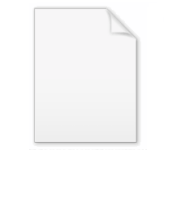
Normality test
Encyclopedia
In statistics
, normality tests are used to determine whether a data set
is well-modeled by a normal distribution or not, or to compute how likely an underlying random variable
is to be normally distributed.
More precisely, they are a form of model selection
, and can be interpreted several ways, depending on one's interpretations of probability:
of the sample data to a normal probability curve. The empirical distribution of the data (the histogram) should be bell-shaped and resemble the normal distribution. This might be difficult to see if the sample is small. In this case one might proceed by regressing the data against the quantile
s of a normal distribution with the same mean and variance as the sample. Lack of fit to the regression line suggests a departure from normality.
A graphical tool for assessing normality is the normal probability plot
, a quantile-quantile plot (QQ plot) of the standardized data against the standard normal distribution. Here the correlation
between the sample data and normal quantiles (a measure of the goodness of fit) measures how well the data is modeled by a normal distribution. For normal data the points plotted in the QQ plot should fall approximately on a straight line, indicating high positive correlation. These plots are easy to interpret and also have the benefit that outliers are easily identified.
and computes their z-score, or more properly t-statistic (number of sample standard deviations that a sample is above or below the sample mean), and compares it to the 68–95–99.7 rule:
if one has a 3σ event (properly, a 3s event) and significantly fewer than 300 samples, or a 4s event and significantly fewer than 15,000 samples, then a normal distribution significantly understates the maximum magnitude of deviations in the sample data.
This test is useful in cases where one faces kurtosis risk
– where large deviations matter – and has the benefits that it is very easy to compute and to communicate: non-statisticians can easily grasp that "6σ events don’t happen in normal distributions".
, the Jarque–Bera test
, the Anderson–Darling test, the Cramér–von Mises criterion, the Lilliefors test for normality (itself an adaptation of the Kolmogorov–Smirnov test), the Shapiro–Wilk test, the Pearson's chi-squared test
, and the Shapiro–Francia test. Some published works recommend the Jarque–Bera test.
Historically, the third and fourth standardized moments (skewness
and kurtosis
) were some of the earliest tests for normality. Mardia's multivariate skewness and kurtosis tests generalize the moment tests to the multivariate case. Other early test statistic
s include the ratio of the mean absolute deviation to the standard deviation and of the range to the standard deviation.
More recent tests of normality include the energy test (Szekely and Rizzo) and the tests based on the empirical characteristic function (ecf) (e.g. Epps and Pulley, Henze–Zirkler, BHEP tests). The energy and the ecf tests are powerful tests that apply for testing univariate or multivariate normality and are statistically consistent against general alternatives.
Spiegelhalter suggests using Bayes factors to compare normality with a different class of distributional alternatives. This approach has been extended by Farrell and Rogers-Stewart.
from a linear regression
model. If they are not normally distributed, the residuals should not be used in Z tests or in any other tests derived from the normal distribution, such as t tests, F tests and chi-squared tests. If the residuals are not normally distributed, then the dependent variable or at least one explanatory variable may have the wrong functional form, or important variables may be missing, etc. Correcting one or more of these systematic errors may produce residuals that are normally distributed.
Statistics
Statistics is the study of the collection, organization, analysis, and interpretation of data. It deals with all aspects of this, including the planning of data collection in terms of the design of surveys and experiments....
, normality tests are used to determine whether a data set
Data set
A data set is a collection of data, usually presented in tabular form. Each column represents a particular variable. Each row corresponds to a given member of the data set in question. Its values for each of the variables, such as height and weight of an object or values of random numbers. Each...
is well-modeled by a normal distribution or not, or to compute how likely an underlying random variable
Random variable
In probability and statistics, a random variable or stochastic variable is, roughly speaking, a variable whose value results from a measurement on some type of random process. Formally, it is a function from a probability space, typically to the real numbers, which is measurable functionmeasurable...
is to be normally distributed.
More precisely, they are a form of model selection
Model selection
Model selection is the task of selecting a statistical model from a set of candidate models, given data. In the simplest cases, a pre-existing set of data is considered...
, and can be interpreted several ways, depending on one's interpretations of probability:
- In descriptive statisticsDescriptive statisticsDescriptive statistics quantitatively describe the main features of a collection of data. Descriptive statistics are distinguished from inferential statistics , in that descriptive statistics aim to summarize a data set, rather than use the data to learn about the population that the data are...
terms, one measures a goodness of fit of a normal model to the data – if the fit is poor then the data is not well modeled in that respect by a normal distribution, without making a judgment on any underlying variable. - In frequentist statistics statistical hypothesis testingStatistical hypothesis testingA statistical hypothesis test is a method of making decisions using data, whether from a controlled experiment or an observational study . In statistics, a result is called statistically significant if it is unlikely to have occurred by chance alone, according to a pre-determined threshold...
, data are tested against the null hypothesisNull hypothesisThe practice of science involves formulating and testing hypotheses, assertions that are capable of being proven false using a test of observed data. The null hypothesis typically corresponds to a general or default position...
that it is normally distributed. - In Bayesian statisticsBayesian statisticsBayesian statistics is that subset of the entire field of statistics in which the evidence about the true state of the world is expressed in terms of degrees of belief or, more specifically, Bayesian probabilities...
, one does not "test normality" per se, but rather computes the likelihood that the data comes from a normal distribution with given parameters μ,σ (for all μ,σ), and compares that with the likelihood that the data comes from other distributions under consideration, most simply using Bayes factors (giving the relatively likelihood of seeing the data given different models), or more finely taking a prior distribution on possible models and parameters and computing a posterior distribution given the computed likelihoods.
