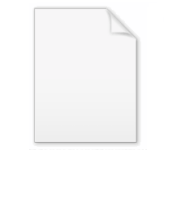
Goldfeld–Quandt test
Encyclopedia
In statistics
, the Goldfeld–Quandt test (named after Stephen Goldfeld
and Richard E. Quandt) checks for homoscedasticity
in regression analyses. It does this by dividing a dataset into two parts or groups, and hence the test is sometimes called a two-group test. The Goldfeld–Quandt test is one of two tests proposed in a 1965 paper by Stephen Goldfeld and Richard Quandt. Both a parametric and nonparametric test are described in the paper, but the term "Goldfeld–Quandt test" is usually associated only with the former.
analyses on two subsets of the original dataset: these subsets are specified so that the observations for which the pre-identified explanatory variable takes the lowest values are in one subset, with higher values in the other. The subsets needs not be of equal size, nor contain all the observations between them. The parametric test
assumes that the errors have a normal distribution. There is an additional assumption here, that the design matrices
for the two subsets of data are both of full rank. The test statistic
used is the ratio of the mean square residual errors for the regressions on the two subsets. This test statistic corresponds to an F-test of equality of variances
, and a one- or two-sided test may be appropriate depending on whether or not the direction of the supposed relation of the error variance to the explanatory variable is known.
Increasing the number of observations dropped in the "middle" of the ordering will increase the power
of the test but reduce the degrees of freedom for the test statistic. As a result of this tradeoff it is common to see the Goldfeld–Quandt test performed by dropping the middle third of observations with smaller proportions of dropped observations as sample size increases.
of the specified explanatory variable. For example, when faced with a quadratic function
mapping the explanatory variable to error variance the Goldfeld–Quandt test may improperly accept the null hypothesis of homoskedastic errors.
to specification errors. The Goldfeld–Quandt test detects non-homoskedastic errors but cannot distinguish between heteroskedastic error structure and an underlying specification problem
such as an incorrect functional form or an omitted variable. Jerry Thursby proposed a modification of the Goldfeld–Quandt test using a variation of the Ramsey RESET test
in order to provide some measure of robustness.
to test the power and sensitivity of the Goldfeld–Quandt test. His results show limited success for the Goldfeld–Quandt test except under cases of "pure heteroskedasticity"--where variance can be described as a function of only the underlying explanatory variable.
Statistics
Statistics is the study of the collection, organization, analysis, and interpretation of data. It deals with all aspects of this, including the planning of data collection in terms of the design of surveys and experiments....
, the Goldfeld–Quandt test (named after Stephen Goldfeld
Stephen Goldfeld
Stephen Goldfeld was a Princeton University professor and provost who served on the Council of Economic Advisers during the Carter administration....
and Richard E. Quandt) checks for homoscedasticity
Homoscedasticity
In statistics, a sequence or a vector of random variables is homoscedastic if all random variables in the sequence or vector have the same finite variance. This is also known as homogeneity of variance. The complementary notion is called heteroscedasticity...
in regression analyses. It does this by dividing a dataset into two parts or groups, and hence the test is sometimes called a two-group test. The Goldfeld–Quandt test is one of two tests proposed in a 1965 paper by Stephen Goldfeld and Richard Quandt. Both a parametric and nonparametric test are described in the paper, but the term "Goldfeld–Quandt test" is usually associated only with the former.
Test
In the context of multiple regression (or univariate regression), the hypothesis to be tested is that the variances of the errors of the regression model are not constant, but instead are monotonically related to a pre-identified explanatory variable. For example, data on income and consumption may be gathered and consumption regressed against income. If the variance increases as levels of income increase, then income may be used as an explanatory variable. Otherwise some third variable (e.g. wealth or last period income) may be chosen.Parametric test
The parametric test is accomplished by undertaking separate least squaresLeast squares
The method of least squares is a standard approach to the approximate solution of overdetermined systems, i.e., sets of equations in which there are more equations than unknowns. "Least squares" means that the overall solution minimizes the sum of the squares of the errors made in solving every...
analyses on two subsets of the original dataset: these subsets are specified so that the observations for which the pre-identified explanatory variable takes the lowest values are in one subset, with higher values in the other. The subsets needs not be of equal size, nor contain all the observations between them. The parametric test
Parametric statistics
Parametric statistics is a branch of statistics that assumes that the data has come from a type of probability distribution and makes inferences about the parameters of the distribution. Most well-known elementary statistical methods are parametric....
