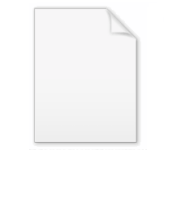
Autoregressive moving average model
Encyclopedia
In statistics
and signal processing
, autoregressive–moving-average (ARMA) models, sometimes called Box–Jenkins models after the iterative Box–Jenkins methodology usually used to estimate them, are typically applied to autocorrelated time series
data.
Given a time series of data Xt, the ARMA model is a tool for understanding and, perhaps, predicting future values in this series. The model consists of two parts, an autoregressive (AR) part and a moving average (MA) part. The model is usually then referred to as the ARMA(p,q) model where p is the order of the autoregressive part and q is the order of the moving average part (as defined below).

where
are the parameters of the model,
is a constant and
is white noise
. The constant term is omitted by many authors for simplicity.
An autoregressive model is essentially an all-pole infinite impulse response
filter with some additional interpretation placed on it.
Some constraints are necessary on the values of the parameters of this model in order that the model remains stationary
. For example, processes in the AR(1) model with |φ1| ≥ 1 are not stationary.

where the θ1, ..., θq are the parameters of the model, μ is the expectation of
(often assumed to equal 0), and the
,
,... are again, white noise
error terms. The moving-average model is essentially a finite impulse response
filter with some additional interpretation placed on it.

are generally assumed to be independent identically-distributed random variables (i.i.d.) sampled from a normal distribution with zero mean:
~ N(0,σ2) where σ2 is
the variance. These assumptions may be weakened but doing so will change the properties of the model. In particular, a change to the i.i.d. assumption would make a rather fundamental difference.
In these terms then the AR(p) model is given by

where
represents the polynomial

The MA(q) model is given by

where θ represents the polynomial

Finally, the combined ARMA(p, q) model is given by

or more concisely,


regression to find the values of the parameters which minimize the error term. It is generally considered good practice to find the smallest values of p and q which provide an acceptable fit to the data. For a pure AR model the Yule-Walker equations may be used to provide a fit.
Finding appropriate values of p and q in the ARMA(p,q) model can be facilitated by plotting the partial autocorrelation function
s for an estimate of p, and likewise using the autocorrelation functions for an estimate of q. Further information can be gleaned by considering the same functions for the residuals of a model fitted with an initial selection of p and q.
Brockwell and Davis (p.273) recommend using AICc
for finding p and q.
Autoregressive–moving-average models can be generalized in other ways. See also autoregressive conditional heteroskedasticity
(ARCH) models and autoregressive integrated moving average
(ARIMA) models. If multiple time series are to be fitted then a vector ARIMA (or VARIMA) model may be fitted. If the time-series in question exhibits long memory then fractional ARIMA (FARIMA, sometimes called ARFIMA) modelling may be appropriate: see Autoregressive fractionally integrated moving average
. If the data is thought to contain seasonal effects, it may be modeled by a SARIMA (seasonal ARIMA) or a periodic ARMA model.
Another generalization is the multiscale autoregressive (MAR) model. A MAR model is indexed by the nodes of a tree, whereas a standard (discrete time) autoregressive model is indexed by integers.
Note that the ARMA model is a univariate model. Extensions for the multivariate case are the Vector Autoregression
(VAR) and Vector Autoregression Moving-Average (VARMA).
The notation ARMAX(p, q, b) refers to the model with p autoregressive terms, q moving average terms and b exogenous inputs terms. This model contains the AR(p) and MA(q) models and a linear combination of the last b terms of a known and external time series
. It is given by:

where
are the parameters of the exogenous input
.
Some nonlinear variants of models with exogenous variables have been defined: see for example Nonlinear autoregressive exogenous model
.
Statistical packages implement the ARMAX model through the use of "exogenous" or "independent" variables. Care must be taken when interpreting the output of those packages, because the estimated parameters usually (for example, in R
and gretl
) refer to the regression:
where mt incorporates all exogenous (or independent) variables:
Statistics
Statistics is the study of the collection, organization, analysis, and interpretation of data. It deals with all aspects of this, including the planning of data collection in terms of the design of surveys and experiments....
and signal processing
Signal processing
Signal processing is an area of systems engineering, electrical engineering and applied mathematics that deals with operations on or analysis of signals, in either discrete or continuous time...
, autoregressive–moving-average (ARMA) models, sometimes called Box–Jenkins models after the iterative Box–Jenkins methodology usually used to estimate them, are typically applied to autocorrelated time series
Time series
In statistics, signal processing, econometrics and mathematical finance, a time series is a sequence of data points, measured typically at successive times spaced at uniform time intervals. Examples of time series are the daily closing value of the Dow Jones index or the annual flow volume of the...
data.
Given a time series of data Xt, the ARMA model is a tool for understanding and, perhaps, predicting future values in this series. The model consists of two parts, an autoregressive (AR) part and a moving average (MA) part. The model is usually then referred to as the ARMA(p,q) model where p is the order of the autoregressive part and q is the order of the moving average part (as defined below).
Autoregressive model
The notation AR(p) refers to the autoregressive model of order p. The AR(p) model is written
where



