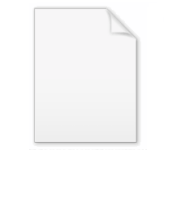
Data dredging
Encyclopedia
Data dredging is the inappropriate (sometimes deliberately so) use of data mining
to uncover misleading relationships in data. Data-snooping bias is a form of statistical bias that arises from this misuse of statistics
. Any relationships found might appear to be valid within the test set
but they would have no statistical significance
in the wider population.
Data dredging and data-snooping bias can occur when researchers either do not form a hypothesis in advance or narrow the data used to reduce the probability of the sample refuting a specific hypothesis. Although data-snooping bias can occur in any field that uses data mining, it is of particular concern in finance
and medical research, both of which make heavy use of data mining techniques.
The process of data mining involves automatically testing huge numbers of hypotheses about a single data set
by exhaustively searching for combinations of variables that might show a correlation. Conventional tests of statistical significance
are based on the probability that an observation arose by chance, and necessarily accept some risk of mistaken test results, called the significance
. When large numbers of tests are performed, it is always expected that some will produce false results, hence 5% of randomly chosen hypotheses will turn out to be significant at the 5% level, 1% will turn out to be significant at the 1% significance level, and so on, by chance alone.
If enough hypotheses are tested, it is virtually certain that some will falsely appear to be statistically significant, since every data set with any degree of randomness contains some bogus correlations. Researchers using data mining techniques can be easily misled by these apparently significant results, even though they are mere artifacts of random variation.
Circumventing the traditional scientific approach by conducting an experiment without a hypothesis can lead to premature conclusions. Data mining can be used negatively to seek more information from a data set than it actually contains. Failure to adjust existing statistical models when applying them to new datasets can also result in the occurrences of new patterns between different attributes that would otherwise have not shown up. Overfitting, oversearching
, overestimation
, and attribute selection errors are all actions that can lead to data dredging.
statistical hypothesis testing
procedure is to formulate a research hypothesis, such as "people in higher social classes live longer", then collect relevant data, followed by carrying out a statistical significance test to see whether the results could be due to the effects of chance. (The last step is called testing against the null hypothesis
).
A key point in proper statistical analysis is to test a hypothesis with evidence (data) that was not used in constructing the hypothesis. This is critical because every data set
will contain some patterns due entirely to chance. If the hypothesis is not tested on a different data set from the same population, it is impossible to determine if the patterns found are chance patterns. See testing hypotheses suggested by the data
.
Here is a simplistic example. Throwing five coins, with a result of 2 heads and 3 tails, might lead one to ask why the coin favors tails by fifty percent. On the other hand, forming the hypothesis might lead one to conclude that only a 5-0 or 0-5 result would be very surprising, since the odds are 93.75% against this happening by chance.
As a more visual example, on a cloudy day, try the experiment of looking for figures in the clouds; if one looks long enough one may see castles, cattle, and all sort of fanciful images; but the images are not really in the clouds, as can be easily confirmed by looking at other clouds.
It is important to realize that the alleged statistical significance here is completely spurious – significance tests do not protect against data dredging. When testing a data set on which the hypothesis is known to be true, the data set is by definition not a representative data set, and any resulting significance levels are meaningless.
Data snooping would, by design, try to find additional similarities between Mary and John, such as:
By going through hundreds or thousands of potential similarities between John and Mary, each having a low probability of being true, we may eventually find proof of virtually any hypothesis.
Perhaps John and Mary are the only two persons in the list who switched minors three times in college, a fact we found out by exhaustively comparing their lives' histories. Our data-snooping bias hypothesis can then become, "People born on August 7 have a much higher chance of switching minors more than twice in college."
The data itself very strongly supports that correlation, since no one with a different birthday had switched minors three times in college.
However, when we turn to the larger sample of the general population and attempt to reproduce the results, we find that there is no statistical correlation between August 7 birthdays and changing college minors more than once. The "fact" exists only for a very small, specific sample, not for the public as a whole.
, dataset A is often weather data up to the present, which ensures that, even subconsciously, subset B of the data could not influence the formulation of the hypothesis. Of course, such a discipline necessitates waiting for new data to come in, to show the formulated theory's predictive power
versus the null hypothesis
. This process ensures that no one can accuse the researcher of hand-tailoring the predictive model to the data on hand, since the upcoming weather is not yet available.
