If we naively took the presence of this trait as evidence of a relationship, we would reconstruct an incorrect tree. Real phylogenetic data include substantial homoplasy, with different parts of the data suggesting sometimes very different relationships. Methods used to estimate phylogenetic trees are explicitly intended to resolve the conflict within the data by picking the phylogenetic tree that is the best fit to all the data overall, accepting that some data simply will not fit. It is often mistakenly believed that parsimony assumes that convergence is rare; in fact, even convergently-derived characters have some value in maximum-parsimony-based phylogenetic analyses, and the prevalence of convergence does not systematically affect the outcome of parsimony-based methods.
Data that do not fit a tree perfectly are not simply "noise", they can contain relevant phylogenetic signal in some parts of a tree, even if they conflict with the tree overall. In the whale example given above, the lack of hair in whales is homoplastic: It reflects a return to the condition present in ancient ancestors of mammals, who lacked hair. This similarity between whales and ancient mammal ancestors is in conflict with the tree we accept, since it implies that the mammals with hair should form a group excluding whales. However, among the whales, the reversal to hairlessness actually correctly associates the various types of whales (including dolphins and porpoises) into the group Cetacea
Cetacea
The order Cetacea includes the marine mammals commonly known as whales, dolphins, and porpoises. Cetus is Latin and is used in biological names to mean "whale"; its original meaning, "large sea animal", was more general. It comes from Ancient Greek , meaning "whale" or "any huge fish or sea...
. Still, the determination of the best-fitting tree—and thus which data do not fit the tree—is a complex process. Maximum parsimony is one method developed to do this.
Character data
The input data used in a maximum parsimony analysis is in the form of "characters" for a range of taxa. There is no generally agreed-upon definition of a phylogenetic character, but operationally a character can be thought of as an attribute, an axis along which taxa are observed to vary. These attributes can be physical (morphological), molecular, genetic, physiological, or behavioral. The only widespread agreement on characters seems to be that variation used for character analysis should reflect heritable variationGenotype
The genotype is the genetic makeup of a cell, an organism, or an individual usually with reference to a specific character under consideration...
. Whether it must be directly heritable, or whether indirect inheritance (e.g., learned behaviors) is acceptable, is not entirely resolved.
Each character is divided into discrete character states, into which the variations observed are classified. Character states are often formulated as descriptors, describing the condition of the character substrate. For example, the character "eye color" might have the states "blue" and "brown." Characters can have two or more states (they can have only one, but these characters lend nothing to a maximum parsimony analysis, and are often excluded).
Coding characters for phylogenetic analysis is not an exact science, and there are numerous complicating issues. Typically, taxa are scored with the same state if they are more similar to one another in that particular attribute than each is to taxa scored with a different state. This is not straightforward when character states are not clearly delineated or when they fail to capture all of the possible variation in a character. How would one score the previously mentioned character for a taxon (or individual) with hazel eyes? Or green? As noted above, character coding is generally based on similarity: Hazel and green eyes might be lumped with blue because they are more similar to that color (being light), and the character could be then recoded as "eye color: light; dark." Alternatively, there can be multi-state characters, such as "eye color: brown; hazel, blue; green."
Ambiguities in character state delineation and scoring can be a major source of confusion, dispute, and error in phylogenetic analysis using character data. Note that, in the above example, "eyes: present; absent" is also a possible character, which creates issues because "eye color" is not applicable if eyes are not present. For such situations, a "?" ("unknown") is scored, although sometimes "X" or "-" (the latter usually in sequence
Sequence
In mathematics, a sequence is an ordered list of objects . Like a set, it contains members , and the number of terms is called the length of the sequence. Unlike a set, order matters, and exactly the same elements can appear multiple times at different positions in the sequence...
data) are used to distinguish cases where a character cannot be scored from a case where the state is simply unknown. Current implementations of maximum parsimony generally treat unknown values in the same manner: the reasons the data are unknown have no particular effect on analysis. Effectively, the program treats a ? as if it held the state that would involve the fewest extra steps in the tree (see below), although this is not an explicit step in the algorithm.
Genetic data are particularly amenable to character-based phylogenetic methods such as maximum parsimony because protein and nucleotide sequences are naturally discrete: A particular position in a nucleotide sequence can be either adenine
Adenine
Adenine is a nucleobase with a variety of roles in biochemistry including cellular respiration, in the form of both the energy-rich adenosine triphosphate and the cofactors nicotinamide adenine dinucleotide and flavin adenine dinucleotide , and protein synthesis, as a chemical component of DNA...
, cytosine
Cytosine
Cytosine is one of the four main bases found in DNA and RNA, along with adenine, guanine, and thymine . It is a pyrimidine derivative, with a heterocyclic aromatic ring and two substituents attached . The nucleoside of cytosine is cytidine...
, guanine
Guanine
Guanine is one of the four main nucleobases found in the nucleic acids DNA and RNA, the others being adenine, cytosine, and thymine . In DNA, guanine is paired with cytosine. With the formula C5H5N5O, guanine is a derivative of purine, consisting of a fused pyrimidine-imidazole ring system with...
, or thymine
Thymine
Thymine is one of the four nucleobases in the nucleic acid of DNA that are represented by the letters G–C–A–T. The others are adenine, guanine, and cytosine. Thymine is also known as 5-methyluracil, a pyrimidine nucleobase. As the name suggests, thymine may be derived by methylation of uracil at...
/ uracil
Uracil
Uracil is one of the four nucleobases in the nucleic acid of RNA that are represented by the letters A, G, C and U. The others are adenine, cytosine, and guanine. In RNA, uracil binds to adenine via two hydrogen bonds. In DNA, the uracil nucleobase is replaced by thymine.Uracil is a common and...
