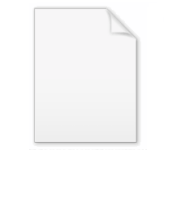
Generalized method of moments
Encyclopedia
In econometrics
, generalized method of moments (GMM) is a generic method for estimating parameters in statistical model
s. Usually it is applied in the context of semiparametric models, where the parameter of interest is finite-dimensional, whereas the full shape of the distribution function of the data may not be known, and therefore the maximum likelihood estimation is not applicable.
The method requires that a certain number of moment conditions were specified for the model. These moment conditions are functions of the model parameters and the data, such that their expectation
is zero at the true values of the parameters. The GMM method then minimizes a certain norm
of the sample averages of the moment conditions.
The GMM estimator
s are known to be consistent
, asymptotically normal
, and efficient in the class of all estimators that don’t use any extra information aside from that contained in the moment conditions.
GMM was developed by Lars Peter Hansen
in 1982 as a generalization of the method of moments.
. The data comes from a certain statistical model
, defined up to an unknown parameter
. The goal of the estimation problem is to find the “true” value of this parameter, θ0, or at least a reasonably close estimate.
In order to apply GMM there should exist a vector-valued function g(Y,θ) such that
Econometrics
Econometrics has been defined as "the application of mathematics and statistical methods to economic data" and described as the branch of economics "that aims to give empirical content to economic relations." More precisely, it is "the quantitative analysis of actual economic phenomena based on...
, generalized method of moments (GMM) is a generic method for estimating parameters in statistical model
Statistical model
A statistical model is a formalization of relationships between variables in the form of mathematical equations. A statistical model describes how one or more random variables are related to one or more random variables. The model is statistical as the variables are not deterministically but...
s. Usually it is applied in the context of semiparametric models, where the parameter of interest is finite-dimensional, whereas the full shape of the distribution function of the data may not be known, and therefore the maximum likelihood estimation is not applicable.
The method requires that a certain number of moment conditions were specified for the model. These moment conditions are functions of the model parameters and the data, such that their expectation
Expected value
In probability theory, the expected value of a random variable is the weighted average of all possible values that this random variable can take on...
is zero at the true values of the parameters. The GMM method then minimizes a certain norm
Norm (mathematics)
In linear algebra, functional analysis and related areas of mathematics, a norm is a function that assigns a strictly positive length or size to all vectors in a vector space, other than the zero vector...
of the sample averages of the moment conditions.
The GMM estimator
Estimator
In statistics, an estimator is a rule for calculating an estimate of a given quantity based on observed data: thus the rule and its result are distinguished....
s are known to be consistent
Consistent estimator
In statistics, a sequence of estimators for parameter θ0 is said to be consistent if this sequence converges in probability to θ0...
, asymptotically normal
Asymptotic distribution
In mathematics and statistics, an asymptotic distribution is a hypothetical distribution that is in a sense the "limiting" distribution of a sequence of distributions...
, and efficient in the class of all estimators that don’t use any extra information aside from that contained in the moment conditions.
GMM was developed by Lars Peter Hansen
Lars Peter Hansen
Lars Peter Hansen is an economist at the University of Chicago.- Biography :After graduating from Utah State University and the University of Minnesota Lars Peter Hansen (b. October 26, 1952 in Champaign, Illinois) is an economist at the University of Chicago.- Biography :After graduating from...
in 1982 as a generalization of the method of moments.
Description
Suppose the available data consists of T of iid observations , where each observation Yt is an n-dimensional multivariate random variableMultivariate random variable
In mathematics, probability, and statistics, a multivariate random variable or random vector is a list of mathematical variables each of whose values is unknown, either because the value has not yet occurred or because there is imperfect knowledge of its value.More formally, a multivariate random...
. The data comes from a certain statistical model
Statistical model
A statistical model is a formalization of relationships between variables in the form of mathematical equations. A statistical model describes how one or more random variables are related to one or more random variables. The model is statistical as the variables are not deterministically but...
, defined up to an unknown parameter
Parameter
Parameter from Ancient Greek παρά also “para” meaning “beside, subsidiary” and μέτρον also “metron” meaning “measure”, can be interpreted in mathematics, logic, linguistics, environmental science and other disciplines....
. The goal of the estimation problem is to find the “true” value of this parameter, θ0, or at least a reasonably close estimate.
In order to apply GMM there should exist a vector-valued function g(Y,θ) such that
-
where E denotes expectationExpected valueIn probability theory, the expected value of a random variable is the weighted average of all possible values that this random variable can take on...
, and Yt is a generic observation, which are all assumed to be iid. Moreover, function m(θ) must not be equal to zero for , or otherwise parameter θ will not be identified.
The basic idea behind GMM is to replace the theoretical expected value E[⋅] with its empirical analog — sample average:-
and then to minimize the norm of this expression with respect to θ.
By the law of large numbersLaw of large numbersIn probability theory, the law of large numbers is a theorem that describes the result of performing the same experiment a large number of times...
