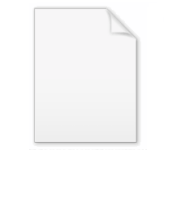
BrownBoost
Encyclopedia
BrownBoost is a boosting
algorithm that may be robust to noisy datasets. BrownBoost is an adaptive version of the boost by majority algorithm. As is true for all boosting
algorithms, BrownBoost is used in conjunction with other machine learning
methods. BrownBoost was introduced by Yoav Freund
in 2001.
performs well on a variety of datasets; however, it can be shown that AdaBoost does not perform well on noisy data sets. This is a result of AdaBoost's focus on examples that are repeatedly misclassified. In contrast, BrownBoost effectively "gives up" on examples that are repeatedly misclassified. The core assumption of BrownBoost is that noisy examples will be repeatedly mislabeled by the weak hypotheses and correctly non-noisy examples will be correctly labeled frequently enough to not be "given up on." Thus only noisy examples will be "given up on," whereas non-noisy examples will contribute to the final classifier. In turn, if the final classifier is learned from the non-noisy examples, the generalization error
of the final classifier may be much better than if learned from noisy and non-noisy examples.
The user of the algorithm can set the amount of error to be tolerated in the training set. Thus, if the training set is noisy (say 10% of all examples are assumed to be mislabeled), the booster can be told to accept a 10% error rate. Since the noisy examples may be ignored, only the true examples will contribute to the learning process.
and LogitBoost
), BrownBoost solves a system of two equations and two unknowns using standard numerical methods.
The only parameter of BrownBoost (
in the algorithm) is the "time" the algorithm runs. The theory of BrownBoost states that each hypothesis takes a variable amount of time (
in the algorithm) which is directly related to the weight given to the hypothesis
. The time parameter in BrownBoost is analogous to the number of iterations
in AdaBoost.
A larger value of
means that BrownBoost will treat the data as if it were less noisy and therefore will give up on fewer examples. Conversely, a smaller value of
means that
BrownBoost will treat the data as more noisy and give up on more examples.
During each iteration of the algorithm, a hypothesis is selected with some advantage over random guessing. The weight of this hypothesis
and the "amount of time passed"
during the iteration are simultaneously solved in a system of two non-linear equations ( 1. uncorrelate hypothesis w.r.t example weights and 2. hold the potential constant) with two unknowns (weight of hypothesis
and time passed
). This can be solved by bisection (as implemented in the JBoost software package) or Newton's method
(as described in the original paper by Freund). Once these equations are solved, the margins of each example (
in the algorithm) and the amount of time remaining
are updated appropriately. This process is repeated until there is no time remaining.
The initial potential is defined to be
. Since a constraint of each iteration is that the potential be held constant, the final potential is
. Thus the final error is likely to be near
. However, the final potential function is not the 0-1 loss error function. For the final error to be exactly
, the variance of the loss function must decrease linearly w.r.t. time to form the 0-1 loss function at the end of boosting iterations. This is not yet discussed in the literature and is not in the definition of the algorithm below.
The final classifier is a linear combination of weak hypotheses and is evaluated in the same manner as most other boosting algorithms.
Initialise:
While
:
Output:
's generalization error; however, LogitBoost
performed as well as BrownBoost. An implementation of BrownBoost can be found in the open source software JBoost.
Boosting
Boosting is a machine learning meta-algorithm for performing supervised learning. Boosting is based on the question posed by Kearns: can a set of weak learners create a single strong learner? A weak learner is defined to be a classifier which is only slightly correlated with the true classification...
algorithm that may be robust to noisy datasets. BrownBoost is an adaptive version of the boost by majority algorithm. As is true for all boosting
Boosting
Boosting is a machine learning meta-algorithm for performing supervised learning. Boosting is based on the question posed by Kearns: can a set of weak learners create a single strong learner? A weak learner is defined to be a classifier which is only slightly correlated with the true classification...
algorithms, BrownBoost is used in conjunction with other machine learning
Machine learning
Machine learning, a branch of artificial intelligence, is a scientific discipline concerned with the design and development of algorithms that allow computers to evolve behaviors based on empirical data, such as from sensor data or databases...
methods. BrownBoost was introduced by Yoav Freund
Yoav Freund
Yoav Freund is a researcher and professor at the University of California, San Diego who mainly works on machine learning, probability theory and related fields and applications.From his homepage:...
in 2001.
Motivation
AdaBoostAdaBoost
AdaBoost, short for Adaptive Boosting, is a machine learning algorithm, formulated by Yoav Freund and Robert Schapire. It is a meta-algorithm, and can be used in conjunction with many other learning algorithms to improve their performance. AdaBoost is adaptive in the sense that subsequent...
performs well on a variety of datasets; however, it can be shown that AdaBoost does not perform well on noisy data sets. This is a result of AdaBoost's focus on examples that are repeatedly misclassified. In contrast, BrownBoost effectively "gives up" on examples that are repeatedly misclassified. The core assumption of BrownBoost is that noisy examples will be repeatedly mislabeled by the weak hypotheses and correctly non-noisy examples will be correctly labeled frequently enough to not be "given up on." Thus only noisy examples will be "given up on," whereas non-noisy examples will contribute to the final classifier. In turn, if the final classifier is learned from the non-noisy examples, the generalization error
Generalization error
The generalization error of a machine learning model is a function that measures how far the student machine is from the teacher machine in average over the entire set of possible data that can be generated by the teacher after each iteration of the learning process...
of the final classifier may be much better than if learned from noisy and non-noisy examples.
The user of the algorithm can set the amount of error to be tolerated in the training set. Thus, if the training set is noisy (say 10% of all examples are assumed to be mislabeled), the booster can be told to accept a 10% error rate. Since the noisy examples may be ignored, only the true examples will contribute to the learning process.
Algorithm Description
BrownBoost uses a non-convex potential loss function, thus it does not fit into the AnyBoost framework. The non-convex optimization provides a method to avoid overfitting noisy data sets. However, in contrast to boosting algorithms that analytically minimize a convex loss function (e.g. AdaBoostAdaBoost
AdaBoost, short for Adaptive Boosting, is a machine learning algorithm, formulated by Yoav Freund and Robert Schapire. It is a meta-algorithm, and can be used in conjunction with many other learning algorithms to improve their performance. AdaBoost is adaptive in the sense that subsequent...
and LogitBoost
LogitBoost
LogitBoost is a boosting algorithm formulated by Jerome Friedman, Trevor Hastie, and Robert Tibshirani. The original paper casts the AdaBoost algorithm into a statistics framework...
), BrownBoost solves a system of two equations and two unknowns using standard numerical methods.
The only parameter of BrownBoost (




