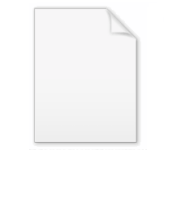
Response surface methodology
Encyclopedia
In statistics, response surface methodology (RSM) explores the relationships between several explanatory variables and one or more response variables. The method was introduced by G. E. P. Box and K. B. Wilson in 1951. The main idea of RSM is to use a sequence of designed experiments
to obtain an optimal response. Box and Wilson suggest using a second-degree
polynomial
model to do this. They acknowledge that this model is only an approximation, but use it because such a model is easy to estimate and apply, even when little is known about the process.
or a fractional factorial designs. This is sufficient to determine which explanatory variables have an impact on the response variable(s) of interest. Once it is suspected that only significant explanatory variables are left, then a more complicated design, such as a central composite design
can be implemented to estimate a second-degree polynomial model, which is still only an approximation at best. However, the second-degree model can be used to optimize (maximize, minimize, or attain a specific target for) a response.
, and in the response-surface methodology textbooks of Box and Draper and of Atkinson, Donev and Tobias. An extensive discussion and survey appears in the advanced textbook by John Cornell.
Nonetheless, response surface methodology has an effective track-record of helping researchers improve products and services: For example, Box's original response-surface modeling enabled chemical engineers to improve a process that had been stuck at a saddle-point for years. The engineers had not been able to afford to fit a cubic three-level design to estimate a quadratic model, and their biased
linear-models estimated the gradient to be zero. Box's design reduced the costs of experimentation so that a quadratic model could be fit, which led to a (long-sought) ascent direction.
Application of response surface methodology can also be incorporated in matters of smaller proportions, such as video games. This is especially prevalent in golf games, where the use of response surface methodology can be applied to help the player achieve greater accomplishments than before.
Design of experiments
In general usage, design of experiments or experimental design is the design of any information-gathering exercises where variation is present, whether under the full control of the experimenter or not. However, in statistics, these terms are usually used for controlled experiments...
to obtain an optimal response. Box and Wilson suggest using a second-degree
Degree of a polynomial
The degree of a polynomial represents the highest degree of a polynominal's terms , should the polynomial be expressed in canonical form . The degree of an individual term is the sum of the exponents acting on the term's variables...
polynomial
Polynomial
In mathematics, a polynomial is an expression of finite length constructed from variables and constants, using only the operations of addition, subtraction, multiplication, and non-negative integer exponents...
model to do this. They acknowledge that this model is only an approximation, but use it because such a model is easy to estimate and apply, even when little is known about the process.
Basic approach of response surface methodology
An easy way to estimate a first-degree polynomial model is to use a factorial experimentFactorial experiment
In statistics, a full factorial experiment is an experiment whose design consists of two or more factors, each with discrete possible values or "levels", and whose experimental units take on all possible combinations of these levels across all such factors. A full factorial design may also be...
or a fractional factorial designs. This is sufficient to determine which explanatory variables have an impact on the response variable(s) of interest. Once it is suspected that only significant explanatory variables are left, then a more complicated design, such as a central composite design
Central composite design
In statistics, a central composite design is an experimental design, useful in response surface methodology, for building a second order model for the response variable without needing to use a complete three-level factorial experiment....
can be implemented to estimate a second-degree polynomial model, which is still only an approximation at best. However, the second-degree model can be used to optimize (maximize, minimize, or attain a specific target for) a response.
Cube
Cubic designs are discussed by Kiefer, by Atkinson, Donev, and Tobias and by Hardin and Sloane.Simplex geometry and mixture experiments
Mixture experiments are discussed in many books on the design of experimentsDesign of experiments
In general usage, design of experiments or experimental design is the design of any information-gathering exercises where variation is present, whether under the full control of the experimenter or not. However, in statistics, these terms are usually used for controlled experiments...
, and in the response-surface methodology textbooks of Box and Draper and of Atkinson, Donev and Tobias. An extensive discussion and survey appears in the advanced textbook by John Cornell.
Multiple objective functions
Some extensions of response surface methodology deal with the multiple response problem. Multiple response variables create difficulty because what is optimal for one response may not be optimal for other responses. Other extensions are used to reduce variability in a single response while targeting a specific value, or attaining a near maximum or minimum while preventing variability in that response from getting too large.Practical concerns
Response surface methodology uses statistical models, and therefore practitioners need to be aware that even the best statistical model is an approximation to reality. In practice, both the models and the parameter values are unknown, and subject to uncertainty on top of ignorance. Of course, an estimated optimum point need not be optimum in reality, because of the errors of the estimates and of the inadequacies of the model.Nonetheless, response surface methodology has an effective track-record of helping researchers improve products and services: For example, Box's original response-surface modeling enabled chemical engineers to improve a process that had been stuck at a saddle-point for years. The engineers had not been able to afford to fit a cubic three-level design to estimate a quadratic model, and their biased
Bias (statistics)
A statistic is biased if it is calculated in such a way that it is systematically different from the population parameter of interest. The following lists some types of, or aspects of, bias which should not be considered mutually exclusive:...
linear-models estimated the gradient to be zero. Box's design reduced the costs of experimentation so that a quadratic model could be fit, which led to a (long-sought) ascent direction.
Application of response surface methodology can also be incorporated in matters of smaller proportions, such as video games. This is especially prevalent in golf games, where the use of response surface methodology can be applied to help the player achieve greater accomplishments than before.
See also
- Plackett-Burman designPlackett-Burman designPlackett–Burman designs are experimental designs presented in 1946 by Robin L. Plackett and J. P. Burman while working in the British Ministry of Supply....
- Box-Behnken designBox-Behnken designIn statistics, Box–Behnken designs are experimental designs for response surface methodology, devised by George E. P. Box and Donald Behnken in 1960, to achieve the following goals:...
- IOSOIOSOIOSO is a multiobjective, multidimensional nonlinear optimization technology.-IOSO approach:IOSO Technology is based on the response surface methodology approach....
method based on response-surface methodology - Optimal designOptimal designOptimal designs are a class of experimental designs that are optimal with respect to some statistical criterion.In the design of experiments for estimating statistical models, optimal designs allow parameters to be estimated without bias and with minimum-variance...
s - Polynomial regressionPolynomial regressionIn statistics, polynomial regression is a form of linear regression in which the relationship between the independent variable x and the dependent variable y is modeled as an nth order polynomial...
- Polynomial and rational function modelingPolynomial and rational function modelingIn statistical modeling , polynomial functions and rational functions are sometimes used as an empirical technique for curve fitting.-Polynomial function models:A polynomial function is one that has the form...
- Surrogate modelSurrogate modelMost engineering design problems require experiments and/or simulations to evaluate design objective and constraint functions as function of design variables. For example, in order to find the optimal airfoil shape for an aircraft wing, an engineer simulates the air flow around the wing for...
- Probabilistic designProbabilistic designProbabilistic design is a discipline within engineering design. It deals primarily with the consideration of the effects of random variability upon the performance of an engineering system during the design phase. Typically, these effects are related to quality and reliability...
Historical
(Appendix No. 14). NOAA PDF Eprint. Reprinted in paragraphs 139–157, and in Abstract at JSTOR.External links
- Response surface designs
- Matlab SUrrogate MOdeling Toolbox - SUMO Toolbox - Matlab code for Response Surface Modeling