, for large values of T, and thus we expect that . The generalized method of moments looks for a number which would make as close to zero as possible. Mathematically, this is equivalent to minimizing a certain normNorm (mathematics)In linear algebra, functional analysis and related areas of mathematics, a norm is a function that assigns a strictly positive length or size to all vectors in a vector space, other than the zero vector...
of (norm of m, denoted as ||m||, measures the distance between m and zero). The properties of the resulting estimator will depend on the particular choice of the norm function, and therefore the theory of GMM considers an entire family of norms, defined as-
where W is a positive-definite weighting matrix, and m′ denotes transpositionTransposeIn linear algebra, the transpose of a matrix A is another matrix AT created by any one of the following equivalent actions:...
. In practice, the weighting matrix W is computed based on the available data set, which will be denoted as . Thus, the GMM estimator can be written as-
Under suitable conditions this estimator is consistentConsistent estimatorIn statistics, a sequence of estimators for parameter θ0 is said to be consistent if this sequence converges in probability to θ0...
, asymptotically normal, and with right choice of weighting matrix asymptotically efficient.
Consistency
ConsistencyConsistent estimatorIn statistics, a sequence of estimators for parameter θ0 is said to be consistent if this sequence converges in probability to θ0...
is a statistical property of an estimator stating that, having sufficient number of observations, the estimator will get arbitrarily close to the true value of parameter:
(see Convergence in probability).
Necessary and sufficient conditions for a GMM estimator to be consistent are as follows:-
where W is a positive semi-definite matrix,
-
only for
-
which is compact
Compact spaceIn mathematics, specifically general topology and metric topology, a compact space is an abstract mathematical space whose topology has the compactness property, which has many important implications not valid in general spaces...
, -
is continuous at each θ with probability one,
-
The second condition here (so-called Global identification condition) is often particularly hard to verify. There exist simpler necessary but not sufficient conditions, which may be used to detect non-identification problem:- Order condition. The dimension of moment function m(θ) should be at least as large as the dimension of parameter vector θ.
- Local identification. If g(Y,θ) is continuously differentiable in a neighborhood of
, then matrix
must have full column rank
Rank (linear algebra)The column rank of a matrix A is the maximum number of linearly independent column vectors of A. The row rank of a matrix A is the maximum number of linearly independent row vectors of A...
.
In practice applied econometricians often simply assume that global identification holds, without actually proving it.
Asymptotic normality
Asymptotic normality is a useful property, as it allows us to construct confidence bandsConfidence intervalIn statistics, a confidence interval is a particular kind of interval estimate of a population parameter and is used to indicate the reliability of an estimate. It is an observed interval , in principle different from sample to sample, that frequently includes the parameter of interest, if the...
for the estimator, and conduct different tests. Before we can make a statement about the asymptotic distribution of the GMM estimator, we need to define two auxiliary matrices:-
Then under conditions 1–6 listed below, the GMM estimator will be asymptotically normal with limiting distribution
(see Convergence in distribution).
Conditions:-
is consistent (see previous section),
-
lies in the interior of set
-
is continuously differentiable in some neighborhood N of
with probability one,
-
-
- matrix
is nonsingular.
Efficiency
So far we have said nothing about the choice of matrix W, except that it must be positive semi-definite. In fact any such matrix will produce a consistent and asymptotically normal GMM estimator, the only difference will be in the asymptotic variance of that estimator. It can be shown that taking
will result in the most efficient estimator in the class of all asymptotically normal estimators. Efficiency in this case means that such an estimator will have the smallest possible variance (we say that matrix A is smaller than matrix B if B–A is positive semi-definite).
In this case the formula for the asymptotic distribution of the GMM estimator simplifies to
The proof that such a choice of weighting matrix is indeed optimal is quite elegant, and is often adopted with slight modifications when establishing efficiency of other estimators. As a rule of thumb, a weighting matrix is optimal whenever it makes the “sandwich formula” for variance collapse into a simpler expression.
Proof. We will consider the difference between asymptotic variance with arbitrary W and asymptotic variance with . If we can factor this difference into a symmetric product of the form CC for some matrix C, then it will guarantee that this difference is nonnegative-definite, and thus
will be optimal by definition.
where we introduced matrices A and B in order to slightly simplify notation; I is an identity matrix Identity matrixIn linear algebra, the identity matrix or unit matrix of size n is the n×n square matrix with ones on the main diagonal and zeros elsewhere. It is denoted by In, or simply by I if the size is immaterial or can be trivially determined by the context...
. We can see that matrix B here is symmetric and idempotentProjection (linear algebra)In linear algebra and functional analysis, a projection is a linear transformation P from a vector space to itself such that P2 = P. It leaves its image unchanged....
:. This means I–B is symmetric and idempotent as well:
. Thus we can continue to factor the previous expression as
Implementation
One difficulty with implementing the outlined method is that we cannot take because, by the definition of matrix Ω, we need to know the value of θ0 in order to compute this matrix, and θ0 is precisely the quantity we don’t know and are trying to estimate in the first place.