White noise
White noise is a random signal with a flat power spectral density. In other words, the signal contains equal power within a fixed bandwidth at any center frequency...
. The constant term is omitted by many authors for simplicity.
An autoregressive model is essentially an all-pole infinite impulse response
Infinite impulse response
Infinite impulse response is a property of signal processing systems. Systems with this property are known as IIR systems or, when dealing with filter systems, as IIR filters. IIR systems have an impulse response function that is non-zero over an infinite length of time...
filter with some additional interpretation placed on it.
Some constraints are necessary on the values of the parameters of this model in order that the model remains stationary
Stationary process
In the mathematical sciences, a stationary process is a stochastic process whose joint probability distribution does not change when shifted in time or space...
. For example, processes in the AR(1) model with |φ1| ≥ 1 are not stationary.
Moving-average model
The notation MA(q) refers to the moving average model of order q:
where the θ1, ..., θq are the parameters of the model, μ is the expectation of



White noise
White noise is a random signal with a flat power spectral density. In other words, the signal contains equal power within a fixed bandwidth at any center frequency...
error terms. The moving-average model is essentially a finite impulse response
Finite impulse response
A finite impulse response filter is a type of a signal processing filter whose impulse response is of finite duration, because it settles to zero in finite time. This is in contrast to infinite impulse response filters, which have internal feedback and may continue to respond indefinitely...
filter with some additional interpretation placed on it.
Autoregressive–moving-average model
The notation ARMA(p, q) refers to the model with p autoregressive terms and q moving-average terms. This model contains the AR(p) and MA(q) models,
Note about the error terms
The error terms

the variance. These assumptions may be weakened but doing so will change the properties of the model. In particular, a change to the i.i.d. assumption would make a rather fundamental difference.
Specification in terms of lag operator
In some texts the models will be specified in terms of the lag operator L.In these terms then the AR(p) model is given by