Suppose that observers note that a particular town appears to be a cancer cluster
, but lack a firm hypothesis of why this is so. However, they have access to a large amount of demographic data about the town and surrounding area, containing measurements for the area of hundreds or thousands of different variables, mostly uncorrelated. Even if all these variables are independent of the cancer incidence rate, it is highly likely that at least one variable will be significantly correlated with the cancer rate across the area. While this may suggest a hypothesis, further testing using the same variables but with data from a different location is needed to confirm. Note that a p-value
of 0.01 suggests that 1% of the time a result at least that extreme would be obtained by chance; if hundreds or thousands of hypotheses (with mutually relatively uncorrelated independent variables) are tested, then one is more likely than not to get at least one null hypothesis with a p-value less than 0.01.
Another remedy for data dredging is to record the number of all significance tests conducted during the experiment and simply multiply the final significance level by this number (the Bonferroni correction
); however, this is a very conservative metric. The use of a false discovery rate
is a more sophisticated approach that has become a popular method for control of multiple hypothesis tests.
Ultimately, the statistical significance of a test and the statistical confidence of a finding are joint properties of data and the method used to examine the data. Thus, if someone says that a certain event has probability of 20% ± 2% 19 times out of 20, this means that if the probability of the event is estimated by the same method used to obtain the 20% estimate, the result will be between 18% and 22% with probability 0.95. No claim of statistical significance can be made by only looking, without due regard to the method used to assess the data.
Data mining
Data mining , a relatively young and interdisciplinary field of computer science is the process of discovering new patterns from large data sets involving methods at the intersection of artificial intelligence, machine learning, statistics and database systems...
to uncover misleading relationships in data. Data-snooping bias is a form of statistical bias that arises from this misuse of statistics
Misuse of statistics
A misuse of statistics occurs when a statistical argument asserts a falsehood. In some cases, the misuse may be accidental. In others, it is purposeful and for the gain of the perpetrator. When the statistical reason involved is false or misapplied, this constitutes a statistical fallacy.The false...
. Any relationships found might appear to be valid within the test set
Test set
A test set is a set of data used in various areas of information science to assess the strength and utility of a predictive relationship. Test sets are used in artificial intelligence, machine learning, genetic programming, intelligent systems, and statistics...
but they would have no statistical significance
Statistical significance
In statistics, a result is called statistically significant if it is unlikely to have occurred by chance. The phrase test of significance was coined by Ronald Fisher....
in the wider population.
Data dredging and data-snooping bias can occur when researchers either do not form a hypothesis in advance or narrow the data used to reduce the probability of the sample refuting a specific hypothesis. Although data-snooping bias can occur in any field that uses data mining, it is of particular concern in finance
Finance
"Finance" is often defined simply as the management of money or “funds” management Modern finance, however, is a family of business activity that includes the origination, marketing, and management of cash and money surrogates through a variety of capital accounts, instruments, and markets created...
and medical research, both of which make heavy use of data mining techniques.
The process of data mining involves automatically testing huge numbers of hypotheses about a single data set
Data set
A data set is a collection of data, usually presented in tabular form. Each column represents a particular variable. Each row corresponds to a given member of the data set in question. Its values for each of the variables, such as height and weight of an object or values of random numbers. Each...
by exhaustively searching for combinations of variables that might show a correlation. Conventional tests of statistical significance
Statistical significance
In statistics, a result is called statistically significant if it is unlikely to have occurred by chance. The phrase test of significance was coined by Ronald Fisher....
are based on the probability that an observation arose by chance, and necessarily accept some risk of mistaken test results, called the significance
Statistical significance
In statistics, a result is called statistically significant if it is unlikely to have occurred by chance. The phrase test of significance was coined by Ronald Fisher....
. When large numbers of tests are performed, it is always expected that some will produce false results, hence 5% of randomly chosen hypotheses will turn out to be significant at the 5% level, 1% will turn out to be significant at the 1% significance level, and so on, by chance alone.
If enough hypotheses are tested, it is virtually certain that some will falsely appear to be statistically significant, since every data set with any degree of randomness contains some bogus correlations. Researchers using data mining techniques can be easily misled by these apparently significant results, even though they are mere artifacts of random variation.
Circumventing the traditional scientific approach by conducting an experiment without a hypothesis can lead to premature conclusions. Data mining can be used negatively to seek more information from a data set than it actually contains. Failure to adjust existing statistical models when applying them to new datasets can also result in the occurrences of new patterns between different attributes that would otherwise have not shown up. Overfitting, oversearching
Search algorithm
In computer science, a search algorithm is an algorithm for finding an item with specified properties among a collection of items. The items may be stored individually as records in a database; or may be elements of a search space defined by a mathematical formula or procedure, such as the roots...