, or a sequence gap; a position (residue
Residue (chemistry)
In chemistry, residue is the material remaining after a distillation or an evaporation, or to a portion of a larger molecule, such as a methyl group. It may also refer to the undesired byproducts of a reaction....
) in a protein sequence will be one of the basic amino acids or a sequence gap. Thus, character scoring is rarely ambiguous, except in cases where sequencing
Sequencing
In genetics and biochemistry, sequencing means to determine the primary structure of an unbranched biopolymer...
methods fail to produce a definitive assignment for a particular sequence position. Sequence gaps are sometimes treated as characters, although there is no consensus on how they should be coded.
Characters can be treated as unordered or ordered. For a binary (two-state) character, this makes little difference. For a multi-state character, unordered characters can be thought of as having an equal "cost" (in terms of number of "evolutionary events") to change from any one state to any other; complementarily, they do not require passing through intermediate states. Ordered characters have a particular sequence in which the states must occur through evolution, such that going between some states requires passing through an intermediate. This can be thought of complementarily as having different costs to pass between different pairs of states. In the eye-color example above, it is possible to leave it unordered, which imposes the same evolutionary "cost" to go from brown-blue, green-blue, green-hazel, etc. Alternatively, it could be ordered brown-hazel-green-blue; this would normally imply that it would cost two evolutionary events to go from brown-green, three from brown-blue, but only one from brown-hazel. This can also be thought of as requiring eyes to evolve through a "hazel stage" to get from brown to green, and a "green stage" to get from hazel to blue, etc.
There is a lively debate on the utility and appropriateness of character ordering, but no consensus. Some authorities order characters when there is a clear logical, ontogenetic, or evolutionary transition among the states (for example, "legs: short; medium; long"). Some accept only some of these criteria. Some run an unordered analysis, and order characters that show a clear order of transition in the resulting tree (which practice might be accused of circular reasoning
Circular reasoning
Circular reasoning, or in other words, paradoxical thinking, is a type of formal logical fallacy in which the proposition to be proved is assumed implicitly or explicitly in one of the premises. For example:"Only an untrustworthy person would run for office...
). Some authorities refuse to order characters at all, suggesting that it biases an analysis to require evolutionary transitions to follow a particular path.
It is also possible to apply differential weighting to individual characters. This is usually done relative to a "cost" of 1. Thus, some characters might be seen as more likely to reflect the true evolutionary relationships among taxa, and thus they might be weighted at a value 2 or more; changes in these characters would then count as two evolutionary "steps" rather than one when calculating tree scores (see below). There has been much discussion in the past about character weighting. Most authorities now weight all characters equally, although exceptions are common. For example, allele frequency
Allele frequency
Allele frequency or Gene frequency is the proportion of all copies of a gene that is made up of a particular gene variant . In other words, it is the number of copies of a particular allele divided by the number of copies of all alleles at the genetic place in a population. It can be expressed for...
data is sometimes pooled in bins and scored as an ordered character. In these cases, the character itself is often downweighted so that small changes in allele frequencies count less than major changes in other characters. Also, the third codon position in a coding nucleotide sequence is particularly labile, and is sometimes downweighted, or given a weight of 0, on the assumption that it is more likely to exhibit homoplasy. In some cases, repeated analyses are run, with characters reweighted in inverse proportion to the degree of homoplasy discovered in the previous analysis (termed successive weighting); this is another technique that might be considered circular reasoning
Circular reasoning
Circular reasoning, or in other words, paradoxical thinking, is a type of formal logical fallacy in which the proposition to be proved is assumed implicitly or explicitly in one of the premises. For example:"Only an untrustworthy person would run for office...
.
Character state changes can also be weighted individually. This is often done for nucleotide sequence data; it has been empirically determined that certain base changes (A-C, A-T, G-C, G-T, and the reverse changes) occur much less often than others. These changes are therefore often weighted more. As shown above in the discussion of character ordering, ordered characters can be thought of as a form of character state weighting.
Some systematists prefer to exclude characters known to be, or suspected to be, highly homoplastic or that have a large number of unknown entries ("?"). As noted below, theoretical and simulation work has demonstrated that this is likely to sacrifice accuracy rather than improve it. This is also the case with characters that are variable in the terminal taxa: theoretical, congruence, and simulation studies have all demonstrated that such polymorphic characters contain significant phylogenetic information.
Taxon sampling
The time required for a parsimony analysis (or any phylogenetic analysis) is proportional to the number of taxa (and characters) included in the analysis. Also, because more taxa require more branches to be estimated, more uncertainty may be expected in large analyses. Because data collection costs in time and money often scale directly with the number of taxa included, most analyses include only a fraction of the taxa that could have been sampled. Indeed, some authors have contended that four taxa (the minimum required to produce a meaningful unrooted tree) are all that is necessary for accurate phylogenetic analysis, and that more characters are more valuable than more taxa in phylogenetics. This has led to a raging controversy about taxon sampling.Empirical, theoretical, and simulation studies have led to a number of dramatic demonstrations of the importance of adequate taxon sampling. Most of these can be summarized by a simple observation: a phylogenetic data matrix has dimensions of characters times taxa. Doubling the number of taxa doubles the amount of information in a matrix just as surely as doubling the number of characters. Each taxon represents a new sample for every character, but, more importantly, it (usually) represents a new combination of character states. These character states can not only determine where that taxon is placed on the tree, they can inform the entire analysis, possibly causing different relationships among the remaining taxa to be favored by changing estimates of the pattern of character changes.