where


The MA(q) model is given by

where θ represents the polynomial

Finally, the combined ARMA(p, q) model is given by

or more concisely,

Alternative notation
Some authors, including Box, Jenkins & Reinsel use a different convention for the autoregression coefficients. This allows all the polynomials involving the lag operator to appear in a similar form throughout. Thus the ARMA model would be written as
Fitting models
ARMA models in general can, after choosing p and q, be fitted by least squaresLeast squares
The method of least squares is a standard approach to the approximate solution of overdetermined systems, i.e., sets of equations in which there are more equations than unknowns. "Least squares" means that the overall solution minimizes the sum of the squares of the errors made in solving every...
regression to find the values of the parameters which minimize the error term. It is generally considered good practice to find the smallest values of p and q which provide an acceptable fit to the data. For a pure AR model the Yule-Walker equations may be used to provide a fit.
Finding appropriate values of p and q in the ARMA(p,q) model can be facilitated by plotting the partial autocorrelation function
Partial autocorrelation function
In time series analysis, the partial autocorrelation function plays an important role in data analyses aimed at identifying the extent of the lag in an autoregressive model...
s for an estimate of p, and likewise using the autocorrelation functions for an estimate of q. Further information can be gleaned by considering the same functions for the residuals of a model fitted with an initial selection of p and q.
Brockwell and Davis (p.273) recommend using AICc
Akaike information criterion
The Akaike information criterion is a measure of the relative goodness of fit of a statistical model. It was developed by Hirotsugu Akaike, under the name of "an information criterion" , and was first published by Akaike in 1974...
for finding p and q.
Implementations in statistics packages
- In RR (programming language)R is a programming language and software environment for statistical computing and graphics. The R language is widely used among statisticians for developing statistical software, and R is widely used for statistical software development and data analysis....
, the tseries package includes an arma function. The function is documented in "Fit ARMA Models to Time Series". or use stats::arima - MathematicaMathematicaMathematica is a computational software program used in scientific, engineering, and mathematical fields and other areas of technical computing...
has a complete library of time series functions including ARMA - MATLABMATLABMATLAB is a numerical computing environment and fourth-generation programming language. Developed by MathWorks, MATLAB allows matrix manipulations, plotting of functions and data, implementation of algorithms, creation of user interfaces, and interfacing with programs written in other languages,...
includes a function ar to estimate AR models, see here for more details. - IMSL Numerical LibrariesIMSL Numerical LibrariesIMSL is a commercial collection of software libraries of numerical analysis functionality that are implemented in the computer programming languages of C, Java, C#.NET, and Fortran...
are libraries of numerical analysis functionality including ARMA and ARIMA procedures implemented in standard programming languages like C, Java, C# .NET, and Fortran. - gretlGretlgretl is an open-source statistical package, mainly for econometrics. The name is an acronym for Gnu Regression, Econometrics and Time-series Library. It has a graphical user interface and can be used together with X-12-ARIMA, TRAMO/SEATS, R, Octave, and Ox. It is written in C, uses GTK as widget...
can also estimate ARMA models, see here where it's mentioned. - GNU OctaveGNU OctaveGNU Octave is a high-level language, primarily intended for numerical computations. It provides a convenient command-line interface for solving linear and nonlinear problems numerically, and for performing other numerical experiments using a language that is mostly compatible with MATLAB...
can estimate AR models using functions from the extra package octave-forge. - SuanShu is a Java library of numerical methods, including comprehensive statistics packages, in which univariate/multivariate ARMA, ARIMA, ARMAX, etc. models are implemented in an object-oriented approach. These implementations are documented in "SuanShu, a Java numerical and statistical library".
- SAS has a econometric package, ETS, that estimates ARIMA models see here for more details.
Applications
ARMA is appropriate when a system is a function of a series of unobserved shocks (the MA part) as well as its own behavior. For example, stock prices may be shocked by fundamental information as well as exhibiting technical trending and mean-reversion effects due to market participants.Generalizations
The dependence of Xt on past values and the error terms εt is assumed to be linear unless specified otherwise. If the dependence is nonlinear, the model is specifically called a nonlinear moving average (NMA), nonlinear autoregressive (NAR), or nonlinear autoregressive–moving-average (NARMA) model.Autoregressive–moving-average models can be generalized in other ways. See also autoregressive conditional heteroskedasticity
Autoregressive conditional heteroskedasticity
In econometrics, AutoRegressive Conditional Heteroskedasticity models are used to characterize and model observed time series. They are used whenever there is reason to believe that, at any point in a series, the terms will have a characteristic size, or variance...
(ARCH) models and autoregressive integrated moving average
Autoregressive integrated moving average
In statistics and econometrics, and in particular in time series analysis, an autoregressive integrated moving average model is a generalization of an autoregressive moving average model. These models are fitted to time series data either to better understand the data or to predict future points...
(ARIMA) models. If multiple time series are to be fitted then a vector ARIMA (or VARIMA) model may be fitted. If the time-series in question exhibits long memory then fractional ARIMA (FARIMA, sometimes called ARFIMA) modelling may be appropriate: see Autoregressive fractionally integrated moving average
Autoregressive fractionally integrated moving average
In statistics, autoregressive fractionally integrated moving average models are time series models that generalize ARIMA models by allowing non-integer values of the differencing parameter and are useful in modeling time series with long memory...
. If the data is thought to contain seasonal effects, it may be modeled by a SARIMA (seasonal ARIMA) or a periodic ARMA model.
Another generalization is the multiscale autoregressive (MAR) model. A MAR model is indexed by the nodes of a tree, whereas a standard (discrete time) autoregressive model is indexed by integers.
Note that the ARMA model is a univariate model. Extensions for the multivariate case are the Vector Autoregression
Vector autoregression
Vector autoregression is a statistical model used to capture the linear interdependencies among multiple time series. VAR models generalize the univariate autoregression models. All the variables in a VAR are treated symmetrically; each variable has an equation explaining its evolution based on...
(VAR) and Vector Autoregression Moving-Average (VARMA).
Autoregressive–moving-average model with exogenous inputs model (ARMAX model)
The notation ARMAX(p, q, b) refers to the model with p autoregressive terms, q moving average terms and b exogenous inputs terms. This model contains the AR(p) and MA(q) models and a linear combination of the last b terms of a known and external time series


where


Some nonlinear variants of models with exogenous variables have been defined: see for example Nonlinear autoregressive exogenous model
Nonlinear autoregressive exogenous model
In time series modeling, a nonlinear autoregressive exogenous model is a nonlinear autoregressive model which has exogenous inputs. This means that the model relates the current value of a time series which one would like to explain or predict to both:...
.
Statistical packages implement the ARMAX model through the use of "exogenous" or "independent" variables. Care must be taken when interpreting the output of those packages, because the estimated parameters usually (for example, in R
R (programming language)
R is a programming language and software environment for statistical computing and graphics. The R language is widely used among statisticians for developing statistical software, and R is widely used for statistical software development and data analysis....
and gretl
Gretl
gretl is an open-source statistical package, mainly for econometrics. The name is an acronym for Gnu Regression, Econometrics and Time-series Library. It has a graphical user interface and can be used together with X-12-ARIMA, TRAMO/SEATS, R, Octave, and Ox. It is written in C, uses GTK as widget...
) refer to the regression:
where mt incorporates all exogenous (or independent) variables:
See also
- Exponential smoothingExponential smoothingExponential smoothing is a technique that can be applied to time series data, either to produce smoothed data for presentation, or to make forecasts. The time series data themselves are a sequence of observations. The observed phenomenon may be an essentially random process, or it may be an...
- Linear predictive codingLinear predictive codingLinear predictive coding is a tool used mostly in audio signal processing and speech processing for representing the spectral envelope of a digital signal of speech in compressed form, using the information of a linear predictive model...
- Predictive analyticsPredictive analyticsPredictive analytics encompasses a variety of statistical techniques from modeling, machine learning, data mining and game theory that analyze current and historical facts to make predictions about future events....
- Demetra+Demetra+Demetra+ is a program for seasonal adjustments that was developed and published by Eurostat – European Commission.- What is the seasonal adjustment? :...