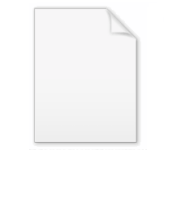
Quasi-likelihood
Encyclopedia
In statistics
, quasi-likelihood estimation is one way of allowing for overdispersion
, that is, greater variability in the data than would be expected from the statistical model
used. It is most often used with models for count data or grouped binary data, i.e. data that otherwise be modelled using the Poisson or binomial
distribution.
The term quasi-likelihood function was introduced by Robert Wedderburn
in 1974 to describe a function which has similar properties to the log-likelihood function
, except that a quasi-likelihood function is not the log-likelihood corresponding to any actual probability distribution
. Quasi-likelihood models can be fitted using a straightforward extension of the algorithms used to fit generalized linear models.
Instead of specifying a probability distribution for the data, only a relationship between the mean and the variance is specified in the form of a variance function giving the variance as a function of the mean. Generally, this function is allowed to include a multiplicative factor known as the overdispersion parameter or scale parameter that is estimated from the data. Most commonly, the variance function is of a form such that fixing the overdispersion parameter at unity results in the variance-mean relationship of an actual probability distribution such as the binomial or Poisson. (For formulae, see the binomial data example and count data example under generalized linear models.)
s (hierarchical models) provide an alternative method of fitting data exhibiting overdispersion using fully specified probability models. However, these methods often become complex and computationally intensive to fit to binary or count data. Quasi-likelihood methods have the advantage of relative computational simplicity, speed and robustness, as they can make use of the more straightforward algorithms developed to fit generalized linear models.
Quasi-likelihood has no role in Bayesian statistics
, as this is based on a fully specified probability model for the data.
Statistics
Statistics is the study of the collection, organization, analysis, and interpretation of data. It deals with all aspects of this, including the planning of data collection in terms of the design of surveys and experiments....
, quasi-likelihood estimation is one way of allowing for overdispersion
Overdispersion
In statistics, overdispersion is the presence of greater variability in a data set than would be expected based on a given simple statistical model....
, that is, greater variability in the data than would be expected from the statistical model
Statistical model
A statistical model is a formalization of relationships between variables in the form of mathematical equations. A statistical model describes how one or more random variables are related to one or more random variables. The model is statistical as the variables are not deterministically but...
used. It is most often used with models for count data or grouped binary data, i.e. data that otherwise be modelled using the Poisson or binomial
Binomial
In algebra, a binomial is a polynomial with two terms —the sum of two monomials—often bound by parenthesis or brackets when operated upon...
distribution.
The term quasi-likelihood function was introduced by Robert Wedderburn
Robert Wedderburn (statistician)
Robert William Maclagan Wedderburn was a Scottish statistician who worked at the Rothamsted Experimental Station. He was co-developer, with John Nelder, of the generalized linear model methodology,...
in 1974 to describe a function which has similar properties to the log-likelihood function
Likelihood function
In statistics, a likelihood function is a function of the parameters of a statistical model, defined as follows: the likelihood of a set of parameter values given some observed outcomes is equal to the probability of those observed outcomes given those parameter values...
, except that a quasi-likelihood function is not the log-likelihood corresponding to any actual probability distribution
Probability distribution
In probability theory, a probability mass, probability density, or probability distribution is a function that describes the probability of a random variable taking certain values....
. Quasi-likelihood models can be fitted using a straightforward extension of the algorithms used to fit generalized linear models.
Instead of specifying a probability distribution for the data, only a relationship between the mean and the variance is specified in the form of a variance function giving the variance as a function of the mean. Generally, this function is allowed to include a multiplicative factor known as the overdispersion parameter or scale parameter that is estimated from the data. Most commonly, the variance function is of a form such that fixing the overdispersion parameter at unity results in the variance-mean relationship of an actual probability distribution such as the binomial or Poisson. (For formulae, see the binomial data example and count data example under generalized linear models.)
Comparison to alternatives
Random-effects models, and more generally mixed modelMixed model
A mixed model is a statistical model containing both fixed effects and random effects, that is mixed effects. These models are useful in a wide variety of disciplines in the physical, biological and social sciences....
s (hierarchical models) provide an alternative method of fitting data exhibiting overdispersion using fully specified probability models. However, these methods often become complex and computationally intensive to fit to binary or count data. Quasi-likelihood methods have the advantage of relative computational simplicity, speed and robustness, as they can make use of the more straightforward algorithms developed to fit generalized linear models.
Quasi-likelihood has no role in Bayesian statistics
Bayesian statistics
Bayesian statistics is that subset of the entire field of statistics in which the evidence about the true state of the world is expressed in terms of degrees of belief or, more specifically, Bayesian probabilities...
, as this is based on a fully specified probability model for the data.