The most disturbing weakness of parsimony analysis, that of long-branch attraction (see below) is particularly pronounced with poor taxon sampling, especially in the four-taxon case. This is a well-understood case in which additional character sampling may not improve the quality of the estimate. As taxa are added, they often break up long branches (especially in the case of fossils), effectively improving the estimation of character state changes along them. Because of the richness of information added by taxon sampling, it is even possible to produce highly accurate estimates of phylogenies with hundreds of taxa using only a few thousand characters.
Although many studies have been performed, there is still much work to be done on taxon sampling strategies. Because of advances in computer performance, and the reduced cost and increased automation of molecular sequencing, sample sizes overall are on the rise, and studies addressing the relationships of hundreds of taxa (or other terminal entities, such as genes) are becoming common. Of course, this is not to say that adding characters is not also useful; the number of characters is increasing as well.
Some systematists prefer to exclude taxa based on the number of unknown character entries ("?") they exhibit, or because they tend to "jump around" the tree in analyses (i.e., they are "wildcards"). As noted below, theoretical and simulation work has demonstrated that this is likely to sacrifice accuracy rather than improve it. Although these taxa may generate more most-parsimonious trees (see below), methods such as agreement subtrees and reduced consensus can still extract information on the relationships of interest.
It has been observed that inclusion of more taxa tends to lower overall support values (bootstrap
Bootstrapping (statistics)
In statistics, bootstrapping is a computer-based method for assigning measures of accuracy to sample estimates . This technique allows estimation of the sample distribution of almost any statistic using only very simple methods...
percentages or decay indices, see below). The cause of this is clear: as additional taxa are added to a tree, they subdivide the branches to which they attach, and thus dilute the information that supports that branch. While support for individual branches is reduced, support for the overall relationships is actually increased. Consider analysis that produces the following tree: (fish ,(lizard ,(whale, (cat, monkey)))). Adding a rat and a walrus will probably reduce the support for the (whale, (cat, monkey)) clade, because the rat and the walrus may fall within this clade, or outside of the clade, and since these five animals are all relatively closely related, there should be more uncertainty about their relationships. Within error, it may be impossible to determine any of these animals' relationships relative to one another. However, the rat and the walrus will probably add character data that cements the grouping any two of these mammals exclusive of the fish or the lizard; where the initial analysis might have been misled, say, by the presence of fins in the fish and the whale, the presence of the walrus, with blubber and fins like a whale but whiskers like a cat and a rat, firmly ties the whale to the mammals.
To cope with this problem, agreement subtrees, reduced consensus, and double-decay analysis seek to identify supported relationships (in the form of "n-taxon statements," such as the four-taxon statement "(fish, (lizard, (cat, whale)))") rather than whole trees. If the goal of an analysis is a resolved tree, as is the case for comparative phylogenetics, these methods cannot solve the problem. However, if the tree estimate is so poorly supported, the results of any analysis derived from the tree will probably be too suspect to use anyway.
Analysis
A maximum parsimony analysis runs in a very straightforward fashion. Trees are scored according to the degree to which they imply a parsimonious distribution of the character data. The most parsimonious tree for the dataset represents the preferred hypothesis of relationships among the taxa in the analysis.Trees are scored (evaluated) by using a simple algorithm to determine how many "steps" (evolutionary transitions) are required to explain the distribution of each character. A step is, in essence, a change from one character state to another, although with ordered characters some transitions require more than one step. Contrary to popular belief, the algorithm does not explicitly assign particular character states to nodes (branch junctions) on a tree: the least number of steps can involve multiple, equally costly assignments and distributions of evolutionary transitions. What is optimized is the total number of changes.
There are many more possible phylogenetic tree
Phylogenetic tree
A phylogenetic tree or evolutionary tree is a branching diagram or "tree" showing the inferred evolutionary relationships among various biological species or other entities based upon similarities and differences in their physical and/or genetic characteristics...
s than can be searched exhaustively for more than eight taxa or so. A number of algorithms are therefore used to searching among the possible trees. Many of these involve taking an initial tree (usually the favored tree from the last iteration of the algorithm), and perturbing it to see if the change produces a higher score.
The trees resulting from parsimony search are unrooted: They show all the possible relationships of the included taxa, but they lack any statement on relative times of divergence. A particular branch is chosen to root the tree by the user. This branch is then taken to be outside all the other branches of the tree, which together form a monophyletic group. This imparts a sense of relative time to the tree. Incorrect choice of a root can result in incorrect relationships on the tree, even if the tree is itself correct in its unrooted form.
Parsimony analysis often returns a number of equally most-parsimonious trees (MPTs). A large number of MPTs is often seen as an analytical failure, and is widely believed to be related to the number of missing entries ("?") in the dataset, characters showing too much homoplasy, or the presence of topologically labile "wildcard" taxa (which may have many missing entries). Numerous methods have been proposed to reduce the number of MPTs, including removing characters or taxa with large amounts of missing data before analysis, removing or downweighting highly homoplastic characters (successive weighting) or removing wildcard taxa (the phylogenetic trunk method) a posteriori
A priori and a posteriori (philosophy)
The terms a priori and a posteriori are used in philosophy to distinguish two types of knowledge, justifications or arguments...
and then reanalyzing the data.
