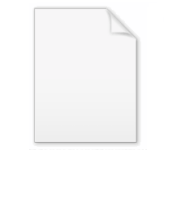
Probabilistic causation
Encyclopedia
Probabilistic causation designates a group of philosophical theories that aim to characterize the relationship between cause and effect using the tools of probability theory
. The central idea behind these theories is that causes raise the probabilities of their effects, all else being equal.
as a deterministic relation means that if A causes B, then A must always be followed by B. In this sense, war does not cause deaths, nor does smoking
cause cancer
. As a result, many turn to a notion of probabilistic causation. Informally, A probabilistically causes B if As occurrence increases the probability of B. This is sometimes interpreted to reflect imperfect knowledge of a deterministic system but other times interpreted to mean that the causal system under study has an inherently indeterministic nature. (Propensity probability
is an analogous idea, according to which probabilities have an objective existence and are not just limitations in a subject's knowledge).
Philosophers such as Hugh Mellor
and Patrick Suppes
have defined causation in terms of a cause preceding and increasing the probability of the effect. (Additionally, Mellor claims that cause and effect are both facts - not events - since even a non-event, such as the failure of a train to arrive, can cause effects such as my taking the bus. Suppes, by contrast, relies on events defined set-theoretically, and much of his discussion is informed by this terminology.)
Pearl argues that the entire enterprise of probabilistic
causation has been misguided from the very beginning, because
the central notion that causes "raise the probabilities" of their
effects cannot be expressed in the language of probability theory.
In particular, the inequality Pr(effect|cause) > Pr(effect|~cause) which philosophers invoked to define causation, as well as its
many variations and nuances, fails to capture the intuition
behind "probability raising", which is inherently a manipulative
or counterfactual notion.
The correct formulation, according to Pearl, should read:
Pr(effect|do(cause)) > Pr(effect|do(~cause))
where do(C) stands for an external intervention that compels the truth
of C. The conditional probability Pr(E|C), in contrast,
represents a probability resulting from a passive observation of
C, and rarely coincides with Pr(E|do(C)).
Indeed, observing the barometer falling
increases the probability of a storm coming, but does not
"cause" the storm; were the act
of manipulating the barometer to change the probability of
storms, the falling barometer would qualify as a cause of storms.
In general, formulating the notion of "probability raising" within
the calculus of do-operators resolves
the difficulties that probabilistic causation
has encountered in the past half-century, among them the
infamous Simpson's paradox
, and
clarifies precisely what relationships exist between
probabilities and causation.
The establishing of cause and effect, even with this relaxed reading, is notoriously difficult, expressed by the widely accepted statement "Correlation does not imply causation
". For instance, the observation that smokers have a dramatically increased lung cancer rate does not establish that smoking must be a cause of that increased cancer rate: maybe there exists a certain genetic defect which both causes cancer and a yearning for nicotine; or even perhaps nicotine craving is a symptom of very early-stage lung cancer which is not otherwise detectable. Scientists are always seeking the exact mechanisms by which Event A produces Event B. But scientists also are comfortable making a statement like, "Smoking probably causes cancer," when the statistical correlation between the two, according to probability theory, is far greater than chance. In this dual approach, scientists accept both deterministic and probabilistic causation in their terminology.
In statistics
, it is generally accepted that observational studies (like counting cancer cases among smokers and among non-smokers and then comparing the two) can give hints, but can never establish cause and effect. Often, however, qualitative causal assumptions (e.g., absence of causation between some variables) may permit the derivation of consistent
causal effect estimates from observational studies.
The gold standard for causation here is the randomized experiment: take a large number of people, randomly divide them into two groups, force one group to smoke and prohibit the other group from smoking, then determine whether one group develops a significantly higher lung cancer rate. Random assignment plays a crucial role in the inference to causation because, in the long run, it renders the two groups equivalent in terms of all other possible effects on the outcome (cancer) so that any changes in the outcome will reflect only the manipulation (smoking). Obviously, for ethical reasons this experiment
cannot be performed, but the method is widely applicable for less damaging experiments. One limitation of experiments, however, is that whereas they do a good job of testing for the presence of some causal effect they do less well at estimating the size of that effect in a population of interest. (This is a common criticism of studies of safety of food additives that use doses much higher than people consuming the product would actually ingest.)
Probability theory
Probability theory is the branch of mathematics concerned with analysis of random phenomena. The central objects of probability theory are random variables, stochastic processes, and events: mathematical abstractions of non-deterministic events or measured quantities that may either be single...
. The central idea behind these theories is that causes raise the probabilities of their effects, all else being equal.
Deterministic versus probabilistic theory
Interpreting causationCausality
Causality is the relationship between an event and a second event , where the second event is understood as a consequence of the first....
as a deterministic relation means that if A causes B, then A must always be followed by B. In this sense, war does not cause deaths, nor does smoking
Tobacco smoking
Tobacco smoking is the practice where tobacco is burned and the resulting smoke is inhaled. The practice may have begun as early as 5000–3000 BCE. Tobacco was introduced to Eurasia in the late 16th century where it followed common trade routes...
cause cancer
Cancer
Cancer , known medically as a malignant neoplasm, is a large group of different diseases, all involving unregulated cell growth. In cancer, cells divide and grow uncontrollably, forming malignant tumors, and invade nearby parts of the body. The cancer may also spread to more distant parts of the...
