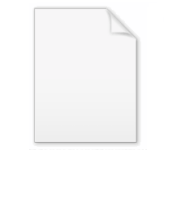
Shrinkage estimator
Encyclopedia
In statistics
, a shrinkage estimator is an estimator
that, either explicitly or implicitly, incorporates the effects of shrinkage
. In loose terms this means that a naïve or raw estimate is improved by combining it with other information. The term relates to the notion that the improved estimate is made closer to the value supplied by the 'other information' than the raw estimate. In this sense, shrinkage is used to regularize
ill-posed inference
problems.
One general result is that many standard estimators can be improved, in terms of mean squared error
(MSE), by shrinking them towards zero (or any other fixed constant value). Assume that the expected value of the raw estimate is not zero and consider other estimators obtained by multiplying the raw estimate by a certain parameter. A value for this parameter can be specified as that minimising the MSE of the new estimate. For this value of the parameter, the new estimate will have a smaller MSE than the raw one. Thus it has been improved. An effect here may be to convert an unbiased raw estimate to an improved biased one. A well-known example arises in the estimation of the population variance based on a simple sample; for a sample size of n, the use of a divisor n − 1 in the usual formula gives an unbiased estimator while a divisor of n + 1 gives one which has the minimum mean square error.
Shrinkage is implicit in Bayesian inference
and penalized likelihood inference, and explicit in James–Stein-type inference. In contrast, simple types of maximum-likelihood
and least-squares estimation procedures do not include shrinkage effects, although they can be used within shrinkage estimation schemes.
The use of shrinkage estimators in the context of regression analysis
, where there may be a large number of explanatory variables, has been described by Copas. Here the values of the estimated regression coefficients are shrunken towards zero with the effect of reducing the mean square error of predicted values from the model when applied to new data. A later paper by Copas applies shrinkage in a context where the problem is to predict a binary response on the basis of binary explanatory variables.
Statistics
Statistics is the study of the collection, organization, analysis, and interpretation of data. It deals with all aspects of this, including the planning of data collection in terms of the design of surveys and experiments....
, a shrinkage estimator is an estimator
Estimator
In statistics, an estimator is a rule for calculating an estimate of a given quantity based on observed data: thus the rule and its result are distinguished....
that, either explicitly or implicitly, incorporates the effects of shrinkage
Shrinkage (statistics)
In statistics, shrinkage has two meanings:*In relation to the general observation that, in regression analysis, a fitted relationship appears to perform less well on a new data set than on the data set used for fitting. In particular the value of the coefficient of determination 'shrinks'...
. In loose terms this means that a naïve or raw estimate is improved by combining it with other information. The term relates to the notion that the improved estimate is made closer to the value supplied by the 'other information' than the raw estimate. In this sense, shrinkage is used to regularize
Regularization (mathematics)
In mathematics and statistics, particularly in the fields of machine learning and inverse problems, regularization involves introducing additional information in order to solve an ill-posed problem or to prevent overfitting...
ill-posed inference
Statistical inference
In statistics, statistical inference is the process of drawing conclusions from data that are subject to random variation, for example, observational errors or sampling variation...
problems.
One general result is that many standard estimators can be improved, in terms of mean squared error
Mean squared error
In statistics, the mean squared error of an estimator is one of many ways to quantify the difference between values implied by a kernel density estimator and the true values of the quantity being estimated. MSE is a risk function, corresponding to the expected value of the squared error loss or...
(MSE), by shrinking them towards zero (or any other fixed constant value). Assume that the expected value of the raw estimate is not zero and consider other estimators obtained by multiplying the raw estimate by a certain parameter. A value for this parameter can be specified as that minimising the MSE of the new estimate. For this value of the parameter, the new estimate will have a smaller MSE than the raw one. Thus it has been improved. An effect here may be to convert an unbiased raw estimate to an improved biased one. A well-known example arises in the estimation of the population variance based on a simple sample; for a sample size of n, the use of a divisor n − 1 in the usual formula gives an unbiased estimator while a divisor of n + 1 gives one which has the minimum mean square error.
Shrinkage is implicit in Bayesian inference
Bayesian inference
In statistics, Bayesian inference is a method of statistical inference. It is often used in science and engineering to determine model parameters, make predictions about unknown variables, and to perform model selection...
and penalized likelihood inference, and explicit in James–Stein-type inference. In contrast, simple types of maximum-likelihood
Maximum likelihood
In statistics, maximum-likelihood estimation is a method of estimating the parameters of a statistical model. When applied to a data set and given a statistical model, maximum-likelihood estimation provides estimates for the model's parameters....
and least-squares estimation procedures do not include shrinkage effects, although they can be used within shrinkage estimation schemes.
The use of shrinkage estimators in the context of regression analysis
Regression analysis
In statistics, regression analysis includes many techniques for modeling and analyzing several variables, when the focus is on the relationship between a dependent variable and one or more independent variables...
, where there may be a large number of explanatory variables, has been described by Copas. Here the values of the estimated regression coefficients are shrunken towards zero with the effect of reducing the mean square error of predicted values from the model when applied to new data. A later paper by Copas applies shrinkage in a context where the problem is to predict a binary response on the basis of binary explanatory variables.
See also
- Stein's exampleStein's exampleStein's example , in decision theory and estimation theory, is the phenomenon that when three or more parameters are estimated simultaneously, there exist combined estimators more accurate on average than any method that handles the parameters separately...
- Shrinkage estimation in Estimation of covariance matricesEstimation of covariance matricesIn statistics, sometimes the covariance matrix of a multivariate random variable is not known but has to be estimated. Estimation of covariance matrices then deals with the question of how to approximate the actual covariance matrix on the basis of a sample from the multivariate distribution...
- Regularization (mathematics)Regularization (mathematics)In mathematics and statistics, particularly in the fields of machine learning and inverse problems, regularization involves introducing additional information in order to solve an ill-posed problem or to prevent overfitting...