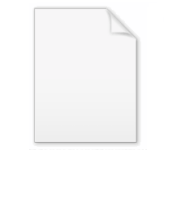
Quasi-maximum likelihood
Encyclopedia
A quasi-maximum likelihood estimate (QMLE, also known as a "pseudo-likelihood estimate" or a "composite likelihood estimate") is an estimate of a parameter
θ in a statistical model
that is formed by maximizing a function that is related to the logarithm of the likelihood function
, but is not equal to it. In contrast, the maximum likelihood
estimate maximizes the actual log likelihood function for the data and model. The function that is maximized to form a QMLE is often a simplified form of the actual log likelihood function. A common way to form such a simplified function is to use the log-likelihood function of a misspecified model that treats certain data values as being independent, even when in actuality they may not be. This removes any parameters from the model that are used to characterize these dependencies. Doing this only makes sense if the dependency structure is a nuisance parameter with respect to the goals of the analysis.
As long as the quasi-likelihood function that is maximized is not overly simplified, the QMLE (or composite likelihood estimate) is consistent
and asymptotically normal. It is less efficient
than the maximum likelihood estimate, but may only be slightly less efficient if the quasi-likelihood is constructed so as to minimize the loss of information relative to the actual likelihood. Standard approaches to statistical inference that are used with maximum likelihood estimates, such as the formation of confidence intervals, and statistics for model comparison, can be generalized to the quasi-maximum likelihood setting.
Parameter
Parameter from Ancient Greek παρά also “para” meaning “beside, subsidiary” and μέτρον also “metron” meaning “measure”, can be interpreted in mathematics, logic, linguistics, environmental science and other disciplines....
θ in a statistical model
Statistical model
A statistical model is a formalization of relationships between variables in the form of mathematical equations. A statistical model describes how one or more random variables are related to one or more random variables. The model is statistical as the variables are not deterministically but...
that is formed by maximizing a function that is related to the logarithm of the likelihood function
Likelihood function
In statistics, a likelihood function is a function of the parameters of a statistical model, defined as follows: the likelihood of a set of parameter values given some observed outcomes is equal to the probability of those observed outcomes given those parameter values...
, but is not equal to it. In contrast, the maximum likelihood
Maximum likelihood
In statistics, maximum-likelihood estimation is a method of estimating the parameters of a statistical model. When applied to a data set and given a statistical model, maximum-likelihood estimation provides estimates for the model's parameters....
estimate maximizes the actual log likelihood function for the data and model. The function that is maximized to form a QMLE is often a simplified form of the actual log likelihood function. A common way to form such a simplified function is to use the log-likelihood function of a misspecified model that treats certain data values as being independent, even when in actuality they may not be. This removes any parameters from the model that are used to characterize these dependencies. Doing this only makes sense if the dependency structure is a nuisance parameter with respect to the goals of the analysis.
As long as the quasi-likelihood function that is maximized is not overly simplified, the QMLE (or composite likelihood estimate) is consistent
Consistent estimator
In statistics, a sequence of estimators for parameter θ0 is said to be consistent if this sequence converges in probability to θ0...
and asymptotically normal. It is less efficient
Efficiency (statistics)
In statistics, an efficient estimator is an estimator that estimates the quantity of interest in some “best possible” manner. The notion of “best possible” relies upon the choice of a particular loss function — the function which quantifies the relative degree of undesirability of estimation errors...
than the maximum likelihood estimate, but may only be slightly less efficient if the quasi-likelihood is constructed so as to minimize the loss of information relative to the actual likelihood. Standard approaches to statistical inference that are used with maximum likelihood estimates, such as the formation of confidence intervals, and statistics for model comparison, can be generalized to the quasi-maximum likelihood setting.