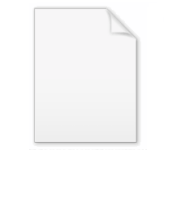
CURE data clustering algorithm
Encyclopedia
CURE is an efficient data clustering
algorithm for large database
s that is more robust
to outlier
s and identifies clusters having non-spherical shapes and wide variances in size.
when there are large differences in sizes or geometries of different clusters, the square error method could split the large clusters to minimize the square error which is not always correct. Also, with hierarchic clustering algorithms these problems exist as none of the distance measures between clusters (
) tend to work with different shapes of clusters. Also the running time
is high when n is very large. The problem with the BIRCH algorithm
is that once the clusters are generated after step 3, it uses centroids of the clusters and assign each data point
to the cluster with closest centroid. Using only the centroid to redistribute the data has problems when clusters do not have uniform sizes and shapes.
algorithm that adopts a middle ground
between the centroid based and all point extremes. In CURE, a constant number c of well scattered points of a cluster are chosen and they are shrunk towards the centroid of the cluster by a fraction α. The scattered points after shrinking are used as representatives of the cluster. The clusters with the closest pair of representatives are the clusters that are merged at each step of CURE's hierarchical clustering algorithm. This enables CURE to correctly identify the clusters and makes it less sensitive to outliers.
The algorithm is given below.
The running time of the algorithm is O(n2 log n) and space complexity
is O(n).
The algorithm cannot be directly applied to large databases. So for this purpose we do the following enhancements
Input : A set of points S
Output : k clusters
Data clustering
Cluster analysis or clustering is the task of assigning a set of objects into groups so that the objects in the same cluster are more similar to each other than to those in other clusters....
algorithm for large database
Database
A database is an organized collection of data for one or more purposes, usually in digital form. The data are typically organized to model relevant aspects of reality , in a way that supports processes requiring this information...
s that is more robust
Robust statistics
Robust statistics provides an alternative approach to classical statistical methods. The motivation is to produce estimators that are not unduly affected by small departures from model assumptions.- Introduction :...
to outlier
Outlier
In statistics, an outlier is an observation that is numerically distant from the rest of the data. Grubbs defined an outlier as: An outlying observation, or outlier, is one that appears to deviate markedly from other members of the sample in which it occurs....
s and identifies clusters having non-spherical shapes and wide variances in size.
Drawbacks of traditional algorithms
With the partitional clustering algorithms, which for example use the sum of squared errors criterionwhen there are large differences in sizes or geometries of different clusters, the square error method could split the large clusters to minimize the square error which is not always correct. Also, with hierarchic clustering algorithms these problems exist as none of the distance measures between clusters (

Analysis of algorithms
To analyze an algorithm is to determine the amount of resources necessary to execute it. Most algorithms are designed to work with inputs of arbitrary length...
is high when n is very large. The problem with the BIRCH algorithm
BIRCH (data clustering)
BIRCH is an unsupervised data mining algorithm used to perform hierarchical clustering over particularly large data-sets...
is that once the clusters are generated after step 3, it uses centroids of the clusters and assign each data point
Data point
In statistics, a data point is a set of measurements on a single member of a statistical population, or a subset of those measurements for a given individual...
to the cluster with closest centroid. Using only the centroid to redistribute the data has problems when clusters do not have uniform sizes and shapes.
CURE clustering algorithm
To avoid the problems with non-uniform sized or shaped clusters, CURE employs a novel hierarchical clusteringHierarchical clustering
In statistics, hierarchical clustering is a method of cluster analysis which seeks to build a hierarchy of clusters. Strategies for hierarchical clustering generally fall into two types:...
algorithm that adopts a middle ground
Middle ground
Argument to moderation is a logical fallacy which asserts that given two positions there exists a compromise between them which must be correct.An individual demonstrating the false compromise fallacy implies that the positions...
between the centroid based and all point extremes. In CURE, a constant number c of well scattered points of a cluster are chosen and they are shrunk towards the centroid of the cluster by a fraction α. The scattered points after shrinking are used as representatives of the cluster. The clusters with the closest pair of representatives are the clusters that are merged at each step of CURE's hierarchical clustering algorithm. This enables CURE to correctly identify the clusters and makes it less sensitive to outliers.
The algorithm is given below.
The running time of the algorithm is O(n2 log n) and space complexity
Computational complexity theory
Computational complexity theory is a branch of the theory of computation in theoretical computer science and mathematics that focuses on classifying computational problems according to their inherent difficulty, and relating those classes to each other...
is O(n).
The algorithm cannot be directly applied to large databases. So for this purpose we do the following enhancements
- Random sampling : To handle large data sets, we do random samplingSampling (statistics)In statistics and survey methodology, sampling is concerned with the selection of a subset of individuals from within a population to estimate characteristics of the whole population....
and draw a sample data setData setA data set is a collection of data, usually presented in tabular form. Each column represents a particular variable. Each row corresponds to a given member of the data set in question. Its values for each of the variables, such as height and weight of an object or values of random numbers. Each...
. Generally the random sampleRandom sampleIn statistics, a sample is a subject chosen from a population for investigation; a random sample is one chosen by a method involving an unpredictable component...
fits in main memory. Also because of the random sampling there is a trade offTrade-offA trade-off is a situation that involves losing one quality or aspect of something in return for gaining another quality or aspect...
between accuracy and efficiency.
- Partitioning for speed up : The basic idea is to partition the sample space into p partitions. Then in the first pass partially cluster each partition until the final number of clusters reduces to np/q for some constant q ≥ 1. Then run a second clustering pass on n/q partial clusters for all the partitions. For the second pass we only store the representative points since the merge procedure only requires representative points of previous clusters before computing the new representative points for the merged cluster. The advantage of partitioning the input is that we can reduce the execution times.
- Labeling data on disk : Since we only have representative points for k clusters, the remaining data points should also be assigned to the clusters. For this a fraction of randomly selected representative points for each of the k clusters is chosen and data point is assigned to the cluster containing the representative point closest to it.
Pseudocode
CURE(no. of points,k)Input : A set of points S
Output : k clusters
- For every cluster u (each input point), in u.mean and u.rep store the mean of the points in the cluster and a set of c representative points of the cluster (initially c = 1 since each cluster has one data point). Also u.closest stores the cluster closest to u.
- All the input points are inserted into a k-d treeKd-treeIn computer science, a k-d tree is a space-partitioning data structure for organizing points in a k-dimensional space. k-d trees are a useful data structure for several applications, such as searches involving a multidimensional search key...
T - Treat each input point as separate cluster, compute u.closest for each u and then insert each cluster into the heap Q. (clusters are arranged in increasing order of distances between u and u.closest).
- While size(Q) > k
- Remove the top elemnt of Q(say u) and merge it with its closest cluster u.closest(say v) and compute
- the new representative points for the merged cluster w. Also remove u and v from T and Q.
- Also for all the clusters x in Q, update x.closest and relocate x
- insert w into Q
- repeat