Numerous theoretical and simulation studies have demonstrated that highly homoplastic characters, characters and taxa with abundant missing data, and "wildcard" taxa contribute to the analysis. Although excluding characters or taxa may appear to improve resolution, the resulting tree is based on less data, and is therefore a less reliable estimate of the phylogeny (unless the characters or taxa are non informative, see safe taxonomic reduction). Today's general consensus is that having multiple MPTs is a valid analytical result; it simply indicates that there is insufficient data to resolve the tree completely. In many cases, there is substantial common structure in the MPTs, and differences are slight and involve uncertainty in the placement of a few taxa. There are a number of methods for summarizing the relationships within this set, including consensus trees, which show common relationships among all the taxa, and pruned agreement subtrees, which show common structure by temporarily pruning "wildcard" taxa from every tree until they all agree. Reduced consensus takes this one step further, but showing all subtrees (and therefore all relationships) supported by the input trees.
Even if multiple MPTs are returned, parsimony analysis still basically produces a point-estimate, lacking confidence interval
Confidence interval
In statistics, a confidence interval is a particular kind of interval estimate of a population parameter and is used to indicate the reliability of an estimate. It is an observed interval , in principle different from sample to sample, that frequently includes the parameter of interest, if the...
s of any sort. This has often been levelled as a criticism, since there is certainly error in estimating the most-parsimonious tree, and the method does not inherently include any means of establishing how sensitive its conclusions are to this error. Several methods have been used to assess support.
Jackknifing and bootstrapping
Bootstrapping (statistics)
In statistics, bootstrapping is a computer-based method for assigning measures of accuracy to sample estimates . This technique allows estimation of the sample distribution of almost any statistic using only very simple methods...
, well-known statistical resampling
Resampling (statistics)
In statistics, resampling is any of a variety of methods for doing one of the following:# Estimating the precision of sample statistics by using subsets of available data or drawing randomly with replacement from a set of data points # Exchanging labels on data points when performing significance...
procedures, have been employed with parsimony analysis. The jackknife, which involves resampling without replacement ("leave-one-out") can be employed on characters or taxa; interpretation may become complicated in the latter case, because the variable of interest is the tree, and comparison of trees with different taxa is not straightforward. The bootstrap, resampling with replacement (sample x items randomly out of a sample of size x, but items can be picked multiple times), is only used on characters, because adding duplicate taxa does not change the result of a parsimony analysis. The bootstrap is much more commonly employed in phylogenetics (as elsewhere); both methods involve an arbitrary but large number of repeated iterations involving perturbation of the original data followed by analysis. The resulting MPTs from each analysis are pooled, and the results are usually presented on a 50% Majority Rule Consensus tree, with individual branches (or nodes) labelled with the percentage of bootstrap MPTs in which they appear. This "bootstrap percentage" (which is not a P-value
P-value
In statistical significance testing, the p-value is the probability of obtaining a test statistic at least as extreme as the one that was actually observed, assuming that the null hypothesis is true. One often "rejects the null hypothesis" when the p-value is less than the significance level α ,...
, as is sometimes claimed) is used as a measure of support. Technically, it is supposed to be a measure of repeatability, the probability that that branch (node, clade) would be recovered if the taxa were sampled again. Experimental tests with viral phylogenies suggest that the bootstrap percentage is not a good estimator of repeatability for phylogenetics, but it is a reasonable estimator of accuracy. In fact, it has been shown that the bootstrap percentage, as an estimator of accuracy, is biased, and that this bias results on average in an underestimate of confidence (such that as little as 70% support might really indicate up to 95% confidence). However, the direction of bias cannot be ascertained in individual cases, so assuming that high values bootstrap support indicate even higher confidence is unwarranted.
Another means of assessing support is Bremer support, or the decay index (which is technically not an index). This is simply the difference in number of steps between the score of the MPT(s), and the score of the most parsimonious tree that does NOT contain a particular clade (node, branch). It can be thought of as the number of steps you have to add to lose that clade; implicitly, it is meant to suggest how great the error in the estimate of the score of the MPT must be for the clade to no longer be supported by the analysis, although this is not necessarily what it does. Decay index values are often fairly low (one or two steps being typical), but they often appear to be proportional to bootstrap percentages. However, interpretation of decay values is not straightforward, and they seem to be preferred by authors with philosophical objections to the bootstrap (although many morphological systematists, especially paleontologists, report both). Double-decay analysis is a decay counterpart to reduced consensus that evaluates the decay index for all possible subtree relationships (n-taxon statements) within a tree.
Problems with maximum parsimony phylogeny estimation
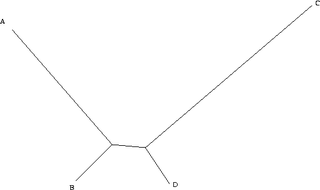
Statistics
Statistics is the study of the collection, organization, analysis, and interpretation of data. It deals with all aspects of this, including the planning of data collection in terms of the design of surveys and experiments....
. As demonstrated in 1978 by Joe Felsenstein
Joe Felsenstein
Joseph "Joe" Felsenstein is Professor in the Departments of Genome Sciences and Biology and Adjunct Professor in the Departments of Computer Science and Statistics at the University of Washington in Seattle...
, maximum parsimony can be inconsistent under certain conditions. The category of situations in which this is known to occur is called long branch attraction
Long branch attraction
Long branch attraction is a phenomenon in phylogenetic analyses when rapidly evolving lineages are inferred to be closely related, regardless of their true evolutionary relationships. For example, in DNA sequence-based analyses, the problem arises when sequences from two lineages evolve rapidly...
, and occurs, for example, where there are long branches (a high level of substitutions) for two characters (A & C), but short branches for another two (B & D). A and B diverged from a common ancestor, as did C and D.