Several approaches exist to deal with this issue, the first one being the most popular:
- Two-step feasible GMM:
- Step 1: Take W = I (the identity matrixIdentity matrixIn linear algebra, the identity matrix or unit matrix of size n is the n×n square matrix with ones on the main diagonal and zeros elsewhere. It is denoted by In, or simply by I if the size is immaterial or can be trivially determined by the context...
), and compute preliminary GMM estimate . This estimator is consistent for θ0, although not efficient.
- Step 2: Take
where we have plugged in our first-step preliminary estimate . This matrix converges in probability to Ω−1 and therefore if we compute with this weighting matrix, the estimator will be asymptotically efficient.
-
- Step 1: Take W = I (the identity matrix
- Iterated GMM. Essentially the same procedure as 2-step GMM, except that the matrix
is recalculated several times. That is, the estimate obtained in step 2 is used to calculate the weighting matrix for step 3, and so on. Such estimator, denoted , is equivalent to solving the following system of equations:
-
Asymptotically no improvement can be achieved through such iterations, although certain Monte-Carlo experiments suggest that finite-sample properties of this estimator are slightly better.
- Continuously Updating GMM (CUGMM, or CUE). Estimates simultaneously with estimating the weighting matrix W:
-
In Monte-Carlo experiments this method demonstrated a better performance than the traditional two-step GMM: the estimator has smaller median bias (although fatter tails), and the J-test for overidentifying restrictions in many cases was more reliable.
- Continuously Updating GMM (CUGMM, or CUE). Estimates simultaneously with estimating the weighting matrix W:
-
Another important issue in implementation of minimization procedure is that the function is supposed to search through (possibly high-dimensional) parameter space Θ and find the value of θ which minimizes the objective function. No generic recommendation for such procedure exists, it is a subject of its own field, numerical optimization.
J-test
When the number of moment conditions is greater than the dimension of the parameter vector θ, the model is said to be over-identified. Over-identification allows us to check whether the model's moment conditions match the data well or not.
Conceptually we can check whetheris sufficiently close to zero to suggest that the model fits the data well. The GMM method has then replaced the problem of solving the equation
, which chooses
to match the restrictions exactly, by a minimization calculation. The minimization can always be conducted even when no
exists such that
. This is what J-test does. The J-test is also called a test for over-identifying restrictions.
Formally we consider two hypothesesStatistical hypothesis testingA statistical hypothesis test is a method of making decisions using data, whether from a controlled experiment or an observational study . In statistics, a result is called statistically significant if it is unlikely to have occurred by chance alone, according to a pre-determined threshold...
:-
(the null hypothesis
Null hypothesisThe practice of science involves formulating and testing hypotheses, assertions that are capable of being proven false using a test of observed data. The null hypothesis typically corresponds to a general or default position...
that the model is “valid”), and -
(the alternative hypothesis that model is “invalid”; the data do not come close to meeting the restrictions)
Under hypothesis, the following so-called J-statistic is asymptotically chi-squared with k–l degrees of freedom. Define J to be:
under
whereis the GMM estimator of the parameter
, k is the number of moment conditions (dimension of vector g), and l is the number of estimated parameters (dimension of vector θ). Matrix
must converge in probability to
, the efficient weighting matrix (note that previously we only required that W be proportional to
for estimator to be efficient; however in order to conduct the J-test W must be exactly equal to
, not simply proportional).
Under the alternative hypothesis, the J-statistic is asymptotically unbounded:
-
under
To conduct the test we compute the value of J from the data. It is a nonnegative number. We compare it with (say) the 0.95 quantileQuantileQuantiles are points taken at regular intervals from the cumulative distribution function of a random variable. Dividing ordered data into q essentially equal-sized data subsets is the motivation for q-quantiles; the quantiles are the data values marking the boundaries between consecutive subsets...
of the
distribution:
-
is rejected at 95% confidence level if
-
cannot be rejected at 95% confidence level if
Scope
Many other popular estimation techniques can be cast in terms of GMM optimization:
- Ordinary Least Squares (OLS) is equivalent to GMM with moment conditions:
-
- Generalized Least SquaresGeneralized least squaresIn statistics, generalized least squares is a technique for estimating the unknown parameters in a linear regression model. The GLS is applied when the variances of the observations are unequal , or when there is a certain degree of correlation between the observations...
(GLS)
-
- Instrumental variableInstrumental variableIn statistics, econometrics, epidemiology and related disciplines, the method of instrumental variables is used to estimate causal relationships when controlled experiments are not feasible....
s regression (IV)
-
- Non-linear Least SquaresNon-linear least squaresNon-linear least squares is the form of least squares analysis which is used to fit a set of m observations with a model that is non-linear in n unknown parameters . It is used in some forms of non-linear regression. The basis of the method is to approximate the model by a linear one and to...
(NLLS):
-
- Maximum likelihoodMaximum likelihoodIn statistics, maximum-likelihood estimation is a method of estimating the parameters of a statistical model. When applied to a data set and given a statistical model, maximum-likelihood estimation provides estimates for the model's parameters....
estimation (MLE):
-
Implementations
-
-
-
-
-