, overestimation
Estimation theory
Estimation theory is a branch of statistics and signal processing that deals with estimating the values of parameters based on measured/empirical data that has a random component. The parameters describe an underlying physical setting in such a way that their value affects the distribution of the...
, and attribute selection errors are all actions that can lead to data dredging.
Drawing conclusions from data
The conventional frequentistFrequency probability
Frequency probability is the interpretation of probability that defines an event's probability as the limit of its relative frequency in a large number of trials. The development of the frequentist account was motivated by the problems and paradoxes of the previously dominant viewpoint, the...
statistical hypothesis testing
Statistical hypothesis testing
A statistical hypothesis test is a method of making decisions using data, whether from a controlled experiment or an observational study . In statistics, a result is called statistically significant if it is unlikely to have occurred by chance alone, according to a pre-determined threshold...
procedure is to formulate a research hypothesis, such as "people in higher social classes live longer", then collect relevant data, followed by carrying out a statistical significance test to see whether the results could be due to the effects of chance. (The last step is called testing against the null hypothesis
Null hypothesis
The practice of science involves formulating and testing hypotheses, assertions that are capable of being proven false using a test of observed data. The null hypothesis typically corresponds to a general or default position...
).
A key point in proper statistical analysis is to test a hypothesis with evidence (data) that was not used in constructing the hypothesis. This is critical because every data set
Data set
A data set is a collection of data, usually presented in tabular form. Each column represents a particular variable. Each row corresponds to a given member of the data set in question. Its values for each of the variables, such as height and weight of an object or values of random numbers. Each...
will contain some patterns due entirely to chance. If the hypothesis is not tested on a different data set from the same population, it is impossible to determine if the patterns found are chance patterns. See testing hypotheses suggested by the data
Testing hypotheses suggested by the data
In statistics, hypotheses suggested by the data, if tested using the data set that suggested them, are likely to be accepted even when they are not true...
.
Here is a simplistic example. Throwing five coins, with a result of 2 heads and 3 tails, might lead one to ask why the coin favors tails by fifty percent. On the other hand, forming the hypothesis might lead one to conclude that only a 5-0 or 0-5 result would be very surprising, since the odds are 93.75% against this happening by chance.
As a more visual example, on a cloudy day, try the experiment of looking for figures in the clouds; if one looks long enough one may see castles, cattle, and all sort of fanciful images; but the images are not really in the clouds, as can be easily confirmed by looking at other clouds.
It is important to realize that the alleged statistical significance here is completely spurious – significance tests do not protect against data dredging. When testing a data set on which the hypothesis is known to be true, the data set is by definition not a representative data set, and any resulting significance levels are meaningless.
Hypothesis suggested by non-representative data
In a list of 367 people, at least two will have the same day and month of birth. Suppose Mary and John both celebrate birthdays on August 7.Data snooping would, by design, try to find additional similarities between Mary and John, such as:
- Are they the youngest and the oldest persons in the list?
- Have they met in person once? Twice? Three times?
- Do their fathers have the same first name, or mothers have the same maiden name?
By going through hundreds or thousands of potential similarities between John and Mary, each having a low probability of being true, we may eventually find proof of virtually any hypothesis.
Perhaps John and Mary are the only two persons in the list who switched minors three times in college, a fact we found out by exhaustively comparing their lives' histories. Our data-snooping bias hypothesis can then become, "People born on August 7 have a much higher chance of switching minors more than twice in college."
The data itself very strongly supports that correlation, since no one with a different birthday had switched minors three times in college.
However, when we turn to the larger sample of the general population and attempt to reproduce the results, we find that there is no statistical correlation between August 7 birthdays and changing college minors more than once. The "fact" exists only for a very small, specific sample, not for the public as a whole.
Example in meteorology
In meteorologyMeteorology
Meteorology is the interdisciplinary scientific study of the atmosphere. Studies in the field stretch back millennia, though significant progress in meteorology did not occur until the 18th century. The 19th century saw breakthroughs occur after observing networks developed across several countries...
, dataset A is often weather data up to the present, which ensures that, even subconsciously, subset B of the data could not influence the formulation of the hypothesis. Of course, such a discipline necessitates waiting for new data to come in, to show the formulated theory's predictive power
Predictive power
The predictive power of a scientific theory refers to its ability to generate testable predictions. Theories with strong predictive power are highly valued, because the predictions can often encourage the falsification of the theory...
versus the null hypothesis
Null hypothesis
The practice of science involves formulating and testing hypotheses, assertions that are capable of being proven false using a test of observed data. The null hypothesis typically corresponds to a general or default position...
