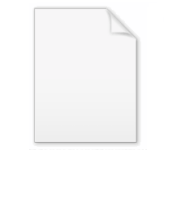
Berkson's paradox
Encyclopedia
Berkson's paradox or Berkson's fallacy is a result in conditional probability
and statistics
which is counter-intuitive for some people, and hence a veridical paradox
. It is a complicating factor arising in statistical tests of proportions. Specifically, it arises when there is an ascertainment bias inherent in a study design.
It is often described in the fields of medical statistics
or biostatistics
, as in the original description of the problem by Joseph Berkson.
events become conditionally dependent
(negatively dependent) given that at least one of them occurs. Symbolically:
In words, given two independent events, if you only consider outcomes where at least one occurs, then they become negatively dependent.
One can see this in tabular form as follows: the gray regions are the outcomes where at least one event occurs (and ~A means "not A").
For instance, if one has a sample of 100, and both A and B occur independently half the time (So P(A) = P(B) = 1/2), one obtains:
So in 75 outcomes, either A or B occurs, of which 50 have A occurring, so
Thus the probability of A is higher in the subset (of outcomes where it or B occurs), 2/3, than in the overall population, 1/2.
Berkson's paradox arises because the conditional probability of A given B within this subset equals the conditional probability in the overall population, but the unconditional probability within the subset is inflated relative to the unconditional probability in the overall population, hence, within the subset, the presence of B decreases the conditional probability of A (back to its overall unconditional probability):
for a disease in a statistical sample from a hospital
in-patient population. If a control group is also ascertained from the in-patient population, a difference in hospital admission rates for the case sample and control sample can result in a spurious association between the disease and the risk factor.
As another example, suppose a collector has 1000 postage stamp
s, of which 300 are pretty and 100 are rare, with 30 being both pretty and rare. 10% of all her stamps are rare and 10% of her pretty stamps are rare, so prettiness tells nothing about rarity. She puts the 370 stamps which are pretty or rare on display. Just over 27% of the stamps on display are rare, but still only 10% of the pretty stamps on display are rare (and 100% of the 70 not-pretty stamps on display are rare). If an observer only considers stamps on display, he will observe a spurious negative relationship between prettiness and rarity as a result of the selection bias
(that is, not-prettiness strongly indicates rarity in the display, but not in the total collection).
Conditional probability
In probability theory, the "conditional probability of A given B" is the probability of A if B is known to occur. It is commonly notated P, and sometimes P_B. P can be visualised as the probability of event A when the sample space is restricted to event B...
and statistics
Statistics
Statistics is the study of the collection, organization, analysis, and interpretation of data. It deals with all aspects of this, including the planning of data collection in terms of the design of surveys and experiments....
which is counter-intuitive for some people, and hence a veridical paradox
Paradox
Similar to Circular reasoning, A paradox is a seemingly true statement or group of statements that lead to a contradiction or a situation which seems to defy logic or intuition...
. It is a complicating factor arising in statistical tests of proportions. Specifically, it arises when there is an ascertainment bias inherent in a study design.
It is often described in the fields of medical statistics
Medical statistics
Medical statistics deals with applications of statistics to medicine and the health sciences, including epidemiology, public health, forensic medicine, and clinical research...
or biostatistics
Biostatistics
Biostatistics is the application of statistics to a wide range of topics in biology...
, as in the original description of the problem by Joseph Berkson.
Statement
The result is that two independentStatistical independence
In probability theory, to say that two events are independent intuitively means that the occurrence of one event makes it neither more nor less probable that the other occurs...
events become conditionally dependent
Conditional independence
In probability theory, two events R and B are conditionally independent given a third event Y precisely if the occurrence or non-occurrence of R and the occurrence or non-occurrence of B are independent events in their conditional probability distribution given Y...
(negatively dependent) given that at least one of them occurs. Symbolically:
- if 0 < P(A) < 1 and 0 < P(B) < 1,
- and P(A|B) = P(A), i.e. they are independent,
- then P(A|B,C) < P(A|C) where C = A∪B (i.e. A or B).
In words, given two independent events, if you only consider outcomes where at least one occurs, then they become negatively dependent.
Explanation
The cause is that the conditional probability of event A occurring, given that it or B occurs, is inflated: it is higher than the unconditional probability, because we have excluded cases where neither occur.- P(A|A∪B) > P(A)
- conditional probability inflated relative to unconditional
One can see this in tabular form as follows: the gray regions are the outcomes where at least one event occurs (and ~A means "not A").
A | ~A | |
---|---|---|
B | A & B | ~A & B |
~B | A & ~B | ~A & ~B |
For instance, if one has a sample of 100, and both A and B occur independently half the time (So P(A) = P(B) = 1/2), one obtains:
A | ~A | |
---|---|---|
B | 25 | 25 |
~B | 25 | 25 |
So in 75 outcomes, either A or B occurs, of which 50 have A occurring, so
- P(A|A∪B) = 50/75 = 2/3 > 1/2 = 50/100 = P(A).
Thus the probability of A is higher in the subset (of outcomes where it or B occurs), 2/3, than in the overall population, 1/2.
Berkson's paradox arises because the conditional probability of A given B within this subset equals the conditional probability in the overall population, but the unconditional probability within the subset is inflated relative to the unconditional probability in the overall population, hence, within the subset, the presence of B decreases the conditional probability of A (back to its overall unconditional probability):
- P(A|B, A∪B) = P(A|B) = P(A)
- P(A|A∪B) > P(A).
Examples
A classic illustration involves a retrospective study examining a risk factorRisk factor
In epidemiology, a risk factor is a variable associated with an increased risk of disease or infection. Sometimes, determinant is also used, being a variable associated with either increased or decreased risk.-Correlation vs causation:...
for a disease in a statistical sample from a hospital
Hospital
A hospital is a health care institution providing patient treatment by specialized staff and equipment. Hospitals often, but not always, provide for inpatient care or longer-term patient stays....
in-patient population. If a control group is also ascertained from the in-patient population, a difference in hospital admission rates for the case sample and control sample can result in a spurious association between the disease and the risk factor.
As another example, suppose a collector has 1000 postage stamp
Postage stamp
A postage stamp is a small piece of paper that is purchased and displayed on an item of mail as evidence of payment of postage. Typically, stamps are made from special paper, with a national designation and denomination on the face, and a gum adhesive on the reverse side...
s, of which 300 are pretty and 100 are rare, with 30 being both pretty and rare. 10% of all her stamps are rare and 10% of her pretty stamps are rare, so prettiness tells nothing about rarity. She puts the 370 stamps which are pretty or rare on display. Just over 27% of the stamps on display are rare, but still only 10% of the pretty stamps on display are rare (and 100% of the 70 not-pretty stamps on display are rare). If an observer only considers stamps on display, he will observe a spurious negative relationship between prettiness and rarity as a result of the selection bias
Selection bias
Selection bias is a statistical bias in which there is an error in choosing the individuals or groups to take part in a scientific study. It is sometimes referred to as the selection effect. The term "selection bias" most often refers to the distortion of a statistical analysis, resulting from the...
(that is, not-prettiness strongly indicates rarity in the display, but not in the total collection).