A larger value of



During each iteration of the algorithm, a hypothesis is selected with some advantage over random guessing. The weight of this hypothesis




Newton's method
In numerical analysis, Newton's method , named after Isaac Newton and Joseph Raphson, is a method for finding successively better approximations to the roots of a real-valued function. The algorithm is first in the class of Householder's methods, succeeded by Halley's method...
(as described in the original paper by Freund). Once these equations are solved, the margins of each example (


The initial potential is defined to be




The final classifier is a linear combination of weak hypotheses and is evaluated in the same manner as most other boosting algorithms.
BrownBoost Learning Algorithm Definition
Input:-
training examples
where
- The parameter
Initialise:
-
. The value of
is the amount of time remaining in the game)
-
. The value of
is the margin at iteration
for example
.
While

- Set the weights of each example:
, where
is the margin of example
- Find a classifier
such that
- Find values
that satisfy the equation:
.
(Note this is similar to the conditionset forth by Schapire and Singer. In this setting, we are numerically finding the
such that
.)
This update is subject to the constraint
,
whereis the potential loss for a point with margin
- Update the margins for each example:
- Update the time remaining:
Output:

Empirical Results
In preliminary experimental results with noisy datasets, BrownBoost outperformed AdaBoostAdaBoost
AdaBoost, short for Adaptive Boosting, is a machine learning algorithm, formulated by Yoav Freund and Robert Schapire. It is a meta-algorithm, and can be used in conjunction with many other learning algorithms to improve their performance. AdaBoost is adaptive in the sense that subsequent...
's generalization error; however, LogitBoost
LogitBoost
LogitBoost is a boosting algorithm formulated by Jerome Friedman, Trevor Hastie, and Robert Tibshirani. The original paper casts the AdaBoost algorithm into a statistics framework...
performed as well as BrownBoost. An implementation of BrownBoost can be found in the open source software JBoost.
See also
- BoostingBoostingBoosting is a machine learning meta-algorithm for performing supervised learning. Boosting is based on the question posed by Kearns: can a set of weak learners create a single strong learner? A weak learner is defined to be a classifier which is only slightly correlated with the true classification...
- AdaBoostAdaBoostAdaBoost, short for Adaptive Boosting, is a machine learning algorithm, formulated by Yoav Freund and Robert Schapire. It is a meta-algorithm, and can be used in conjunction with many other learning algorithms to improve their performance. AdaBoost is adaptive in the sense that subsequent...
- Alternating decision treeAlternating decision treeAn alternating decision tree is a machine learning method for classification. It generalizes decision trees and has connections to boosting.-History:...
s - JBoost