assumes that the errors have a normal distribution. There is an additional assumption here, that the design matrices
Design matrix
In statistics, a design matrix is a matrix of explanatory variables, often denoted by X, that is used in certain statistical models, e.g., the general linear model....
for the two subsets of data are both of full rank. The test statistic
Test statistic
In statistical hypothesis testing, a hypothesis test is typically specified in terms of a test statistic, which is a function of the sample; it is considered as a numerical summary of a set of data that...
used is the ratio of the mean square residual errors for the regressions on the two subsets. This test statistic corresponds to an F-test of equality of variances
F-test of equality of variances
In statistics, an F-test for the null hypothesis that two normal populations have the same variance is sometimes used, although it needs to be used with caution as it can be sensitive to the assumption that the variables have this distribution....
, and a one- or two-sided test may be appropriate depending on whether or not the direction of the supposed relation of the error variance to the explanatory variable is known.
Increasing the number of observations dropped in the "middle" of the ordering will increase the power
Statistical power
The power of a statistical test is the probability that the test will reject the null hypothesis when the null hypothesis is actually false . The power is in general a function of the possible distributions, often determined by a parameter, under the alternative hypothesis...
of the test but reduce the degrees of freedom for the test statistic. As a result of this tradeoff it is common to see the Goldfeld–Quandt test performed by dropping the middle third of observations with smaller proportions of dropped observations as sample size increases.
Nonparametric test
The second test proposed in the paper is a nonparametric one and hence does not rely on the assumption that the errors have a normal distribution. For this test, a single regression model is fitted to the complete dataset. The squares of the residuals are listed according to the order of the pre-identified explanatory variable. The test statistic used to test for homogeneity is the number of peaks in this list: ie. the count of the number of cases in which a squared residual is larger than all previous squared residuals. Critical values for this test statistic are constructed by an argument related to permutation tests.Advantages and disadvantages
The parametric Goldfeld–Quandt test offers a simple and intuitive diagnostic for heteroskedastic errors in a univariate or multivariate regression model. However some disadvantages arise under certain specifications or in comparison to other diagnostics, namely the Breusch–Pagan test, as the Goldfeld–Quandt test is somewhat of an ad hoc test. Primarily, the Goldfeld–Quandt test requires that data be ordered along a known explanatory variable. The parametric test orders along this explanatory variable from lowest to highest. If the error structure depends on an unknown variable or an unobserved variable the Goldfeld–Quandt test provides little guidance. Also, error variance must be a monotonic functionMonotonic function
In mathematics, a monotonic function is a function that preserves the given order. This concept first arose in calculus, and was later generalized to the more abstract setting of order theory....
of the specified explanatory variable. For example, when faced with a quadratic function
Quadratic function
A quadratic function, in mathematics, is a polynomial function of the formf=ax^2+bx+c,\quad a \ne 0.The graph of a quadratic function is a parabola whose axis of symmetry is parallel to the y-axis....
mapping the explanatory variable to error variance the Goldfeld–Quandt test may improperly accept the null hypothesis of homoskedastic errors.
Robustness
Unfortunately the Goldfeld–Quandt test is not very robustRobust statistics
Robust statistics provides an alternative approach to classical statistical methods. The motivation is to produce estimators that are not unduly affected by small departures from model assumptions.- Introduction :...
to specification errors. The Goldfeld–Quandt test detects non-homoskedastic errors but cannot distinguish between heteroskedastic error structure and an underlying specification problem
Specification (regression)
In regression analysis and related fields such as econometrics, specification is the process of converting a theory into a regression model. This process consists of selecting an appropriate functional form for the model and choosing which variables to include. Model specification is one of the...
such as an incorrect functional form or an omitted variable. Jerry Thursby proposed a modification of the Goldfeld–Quandt test using a variation of the Ramsey RESET test
Ramsey reset test
The Ramsey Regression Equation Specification Error Test test is a general specification test for the linear regression model. More specifically, it tests whether non-linear combinations of the estimated values help explain the endogenous variable...
in order to provide some measure of robustness.
Small sample properties
Henry Glejser, in his 1969 paper outlining the Glejser test, provides a small sampling experimentMonte Carlo method
Monte Carlo methods are a class of computational algorithms that rely on repeated random sampling to compute their results. Monte Carlo methods are often used in computer simulations of physical and mathematical systems...
to test the power and sensitivity of the Goldfeld–Quandt test. His results show limited success for the Goldfeld–Quandt test except under cases of "pure heteroskedasticity"--where variance can be described as a function of only the underlying explanatory variable.