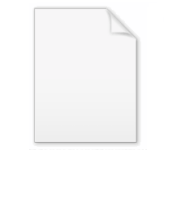
Evolution strategy
Encyclopedia
In computer science, evolution strategy (ES) is an optimization
technique based on ideas of adaptation and evolution. It belongs to the general class of evolutionary computation
or artificial evolution methodologies.
, Hans-Paul Schwefel
and his co-workers.
, as search operators. In common with evolutionary algorithms, the operators are applied in a loop. An iteration of the loop is called a generation. The sequence of generations is continued until a termination criterion is met.
As far as real-valued search spaces are concerned, mutation is normally performed by adding a normally distributed random value to each vector component. The step size or mutation strength (i.e. the standard deviation of the normal distribution) is often governed by self-adaptation (see evolution window
). Individual step sizes for each coordinate or correlations between coordinates are either governed by self-adaptation or by covariance matrix adaptation (CMA-ES
).
The (environmental) selection in evolution strategies is deterministic and only based on the fitness rankings, not on the actual fitness values. The resulting algorithm is therefore invariant with respect to monotonic transformations of the objective function. The simplest evolution strategy operates on a population of size two: the current point (parent) and the result of its mutation. Only if the mutant's fitness is at least as good as the parent one, it becomes the parent of the next generation. Otherwise the mutant is disregarded. This is a (1 + 1)-ES. More generally, λ mutants can be generated and compete with the parent, called (1 + λ)-ES. In (1 , λ)-ES the best mutant becomes the parent of the next generation while the current parent is always disregarded. For some of these variants, proofs of linear convergence
(in a stochastic
sense) have been derived on unimodal objective functions.
Contemporary derivatives of evolution strategy often use a population of μ parents and also recombination as an additional operator, called (μ/ρ+, λ)-ES. This makes them less prone to get stuck in local optima.
Optimization (mathematics)
In mathematics, computational science, or management science, mathematical optimization refers to the selection of a best element from some set of available alternatives....
technique based on ideas of adaptation and evolution. It belongs to the general class of evolutionary computation
Evolutionary computation
In computer science, evolutionary computation is a subfield of artificial intelligence that involves combinatorial optimization problems....
or artificial evolution methodologies.
History
The evolution strategy optimization technique was created in the early 1960s and developed further in the 1970s and later by Ingo RechenbergIngo Rechenberg
Ingo Rechenberg is a German computer scientist and professor. Rechenberg is a pioneer of the fields of evolutionary computation and artificial evolution. In the 1960s and 1970s he invented a highly influential set of optimization methods known as evolution strategies...
, Hans-Paul Schwefel
Hans-Paul Schwefel
Hans-Paul Schwefel is a German computer scientist and professor emeritus at University of Dortmund , where he held the chair of systems analysis from 1985 until 2006. He is one of the pioneers in evolutionary computation and one of the authors responsible for the evolution strategies...
and his co-workers.
Methods
Evolution strategies use natural problem-dependent representations, and primarily mutation and selectionSelection
In the context of evolution, certain traits or alleles of genes segregating within a population may be subject to selection. Under selection, individuals with advantageous or "adaptive" traits tend to be more successful than their peers reproductively—meaning they contribute more offspring to the...
, as search operators. In common with evolutionary algorithms, the operators are applied in a loop. An iteration of the loop is called a generation. The sequence of generations is continued until a termination criterion is met.
As far as real-valued search spaces are concerned, mutation is normally performed by adding a normally distributed random value to each vector component. The step size or mutation strength (i.e. the standard deviation of the normal distribution) is often governed by self-adaptation (see evolution window
Evolution window
It was observed in evolution strategies that significant progress toward the fitness/objective function's optimum, generally, can only happen in a narrow band of the mutation step size σ...
). Individual step sizes for each coordinate or correlations between coordinates are either governed by self-adaptation or by covariance matrix adaptation (CMA-ES
CMA-ES
CMA-ES stands for Covariance Matrix Adaptation Evolution Strategy. Evolution strategies are stochastic, derivative-free methods for numerical optimization of non-linear or non-convex continuous optimization problems. They belong to the class of evolutionary algorithms and evolutionary computation...
).