. As a result, many turn to a notion of probabilistic causation. Informally, A probabilistically causes B if As occurrence increases the probability of B. This is sometimes interpreted to reflect imperfect knowledge of a deterministic system but other times interpreted to mean that the causal system under study has an inherently indeterministic nature. (Propensity probability
Propensity probability
The propensity theory of probability is one interpretation of the concept of probability. Theorists who adopt this interpretation think of probability as a physical propensity, or disposition, or tendency of a given type of physical situation to yield an outcome of a certain kind, or to yield a...
is an analogous idea, according to which probabilities have an objective existence and are not just limitations in a subject's knowledge).
Philosophers such as Hugh Mellor
Hugh Mellor
David Hugh Mellor is an English philosopher.Mellor was born on 10 July 1938 in London. After studying chemical engineering at university, he took up philosophy. His main work has been in metaphysics....
and Patrick Suppes
Patrick Suppes
Patrick Colonel Suppes is an American philosopher who has made significant contributions to philosophy of science, the theory of measurement, the foundations of quantum mechanics, decision theory, psychology, and educational technology...
have defined causation in terms of a cause preceding and increasing the probability of the effect. (Additionally, Mellor claims that cause and effect are both facts - not events - since even a non-event, such as the failure of a train to arrive, can cause effects such as my taking the bus. Suppes, by contrast, relies on events defined set-theoretically, and much of his discussion is informed by this terminology.)
Pearl argues that the entire enterprise of probabilistic
causation has been misguided from the very beginning, because
the central notion that causes "raise the probabilities" of their
effects cannot be expressed in the language of probability theory.
In particular, the inequality Pr(effect|cause) > Pr(effect|~cause) which philosophers invoked to define causation, as well as its
many variations and nuances, fails to capture the intuition
behind "probability raising", which is inherently a manipulative
or counterfactual notion.
The correct formulation, according to Pearl, should read:
Pr(effect|do(cause)) > Pr(effect|do(~cause))
where do(C) stands for an external intervention that compels the truth
of C. The conditional probability Pr(E|C), in contrast,
represents a probability resulting from a passive observation of
C, and rarely coincides with Pr(E|do(C)).
Indeed, observing the barometer falling
increases the probability of a storm coming, but does not
"cause" the storm; were the act
of manipulating the barometer to change the probability of
storms, the falling barometer would qualify as a cause of storms.
In general, formulating the notion of "probability raising" within
the calculus of do-operators resolves
the difficulties that probabilistic causation
has encountered in the past half-century, among them the
infamous Simpson's paradox
Simpson's paradox
In probability and statistics, Simpson's paradox is a paradox in which a correlation present in different groups is reversed when the groups are combined. This result is often encountered in social-science and medical-science statistics, and it occurs when frequencydata are hastily given causal...
, and
clarifies precisely what relationships exist between
probabilities and causation.
The establishing of cause and effect, even with this relaxed reading, is notoriously difficult, expressed by the widely accepted statement "Correlation does not imply causation
Correlation does not imply causation
"Correlation does not imply causation" is a phrase used in science and statistics to emphasize that correlation between two variables does not automatically imply that one causes the other "Correlation does not imply causation" (related to "ignoring a common cause" and questionable cause) is a...
". For instance, the observation that smokers have a dramatically increased lung cancer rate does not establish that smoking must be a cause of that increased cancer rate: maybe there exists a certain genetic defect which both causes cancer and a yearning for nicotine; or even perhaps nicotine craving is a symptom of very early-stage lung cancer which is not otherwise detectable. Scientists are always seeking the exact mechanisms by which Event A produces Event B. But scientists also are comfortable making a statement like, "Smoking probably causes cancer," when the statistical correlation between the two, according to probability theory, is far greater than chance. In this dual approach, scientists accept both deterministic and probabilistic causation in their terminology.
In statistics
Statistics
Statistics is the study of the collection, organization, analysis, and interpretation of data. It deals with all aspects of this, including the planning of data collection in terms of the design of surveys and experiments....
, it is generally accepted that observational studies (like counting cancer cases among smokers and among non-smokers and then comparing the two) can give hints, but can never establish cause and effect. Often, however, qualitative causal assumptions (e.g., absence of causation between some variables) may permit the derivation of consistent
causal effect estimates from observational studies.
The gold standard for causation here is the randomized experiment: take a large number of people, randomly divide them into two groups, force one group to smoke and prohibit the other group from smoking, then determine whether one group develops a significantly higher lung cancer rate. Random assignment plays a crucial role in the inference to causation because, in the long run, it renders the two groups equivalent in terms of all other possible effects on the outcome (cancer) so that any changes in the outcome will reflect only the manipulation (smoking). Obviously, for ethical reasons this experiment
Experiment
An experiment is a methodical procedure carried out with the goal of verifying, falsifying, or establishing the validity of a hypothesis. Experiments vary greatly in their goal and scale, but always rely on repeatable procedure and logical analysis of the results...
cannot be performed, but the method is widely applicable for less damaging experiments. One limitation of experiments, however, is that whereas they do a good job of testing for the presence of some causal effect they do less well at estimating the size of that effect in a population of interest. (This is a common criticism of studies of safety of food additives that use doses much higher than people consuming the product would actually ingest.)