. This process ensures that no one can accuse the researcher of hand-tailoring the predictive model to the data on hand, since the upcoming weather is not yet available.
Suppose that observers note that a particular town appears to be a cancer cluster
Cancer cluster
Cancer cluster is a term used by epidemiologists, statisticians, and public health workers to define an occurrence of a greater-than-expected number of cancer cases within a group of people in a geographic area over a period of time....
, but lack a firm hypothesis of why this is so. However, they have access to a large amount of demographic data about the town and surrounding area, containing measurements for the area of hundreds or thousands of different variables, mostly uncorrelated. Even if all these variables are independent of the cancer incidence rate, it is highly likely that at least one variable will be significantly correlated with the cancer rate across the area. While this may suggest a hypothesis, further testing using the same variables but with data from a different location is needed to confirm. Note that a p-value
P-value
In statistical significance testing, the p-value is the probability of obtaining a test statistic at least as extreme as the one that was actually observed, assuming that the null hypothesis is true. One often "rejects the null hypothesis" when the p-value is less than the significance level α ,...
of 0.01 suggests that 1% of the time a result at least that extreme would be obtained by chance; if hundreds or thousands of hypotheses (with mutually relatively uncorrelated independent variables) are tested, then one is more likely than not to get at least one null hypothesis with a p-value less than 0.01.
Remedies
The practice of looking for patterns in data is legitimate; the vice of applying a statistical test of significance (hypothesis testing) to the same data from which the pattern was learned is wrong. One way to construct hypotheses while avoiding the problems of data dredging is to conduct randomized out-of-sample tests. The researcher collects a data set, then randomly partitions it into two subsets, A and B. Only one subset - say, subset A - is examined for creating hypotheses. Once a hypothesis has been formulated, it must be tested on subset B, which was not used to construct the hypothesis. Only where such a hypothesis is also supported by B is it reasonable to believe that the hypothesis might be valid.Another remedy for data dredging is to record the number of all significance tests conducted during the experiment and simply multiply the final significance level by this number (the Bonferroni correction
Bonferroni correction
In statistics, the Bonferroni correction is a method used to counteract the problem of multiple comparisons. It was developed and introduced by Italian mathematician Carlo Emilio Bonferroni...
); however, this is a very conservative metric. The use of a false discovery rate
False discovery rate
False discovery rate control is a statistical method used in multiple hypothesis testing to correct for multiple comparisons. In a list of rejected hypotheses, FDR controls the expected proportion of incorrectly rejected null hypotheses...
is a more sophisticated approach that has become a popular method for control of multiple hypothesis tests.
Ultimately, the statistical significance of a test and the statistical confidence of a finding are joint properties of data and the method used to examine the data. Thus, if someone says that a certain event has probability of 20% ± 2% 19 times out of 20, this means that if the probability of the event is estimated by the same method used to obtain the 20% estimate, the result will be between 18% and 22% with probability 0.95. No claim of statistical significance can be made by only looking, without due regard to the method used to assess the data.
See also
- Base rate fallacyBase rate fallacyThe base rate fallacy, also called base rate neglect or base rate bias, is an error that occurs when the conditional probability of some hypothesis H given some evidence E is assessed without taking into account the "base rate" or "prior probability" of H and the total probability of evidence...
- Bonferroni inequalities
- False discovery rateFalse discovery rateFalse discovery rate control is a statistical method used in multiple hypothesis testing to correct for multiple comparisons. In a list of rejected hypotheses, FDR controls the expected proportion of incorrectly rejected null hypotheses...
- Multiple comparisonsMultiple comparisonsIn statistics, the multiple comparisons or multiple testing problem occurs when one considers a set of statistical inferences simultaneously. Errors in inference, including confidence intervals that fail to include their corresponding population parameters or hypothesis tests that incorrectly...
- PareidoliaPareidoliaPareidolia is a psychological phenomenon involving a vague and random stimulus being perceived as significant. Common examples include seeing images of animals or faces in clouds, the man in the moon or the Moon rabbit, and hearing hidden messages on records played in reverse...
- Predictive analyticsPredictive analyticsPredictive analytics encompasses a variety of statistical techniques from modeling, machine learning, data mining and game theory that analyze current and historical facts to make predictions about future events....