Assume for simplicity that we are considering a single binary character (it can either be + or -). Because the distance from B to D is small, in the vast majority of all cases, B and D will be the same. Here, we will assume that they are both + (+ and - are assigned arbitrarily and swapping them is only a matter of definition). If this is the case, there are four remaining possibilities. A and C can both be +, in which case all taxa are the same and all the trees have the same length. A can be + and C can be -, in which case only one character is different, and we cannot learn anything, as all trees have the same length. Similarly, A can be - and C can be +. The only remaining possibility is that A and C are both -. In this case, however, we group A and C together, and B and D together. As a consequence, when we have a tree of this type, the more data we collect (i.e. the more characters we study), the more we tend towards the wrong tree.
A simple and effective method for determining whether or not long branch attraction is affecting tree topology is the SAW method, named for Siddal and Whiting. If long branch attraction is suspected in a pair of taxa (A and B), simply remove taxon A ("saw" off the branch) and re-run the analysis. Then remove A and replace B, running the analysis again. If either of the taxa appear at different branch points in the absence of the other, there is evidence of long branch attraction. Since long branches can't possibly attract one another when only one is in the analysis, consistent taxon placement between treatments would indicate long branch attraction is not a problem.
Several other methods of phylogeny estimation are available, including maximum likelihood
Maximum likelihood
In statistics, maximum-likelihood estimation is a method of estimating the parameters of a statistical model. When applied to a data set and given a statistical model, maximum-likelihood estimation provides estimates for the model's parameters....
, Bayesian phylogeny inference
Bayesian inference in phylogeny
Bayesian inference in phylogeny generates a posterior distribution for a parameter, composed of a phylogenetic tree and a model of evolution, based on the prior for that parameter and the likelihood of the data, generated by a multiple alignment. The Bayesian approach has become more popular due...
, neighbour joining, and quartet methods. Of these, the first two both use a likelihood function
Likelihood function
In statistics, a likelihood function is a function of the parameters of a statistical model, defined as follows: the likelihood of a set of parameter values given some observed outcomes is equal to the probability of those observed outcomes given those parameter values...
, and, if used properly, are theoretically immune to long-branch attraction. These methods are both parametric
Parametric
Parametric may refer to:*Parametric equation*Parametric statistics*Parametric derivative*Parametric plot*Parametric model*Parametric oscillator *Parametric contract*Parametric insurance*Parametric feature based modeler...
, meaning that they rely on an explicit model of character evolution. It has been shown that, for some suboptimal models, these methods can also be inconsistent.
Another complication with maximum parsimony is that finding the most parsimonious tree is an NP-Hard
NP-hard
NP-hard , in computational complexity theory, is a class of problems that are, informally, "at least as hard as the hardest problems in NP". A problem H is NP-hard if and only if there is an NP-complete problem L that is polynomial time Turing-reducible to H...
problem. The only currently available, efficient way of obtaining a solution, given an arbitrarily large set of taxa, is by using heuristic methods which do not guarantee that the most parsimonious tree will be recovered. These methods employ hill-climbing algorithms to progressively approach the best tree. However, it has been shown that there can be "tree islands" of suboptimal solutions, and the analysis can become trapped in these local optima. Thus, complex, flexible heuristics are required to ensure that tree space has been adequately explored. Several heuristics are available, including nearest neighbor interchange (NNI), tree bisection / reconnection (TBR), and the phylogenetic ratchet. This problem is certainly not unique to MP; any method that uses an optimality criterion faces the same problem, and none offer easy solutions.
Criticism
It has been asserted that a major problem, especially for paleontologyPaleontology
Paleontology "old, ancient", ὄν, ὀντ- "being, creature", and λόγος "speech, thought") is the study of prehistoric life. It includes the study of fossils to determine organisms' evolution and interactions with each other and their environments...
, is that maximum parsimony assumes that the only way two species can share the same nucleotide at the same position is if they are genetically related. This asserts that phylogenetic applications of parsimony assume that all similarity is homologous (other interpretations, such as the assertion that two organisms might NOT be related at all, are nonsensical). This is emphatically not the case: as with any form of character-based phylogeny estimation, parsimony is used to test the homologous nature of similarities by finding the phylogenetic tree which best accounts for all of the similarities.
For example, birds and bats have wings, while crocodiles and humans do not. If these were the only data available, maximum parsimony would tend to group crocodiles with humans, and birds with bats (as would any other method of phylogenetic inference). We believe that humans are actually more closely related to bats than to crocodiles or birds. Our belief is founded on additional data that were not considered in the one-character example (using wings). If even a tiny fraction of these additional data, including information on skeletal structure, soft-tissue
Soft tissue
In anatomy, the term soft tissue refers to tissues that connect, support, or surround other structures and organs of the body, not being bone. Soft tissue includes tendons, ligaments, fascia, skin, fibrous tissues, fat, and synovial membranes , and muscles, nerves and blood vessels .It is sometimes...
morphology, integument, behaviour, genetics, etc., were included in the analysis, the faint phylogenetic signal produced by the presence of wings in birds and bats would be overwhelmed by the preponderance of data supporting the (human, bat)(bird, crocodile) tree.
It is often stated that parsimony is not relevant to phylogenetic inference because "evolution is not parsimonious." In most cases, there is no explicit alternative proposed; if no alternative is available, any statistical method is preferable to none at all. Additionally, it is not clear what would be meant if the statement "evolution is parsimonious" were in fact true. This could be taken to mean that more character changes may have occurred historically than are predicted using the parsimony criterion. Because parsimony phylogeny estimation reconstructs the minimum number of changes necessary to explain a tree, this is quite possible. However, it has been shown through simulation studies, testing with known in vitro
In vitro
In vitro refers to studies in experimental biology that are conducted using components of an organism that have been isolated from their usual biological context in order to permit a more detailed or more convenient analysis than can be done with whole organisms. Colloquially, these experiments...
viral phylogenies, and congruence with other methods, that the accuracy of parsimony is in most cases not compromised by this. Parsimony analysis uses the number of character changes on trees to choose the best tree, but it does not require that exactly that many changes, and no more, produced the tree. As long as the changes that have not been accounted for are randomly distributed over the tree (a reasonable null expectation), the result should not be biased. In practice, the technique is robust: maximum parsimony exhibits minimal bias as a result of choosing the tree with the fewest changes.