The (environmental) selection in evolution strategies is deterministic and only based on the fitness rankings, not on the actual fitness values. The resulting algorithm is therefore invariant with respect to monotonic transformations of the objective function. The simplest evolution strategy operates on a population of size two: the current point (parent) and the result of its mutation. Only if the mutant's fitness is at least as good as the parent one, it becomes the parent of the next generation. Otherwise the mutant is disregarded. This is a (1 + 1)-ES. More generally, λ mutants can be generated and compete with the parent, called (1 + λ)-ES. In (1 , λ)-ES the best mutant becomes the parent of the next generation while the current parent is always disregarded. For some of these variants, proofs of linear convergence
Rate of convergence
In numerical analysis, the speed at which a convergent sequence approaches its limit is called the rate of convergence. Although strictly speaking, a limit does not give information about any finite first part of the sequence, this concept is of practical importance if we deal with a sequence of...
(in a stochastic
Stochastic
Stochastic refers to systems whose behaviour is intrinsically non-deterministic. A stochastic process is one whose behavior is non-deterministic, in that a system's subsequent state is determined both by the process's predictable actions and by a random element. However, according to M. Kac and E...
sense) have been derived on unimodal objective functions.
Contemporary derivatives of evolution strategy often use a population of μ parents and also recombination as an additional operator, called (μ/ρ+, λ)-ES. This makes them less prone to get stuck in local optima.
See also
- Genetic algorithmGenetic algorithmA genetic algorithm is a search heuristic that mimics the process of natural evolution. This heuristic is routinely used to generate useful solutions to optimization and search problems...
- Evolutionary computationEvolutionary computationIn computer science, evolutionary computation is a subfield of artificial intelligence that involves combinatorial optimization problems....
- Covariance matrix adaptation evolution strategy (CMA-ES)CMA-ESCMA-ES stands for Covariance Matrix Adaptation Evolution Strategy. Evolution strategies are stochastic, derivative-free methods for numerical optimization of non-linear or non-convex continuous optimization problems. They belong to the class of evolutionary algorithms and evolutionary computation...
- Natural evolution strategyNatural evolution strategyNatural evolution strategies are a family of numerical optimization algorithms for black-box problems. Similar in spirit to evolution strategies, they iteratively update the parameters of a search distribution by following the natural gradient towards higher expected fitness.-Method:The general...
Research centers
- Bionics & Evolutiontechnique at the Technical University Berlin
- Chair of Algorithm Engineering (Ls11) – University of Dortmund
- Collaborative Research Center 531 – University of Dortmund
External links
- http://www.scholarpedia.org/article/Evolution_Strategies :A peer-reviewed discussion of the subject.
- Animation: Optimization of a Two-Phase Flashing Nozzle with an Evolution Strategy. Animation of the Classical Experimental Optimization of a two phase flashing nozzle made by Professor Hans-Paul Schwefel and J. Klockgether. The result was shown at the Proceedings of the 11th Symposium on Engineering Aspects of Magneto-Hydrodynamics, Caltech, Pasadena, Cal., 24.–26.3. 1970.
- CMA Evolution Strategy – a contemporary variant where the complete covariance matrix of the multivariate normal mutation distribution is adapted.
- Comparison of Evolutionary Algorithms on a Benchmark Function Set – The 2005 IEEE Congress on Evolutionary Computation: Session on Real-Parameter Optimization - The CMA-ES (Covariance Matrix Adaptation Evolution Strategy) applied in a benchmark function set and compared to nine other Evolutionary Algorithms.
- Evolution Strategies – A brief description.
- Evolution Strategies Animations - Some interesting animations and real world problems (such as format of lenses, bridges configurations, etc) solved through Evolution Strategies.
- Evolution Strategy in Action – 10 ES-Demonstrations. By Michael Herdy and Gianino Patone – 10 problems solved through Evolution Strategies.
- Evolutionary Algorithms Demos – There are some applets with Evolution Strategies and Genetic Algorithms that the user can manipulate to solve problems. Very interesting for a comparison between the two Evolutionary Algorithms.
- Evolutionary Car Racing Videos – The application of Evolution Strategies to evolve cars' behaviours.
- EvoWeb. – The European Network of Excellence in Evolutionary Computing.
- Learning To Fly: Evolving Helicopter Flight Through Simulated Evolution – A (10 + 23)-ES applied to evolve a helicopter flight controller.
- Professor Hans-Paul Schwefel talks to EvoNews – An interview with Professor Hans-Paul Schwefel, one of the Evolution Strategy pioneers.