Graphical methods
An informal approach to testing normality is to compare a histogramHistogram
In statistics, a histogram is a graphical representation showing a visual impression of the distribution of data. It is an estimate of the probability distribution of a continuous variable and was first introduced by Karl Pearson...
of the sample data to a normal probability curve. The empirical distribution of the data (the histogram) should be bell-shaped and resemble the normal distribution. This might be difficult to see if the sample is small. In this case one might proceed by regressing the data against the quantile
Quantile
Quantiles are points taken at regular intervals from the cumulative distribution function of a random variable. Dividing ordered data into q essentially equal-sized data subsets is the motivation for q-quantiles; the quantiles are the data values marking the boundaries between consecutive subsets...
s of a normal distribution with the same mean and variance as the sample. Lack of fit to the regression line suggests a departure from normality.
A graphical tool for assessing normality is the normal probability plot
Normal probability plot
The normal probability plot is a graphical technique for normality testing: assessing whether or not a data set is approximately normally distributed....
, a quantile-quantile plot (QQ plot) of the standardized data against the standard normal distribution. Here the correlation
Pearson product-moment correlation coefficient
In statistics, the Pearson product-moment correlation coefficient is a measure of the correlation between two variables X and Y, giving a value between +1 and −1 inclusive...
between the sample data and normal quantiles (a measure of the goodness of fit) measures how well the data is modeled by a normal distribution. For normal data the points plotted in the QQ plot should fall approximately on a straight line, indicating high positive correlation. These plots are easy to interpret and also have the benefit that outliers are easily identified.
Back-of-the-envelope test
A simple back-of-the-envelope test takes the sample maximum and minimumSample maximum and minimum
In statistics, the maximum and sample minimum, also called the largest observation, and smallest observation, are the values of the greatest and least elements of a sample....
and computes their z-score, or more properly t-statistic (number of sample standard deviations that a sample is above or below the sample mean), and compares it to the 68–95–99.7 rule:
if one has a 3σ event (properly, a 3s event) and significantly fewer than 300 samples, or a 4s event and significantly fewer than 15,000 samples, then a normal distribution significantly understates the maximum magnitude of deviations in the sample data.
This test is useful in cases where one faces kurtosis risk
Kurtosis risk
Kurtosis risk in statistics and decision theory denotes the fact that observations are spread in a wider fashion than the normal distribution entails...
– where large deviations matter – and has the benefits that it is very easy to compute and to communicate: non-statisticians can easily grasp that "6σ events don’t happen in normal distributions".
Frequentist tests
Tests of univariate normality include D'Agostino's K-squared testD'Agostino's K-squared test
In statistics, D’Agostino’s K2 test is a goodness-of-fit measure of departure from normality, that is the test aims to establish whether or not the given sample comes from a normally distributed population...
, the Jarque–Bera test
Jarque–Bera test
In statistics, the Jarque–Bera test is a goodness-of-fit test of whether sample data have the skewness and kurtosis matching a normal distribution. The test is named after Carlos Jarque and Anil K. Bera...
, the Anderson–Darling test, the Cramér–von Mises criterion, the Lilliefors test for normality (itself an adaptation of the Kolmogorov–Smirnov test), the Shapiro–Wilk test, the Pearson's chi-squared test
Pearson's chi-squared test
Pearson's chi-squared test is the best-known of several chi-squared tests – statistical procedures whose results are evaluated by reference to the chi-squared distribution. Its properties were first investigated by Karl Pearson in 1900...
, and the Shapiro–Francia test. Some published works recommend the Jarque–Bera test.
Historically, the third and fourth standardized moments (skewness
Skewness
In probability theory and statistics, skewness is a measure of the asymmetry of the probability distribution of a real-valued random variable. The skewness value can be positive or negative, or even undefined...
and kurtosis
Kurtosis
In probability theory and statistics, kurtosis is any measure of the "peakedness" of the probability distribution of a real-valued random variable...
) were some of the earliest tests for normality. Mardia's multivariate skewness and kurtosis tests generalize the moment tests to the multivariate case. Other early test statistic
Test statistic
In statistical hypothesis testing, a hypothesis test is typically specified in terms of a test statistic, which is a function of the sample; it is considered as a numerical summary of a set of data that...
s include the ratio of the mean absolute deviation to the standard deviation and of the range to the standard deviation.
More recent tests of normality include the energy test (Szekely and Rizzo) and the tests based on the empirical characteristic function (ecf) (e.g. Epps and Pulley, Henze–Zirkler, BHEP tests). The energy and the ecf tests are powerful tests that apply for testing univariate or multivariate normality and are statistically consistent against general alternatives.
Bayesian tests
Kullback–Leibler distances between the whole posterior distributions of the slope and variance do not indicate non-normality. However, the ratio of expectations of these posteriors and the expectation of the ratios give similar results to the Shapiro–Wilk statistic except for very small samples, when non-informative priors are used.Spiegelhalter suggests using Bayes factors to compare normality with a different class of distributional alternatives. This approach has been extended by Farrell and Rogers-Stewart.
Applications
One application of normality tests is to the residualsErrors and residuals in statistics
In statistics and optimization, statistical errors and residuals are two closely related and easily confused measures of the deviation of a sample from its "theoretical value"...
from a linear regression
Linear regression
In statistics, linear regression is an approach to modeling the relationship between a scalar variable y and one or more explanatory variables denoted X. The case of one explanatory variable is called simple regression...
model. If they are not normally distributed, the residuals should not be used in Z tests or in any other tests derived from the normal distribution, such as t tests, F tests and chi-squared tests. If the residuals are not normally distributed, then the dependent variable or at least one explanatory variable may have the wrong functional form, or important variables may be missing, etc. Correcting one or more of these systematic errors may produce residuals that are normally distributed.