An analogy can be drawn with choosing among contractors based on their initial (nonbinding) estimate of the cost of a job. The actual finished cost is very likely to be higher than the estimate. Despite this, choosing the contractor who furnished the lowest estimate should theoretically result in the lowest final project cost. This is because, in the absence of other data, we would assume that all of the relevant contractors have the same risk of cost overruns. In practice, of course, unscrupulous business practices may bias this result; in phylogenetics, too, some particular phylogenetic problems (for example, long branch attraction
Long branch attraction
Long branch attraction is a phenomenon in phylogenetic analyses when rapidly evolving lineages are inferred to be closely related, regardless of their true evolutionary relationships. For example, in DNA sequence-based analyses, the problem arises when sequences from two lineages evolve rapidly...
, described above) may potentially bias results. In both cases, however, there is no way to tell if the result is going to be biased, or the degree to which it will be biased, based on the estimate itself. With parsimony too, there is no way to tell that the data are positively misleading, without comparison to other evidence.
Along the same lines, parsimony is often characterized as implicitly adopting the philosophical position that evolutionary change is rare, or that homoplasy (convergence and reversal) is minimal in evolution. This is not entirely true: parsimony minimizes the number of convergences and reversals that are assumed by the preferred tree, but this may result in a relatively large number of such homoplastic events. It would be more appropriate to say that parsimony assumes only the minimum amount of change implied by the data. As above, this does not require that these were the only changes that occurred; it simply does not infer changes for which there is no evidence. The shorthand for describing this is that "parsimony minimizes assumed homoplasies, it does not assume that homoplasy is minimal."
Parsimony is also sometimes associated with the notion that "the simplest possible explanation is the best," a generalisation of Occam's Razor
Occam's razor
Occam's razor, also known as Ockham's razor, and sometimes expressed in Latin as lex parsimoniae , is a principle that generally recommends from among competing hypotheses selecting the one that makes the fewest new assumptions.-Overview:The principle is often summarized as "simpler explanations...
. Parsimony does prefer the solution that requires the least number of unsubstantiated assumptions and unsupportable conclusions, the solution that goes the least theoretical distance beyond the data. This is a very common approach to science, especially when dealing with systems that are so complex as to defy simple models. Parsimony does not by any means necessarily produce a "simple" assumption. Indeed, as a general rule, most character datasets are so "noisy" that no truly "simple" solution is possible.
Alternatives
There are several other methods for inferring phylogenies based on discrete character data. Each offers potential advantages and disadvantages. Most of these methods have particularly avid proponents and detractors; parsimony especially has been advocated as philosophically superior (most notably by ardent cladists).Maximum likelihood
Among the most popular alternative phylogenetic methods is maximum likelihood phylogenetic inference, sometimes simply called "likelihood" or "ML." Maximum likelihood is an optimality criterion, as is parsimony. Mechanically, maximum likelihood analysis functions much like parsimony analysis, in that trees are scored based on a character dataset, and the tree with the best score is selected. Maximum likelihood is a parametric statistical methodParametric statistics
Parametric statistics is a branch of statistics that assumes that the data has come from a type of probability distribution and makes inferences about the parameters of the distribution. Most well-known elementary statistical methods are parametric....
, in that it employs an explicit model of character evolution. Such methods are potentially much more powerful than non-parametric statistical methods
Non-parametric statistics
In statistics, the term non-parametric statistics has at least two different meanings:The first meaning of non-parametric covers techniques that do not rely on data belonging to any particular distribution. These include, among others:...
like parsimony, but only if the model used is a reasonable approximation of the processes that produced the data. Maximum likelihood has probably surpassed parsimony in popularity with nucleotide sequence data, and Bayesian phylogenetic inference
Bayesian inference in phylogeny
Bayesian inference in phylogeny generates a posterior distribution for a parameter, composed of a phylogenetic tree and a model of evolution, based on the prior for that parameter and the likelihood of the data, generated by a multiple alignment. The Bayesian approach has become more popular due...
, which uses the likelihood function, is becoming almost as prevalent.
Likelihood
Likelihood
Likelihood is a measure of how likely an event is, and can be expressed in terms of, for example, probability or odds in favor.-Likelihood function:...
is the relative counterpart to absolute probability
Probability
Probability is ordinarily used to describe an attitude of mind towards some proposition of whose truth we arenot certain. The proposition of interest is usually of the form "Will a specific event occur?" The attitude of mind is of the form "How certain are we that the event will occur?" The...
. If we know the number of possible outcomes of a test (N), and we know the number of those outcomes that fit a particular criterion (n), we can say that the probability of that criterion being met by an execution of that test is n/N. Thus, the probability of heads in the toss of a fair coin
Coin flipping
Coin flipping or coin tossing or heads or tails is the practice of throwing a coin in the air to choose between two alternatives, sometimes to resolve a dispute between two parties...
is 50% (1/2). What if we don't know the number of possible outcomes? Obviously, we cannot then calculate probabilities. However, if we observe that one outcome happens twice as often as the other over an arbitrarily large number of tests, we can say that that outcome is twice as likely. Likelihoods are proportional to the true probabilities: if an outcome is twice as likely, we can say that it is twice as probable, even though we cannot say how probable it is.
