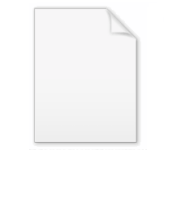
Geometric hashing
Encyclopedia
In computer science
, geometric hashing is originally a method for efficiently finding two-dimensional objects represented by discrete points that have undergone an affine transformation
, though extensions exist to some other object representations and transformations. In an off-line step, the objects are encoded by treating each pairs of points as a geometric basis
. The remaining points can be represented in an invariant
fashion with respect to this basis using two parameters. For each point, its quantized
transformed coordinates are stored in the hash table
as a key, and indices of the basis points as a value. Then a new pair of basis points is selected, and the process is repeated. In the on-line (recognition) step, randomly selected pairs of data points are considered as candidate bases. For each candidate basis, the remaining data points are encoded according to the basis and possible correspondences from the object are found in the previously constructed table. The candidate basis is accepted if a sufficiently large number of the data points index a consistent object basis.
Geometric hashing was originally suggested in computer vision
for object recognition
in 2D and 3D, but later was applied to different problems such as structural alignment
of protein
s.
such as SIFT
could be used for indexing).
Hash Table:
Computer science
Computer science or computing science is the study of the theoretical foundations of information and computation and of practical techniques for their implementation and application in computer systems...
, geometric hashing is originally a method for efficiently finding two-dimensional objects represented by discrete points that have undergone an affine transformation
Affine transformation
In geometry, an affine transformation or affine map or an affinity is a transformation which preserves straight lines. It is the most general class of transformations with this property...
, though extensions exist to some other object representations and transformations. In an off-line step, the objects are encoded by treating each pairs of points as a geometric basis
Basis (linear algebra)
In linear algebra, a basis is a set of linearly independent vectors that, in a linear combination, can represent every vector in a given vector space or free module, or, more simply put, which define a "coordinate system"...
. The remaining points can be represented in an invariant
Invariant (mathematics)
In mathematics, an invariant is a property of a class of mathematical objects that remains unchanged when transformations of a certain type are applied to the objects. The particular class of objects and type of transformations are usually indicated by the context in which the term is used...
fashion with respect to this basis using two parameters. For each point, its quantized
Quantization (signal processing)
Quantization, in mathematics and digital signal processing, is the process of mapping a large set of input values to a smaller set – such as rounding values to some unit of precision. A device or algorithmic function that performs quantization is called a quantizer. The error introduced by...
transformed coordinates are stored in the hash table
Hash table
In computer science, a hash table or hash map is a data structure that uses a hash function to map identifying values, known as keys , to their associated values . Thus, a hash table implements an associative array...
as a key, and indices of the basis points as a value. Then a new pair of basis points is selected, and the process is repeated. In the on-line (recognition) step, randomly selected pairs of data points are considered as candidate bases. For each candidate basis, the remaining data points are encoded according to the basis and possible correspondences from the object are found in the previously constructed table. The candidate basis is accepted if a sufficiently large number of the data points index a consistent object basis.
Geometric hashing was originally suggested in computer vision
Computer vision
Computer vision is a field that includes methods for acquiring, processing, analysing, and understanding images and, in general, high-dimensional data from the real world in order to produce numerical or symbolic information, e.g., in the forms of decisions...
for object recognition
Object recognition
Object recognition in computer vision is the task of finding a given object in an image or video sequence. Humans recognize a multitude of objects in images with little effort, despite the fact that the image of the objects may vary somewhat in different view points, in many different sizes / scale...
in 2D and 3D, but later was applied to different problems such as structural alignment
Structural alignment
Structural alignment attempts to establish homology between two or more polymer structures based on their shape and three-dimensional conformation. This process is usually applied to protein tertiary structures but can also be used for large RNA molecules...
of protein
Protein
Proteins are biochemical compounds consisting of one or more polypeptides typically folded into a globular or fibrous form, facilitating a biological function. A polypeptide is a single linear polymer chain of amino acids bonded together by peptide bonds between the carboxyl and amino groups of...
s.
Geometric Hashing in Computer Vision
Geometric Hashing is a method used for object recognition. Let’s say that we want to check if a model image can be seen in an input image. This can be accomplished with geometric hashing. The method could be used to recognize one of the multiple objects in a base, in this case the hash table should store not only the pose information but also the index of object model in the base.Example
For simplicity, this example will not use too many point features and assume that their descriptors are given by their coordinates only (in practice local descriptorsVisual descriptors
In computer vision, visual descriptors or image descriptors are descriptions of the visual features of the contents in images, videos, algorithms, or applications that produce such descriptions...
such as SIFT
Scale-invariant feature transform
Scale-invariant feature transform is an algorithm in computer vision to detect and describe local features in images. The algorithm was published by David Lowe in 1999....
could be used for indexing).
Training Phase
- Find the model's feature points. Assume that 5 feature points are found in the model image with the coordinates
, see the picture.
- Introduce a basis to describe the locations of the feature points. For 2D space and affine transform the basis is defined by a pair of points. The point of origin is placed in the middle of the segment connecting the two points (P2, P4 in our example), the
axis is directed towards one of them, the
is orthogonal and goes through the origin. The scale is selected such that absolute value of
for both basis points is 1.
- Describe feature locations with respect to that basis, i.e. compute the projections to the new coordinate axes. The coordinates should be discretised to make recognition robustRobust decisionRobust decision is a term dating back to the late 1990s. It is used to identify decisions made with a process that includes formal consideration of uncertainty...
to noise, we take the bin size 0.25. We thus get the coordinates - Store the basis in a hash tableHash tableIn computer science, a hash table or hash map is a data structure that uses a hash function to map identifying values, known as keys , to their associated values . Thus, a hash table implements an associative array...
indexed by the features (only transformed coordinates in this case). If there were more objects to match with, we should also store the object number along with the basis pair. - Repeat the process for a different basis pair (Step 2). It is needed to handle occlusions. Ideally, all the non-colinear pairs should be enumerated. We provide the hash table after two iterations, the pair (P1, P3) is selected for the second one.
Hash Table:
Vector (![]() ![]() |
basis |
---|---|
![]() |
(P2,P4) |
![]() |
(P2,P4) |
![]() |
(P2,P4) |
![]() |
(P2,P4) |
![]() |
(P2,P4) |
![]() |
(P1,P3) |
![]() |
(P1,P3) |
![]() |
(P1,P3) |
![]() |
(P1,P3) |
![]() |
(P1,P3) |
Recognition Phase
- Find interesting feature points in the input image.
- Choose an arbitrary basis. If there isn't a suitable arbitrary basis, then it is likely that the input image does not contain the target object.
- Describe coordinates of the feature points in the new basis. Quantize obtained coordinates as it was done before.
- Compare all the transformed point features in the input image with the hash table. If the point features are identical or similar, then increase the count for the corresponding basis (and the type of object, if any).
- For each basis such that the count exceeds a certain threshold, verify the hypothesis that it corresponds to an image basis chosen in Step 2. Transfer the image coordinate system to the model one (for the supposed object) and try to match them. If succeed, the object is found. Otherwise, go back to Step 2.
Finding mirrored pattern
It seems that this method is only capable of handling scaling, translation, and rotation. However, the input Image may contain the object in mirror transform. Therefore, geometric hashing should be able to find the object, too. In fact, there are two ways to detect mirrored objects.- For the vector graph, make the left side as positive, and the right side as negative. Or multiplying the x position by -1 will give the same result.
- Use 3 points for the basis. This allows detecting mirror images (or objects). Actually, using 3 points for the basis is another approach for geometric hashing.