Practically, the probability of a tree cannot be calculated directly. The probability of the data given a tree can be calculated if you assume a specific set of probabilities of character change (a model). The critical part of likelihood analysis is that the probability of the data given the tree is the likelihood of the tree given the data. Thus, the tree that has the highest probability of producing the observed data is the most likely tree.
Maximum likelihood, as implemented in phylogenetics, uses a stochastic model that gives the probability of a particular character changing at any given point on a tree. This model can have a potentially large number of parameters, which can account for differences in the probabilities of particular states, the probabilities of particular changes, and differences in the probabilities of change among characters.
A likelihood tree has meaningful branch lengths (i.e. it is a phylogram); these lengths are usually interpreted as being proportional to the average probability of change for characters on that branch (thus, on a branch of length 1, we would expect an average of one change per character, which is a lot). The state of each character is plotted on the tree, and the probability of that distribution of character states is calculated using the model and the branch lengths (which can be altered to maximize the probability of the data). This is the probability of that character, given the tree. The probabilities of all of the characters is multiplied together; they are usually negative log-transformed and added (producing the same effect), because the numbers become very small very quickly. This sum is the probability of the data, given the tree, or the likelihood of the tree. The tree with the highest likelihood (lowest negative log-transformed likelihood) given the data is preferred.
In the above analogy regarding choosing a contractor, maximum likelihood would be analogous to gathering data on the final cost of broadly comparable jobs performed by each contractor over the past year, and selecting the contractor with the lowest average cost for those comparable jobs. This method would be highly dependent on how comparable the jobs are, but, if they are properly chosen, it will produce a better estimate of the actual cost of the job. Further, it would not be misled by bias in contractor estimates, because it is based on the final cost, not on the (potentially biased) estimates.
In practice, maximum likelihood tends to favor trees that are very similar to the most parsimonious tree(s) for the same dataset. It has been shown to outperform parsimony in certain situations where the latter is known to be biased, including long-branch attraction. Note, however, that the performance of likelihood is dependent on the quality of the model employed; an incorrect model can produce a biased result. Studies have shown that incorporating a parameter to account for differences in rate of evolution among characters is often critical to accurate estimation of phylogenies; failure to model this or other crucial parameters may produce incorrect or biased results. Model parameters are usually estimated from the data, and the number (and type) of parameters is often determined using the hierarchical likelihood ratio test. The consequences of mis-specified models are just beginning to be explored in detail.
Likelihood is generally regarded as a more desirable method than parsimony, in that it is statistically consistent
Consistent estimator
In statistics, a sequence of estimators for parameter θ0 is said to be consistent if this sequence converges in probability to θ0...
, and has a better statistical foundation, and because it allows complex modeling of evolutionary processes. A major drawback is that ML is still quite slow relative to parsimony methods, sometimes requiring days to run large datasets. Maximum likelihood phylogenetic inference was proposed in the mid-Twentieth Century, but it has only been a popular method for phylogenetic inference since the 1990s, when computational power caught up with tremendous demands of ML analysis. Newer algorithms and implementations are bringing analysis times for large datasets into acceptable ranges. Until these methods gain widespread acceptance, parsimony will probably be preferred for extremely large datasets, especially when bootstrapping is used to assess confidence in the results.
One area where parsimony still holds much sway is in the analysis of morphological data. Until recently, stochastic models of character change were not available for non-molecular data. New methods, proposed by Paul Lewis, make essentially the same assumptions that parsimony analysis does, but do so within a likelihood framework. These models are not, however, widely implemented, and, unless modified, they require the modification of existing datasets (to deal with ordered characters, and the tendency to not record autapomorphies in morphological datasets.
Maximum likelihood has been criticised as assuming neutral evolution implicitly in its adoption of a stochastic model of evolution. This is not necessarily the case: as with parsimony, assuming a stochastic model does not presume that all evolution is stochastic. In practice, likelihood is robust to deviations from stochasticity. It performs well even on coding sequences that include sites believed to be under selection.
A related objection (often brought up by parsimony-only advocates) is the idea that evolution is too complex or too poorly understood to be modeled. This objection probably rests on a misunderstanding of the term "model." While it is customary to think of models as representing the mechanics of a process, this is not necessarily literally the case. In fact, a model is often selected not so much for its faithful reproduction of the phenomenon as its ability to make predictions. In practice, it is best not to try and exactly fit a model to a process, because there is a trade-off between number of parameters in a model and its statistical power
Statistical power
The power of a statistical test is the probability that the test will reject the null hypothesis when the null hypothesis is actually false . The power is in general a function of the possible distributions, often determined by a parameter, under the alternative hypothesis...
. Stochasticity may be a reasonably good fit to evolutionary data at a broad level, even if it does not accurately mirror the process at finer scales.
By analogy, no one claims that the human foot varies only in length and width, but differing combinations of length and width values can be combined to fit a wide variety of feet. In some cases, a slightly wider overall foot may be better fitted by increasing overall size rather than instep width, while a foot with a narrower heel might be better fit by a wider instep and a smaller shoe. Adding several more measurements would probably improve shoe fit somewhat, but would be impractical from a business standpoint. With increasingly precise fitting, differences between feet would make selling matched pairs of shoes impossible, and differences through time would mean that a proper fit at purchase might not be a proper fit when worn.
Parsimony has recently been shown to be more likely to recover the true tree in the face of profound changes in evolutionary ("model") parameters (e.g., the rate of evolutionary change) within a tree . This is particularly troublesome, since it is generally agreed that such changes may be a significant feature of deep divergences. Likelihood has had substantial success recovering known in vitro viral phylogenies, simulated phylogenies, and phylogenies confirmed by other method. It seems likely therefore that this potential complication does not strongly bias results for more shallow divergences. Several research groups are currently exploring ways to incorporate profound shifts in evolutionary parameters into likelihood analysis.
Bayesian phylogenetic inference
Bayesian phylogenetics uses the likelihood function, and is normally implemented using the same models of evolutionary change used in Maximum Likelihood. It is very different, however, in both theory and application. Bayesian phylogenetic analysis uses Bayes' theoremBayes' theorem
In probability theory and applications, Bayes' theorem relates the conditional probabilities P and P. It is commonly used in science and engineering. The theorem is named for Thomas Bayes ....
, which relates the posterior probability
Posterior probability
In Bayesian statistics, the posterior probability of a random event or an uncertain proposition is the conditional probability that is assigned after the relevant evidence is taken into account...
of a tree to the likelihood of data, and the prior probability
Prior probability
In Bayesian statistical inference, a prior probability distribution, often called simply the prior, of an uncertain quantity p is the probability distribution that would express one's uncertainty about p before the "data"...
of the tree and model of evolution. However, unlike parsimony and likelihood methods, Bayesian analysis
Bayesian inference
In statistics, Bayesian inference is a method of statistical inference. It is often used in science and engineering to determine model parameters, make predictions about unknown variables, and to perform model selection...
does not produce a single tree or set of equally optimal trees. Bayesian analysis uses the likelihood of trees in a Markov chain Monte Carlo
Markov chain Monte Carlo
Markov chain Monte Carlo methods are a class of algorithms for sampling from probability distributions based on constructing a Markov chain that has the desired distribution as its equilibrium distribution. The state of the chain after a large number of steps is then used as a sample of the...
(MCMC) simulation to sample trees in proportion to their likelihood, thereby producing a credible sample of trees.
One commonly cited drawback of Bayesian analysis is the need to explicitly set out a set of prior probabilities for the range of potential outcomes. The idea of incorporating prior probabilities into an analysis has been suggested as a potential source of bias. Bayesian methods involve other potential issues, such as the evaluation of "convergence," the point at which the MCMC process stops searching for the "space" of credible solutions and begins to build the credible sample.
Distance matrix methods
Non-parametric distance methods were originally applied to phenetic data using a matrix of pairwise distances. These distances are then reconciled to produce a tree (a phylogram, with informative branch lengths). The distance matrixDistance matrix
In mathematics, computer science and graph theory, a distance matrix is a matrix containing the distances, taken pairwise, of a set of points...
can come from a number of different sources, including measured distance (for example from immunological studies
Immunology
Immunology is a broad branch of biomedical science that covers the study of all aspects of the immune system in all organisms. It deals with the physiological functioning of the immune system in states of both health and diseases; malfunctions of the immune system in immunological disorders ; the...
) or morphometric analysis, various pairwise distance formulae (such as euclidean distance
Euclidean distance
In mathematics, the Euclidean distance or Euclidean metric is the "ordinary" distance between two points that one would measure with a ruler, and is given by the Pythagorean formula. By using this formula as distance, Euclidean space becomes a metric space...
) applied to discrete morphological characters, or genetic distance
Genetic distance
Genetic distance refers to the genetic divergence between species or between populations within a species. It is measured by a variety of parameters. Smaller genetic distances indicate a close genetic relationship whereas large genetic distances indicate a more distant genetic relationship...
from sequence, restriction fragment
Restriction fragment
A restriction fragment is a DNA fragment resulting from the cutting of a DNA strand by a restriction enzyme , a process called restriction. Each restriction enzyme is highly specific, recognising a particular short DNA sequence, or restriction site, and cutting both DNA strands at specific points...
, or allozyme
Allozyme
Variant forms of an enzyme that are coded by different alleles at the same locus are called allozymes. These are opposed to isozymes, which are enzymes that perform the same function, but which are coded by genes located at different loci....
data. For phylogenetic character data, raw distance values can be calculated by simply counting the number of pairwise differences in character states (Manhattan distance).
Several simple algorithms exist to construct a tree directly from pairwise distances, including UPGMA
UPGMA
UPGMA is a simple agglomerative or hierarchical clustering method used in bioinformatics for the creation of phenetic trees...
and neighbor joining (NJ), but these will not necessarily produce the best tree for the data. UPGMA assumes an ultrametric tree (a tree where all the path-lengths from the root to the tips are equal). Neighbor-joining
Neighbor-joining
In bioinformatics, neighbor joining is a bottom-up clustering method for the creation of phenetic trees , created by Naruya Saitou and Masatoshi Nei...
is a form of star decomposition, and can very quickly produce reasonable trees. It is very often used on its own, and in fact quite frequently produces reasonable trees.
Phylogeny estimation using distance methods has produced a number of controversies. The relationship between individual characters and the tree is lost in the process of reducing characters to distances. Since these methods do not use character data directly, and information locked in the distribution of character states can be lost in the pairwise comparison
Pairwise comparison
Pairwise comparison generally refers to any process of comparing entities in pairs to judge which of each entity is preferred, or has a greater amount of some quantitative property. The method of pairwise comparison is used in the scientific study of preferences, attitudes, voting systems, social...
s. Also, some complex phylogenetic relationships may produce biased distances. Despite these potential problems, distance methods are extremely fast, and they often produce a reasonable estimate of phylogeny. They also have certain benefits over the methods that use characters directly. Notably, distance methods allow use of data that may not be easily converted to character data, such as DNA-DNA hybridization assays.