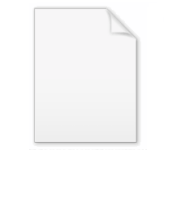
Bayesian additive regression kernels
Encyclopedia
Bayesian additive regression kernels (BARK) is a non-parametric statistical
model for regression
and statistical classification.
The unknown mean function is represented as a weighted sum of kernel functions, which is constructed by a prior using
alpha-stable Lévy random fields
. This leads to a specification of a joint prior distribution for the number of kernels, kernel regression coefficients and kernel location parameters. It can be shown that the alpha-stable prior on the kernel regression coefficients may be approximated by t distribution
s. With a heavy tail prior distribution on the kernel regression coefficients and a finite support on the kernel location parameter, BARK achieves sparse representations. The shape parameters in the kernel functions capture the non-linear interactions of the variables, which can be used for feature selection. A reversible-jump Markov chain Monte Carlo
algorithm is developed to make posterior inference on the unknown mean function, and the R package is available on CRAN. For binary classification
using a probit
link, the model can be augmented with latent normal variables and hence the same method for Gaussian noise applies in the classification problem.
Non-parametric statistics
In statistics, the term non-parametric statistics has at least two different meanings:The first meaning of non-parametric covers techniques that do not rely on data belonging to any particular distribution. These include, among others:...
model for regression
Regression analysis
In statistics, regression analysis includes many techniques for modeling and analyzing several variables, when the focus is on the relationship between a dependent variable and one or more independent variables...
and statistical classification.
The unknown mean function is represented as a weighted sum of kernel functions, which is constructed by a prior using
alpha-stable Lévy random fields
Lévy process
In probability theory, a Lévy process, named after the French mathematician Paul Lévy, is any continuous-time stochastic process that starts at 0, admits càdlàg modification and has "stationary independent increments" — this phrase will be explained below...
. This leads to a specification of a joint prior distribution for the number of kernels, kernel regression coefficients and kernel location parameters. It can be shown that the alpha-stable prior on the kernel regression coefficients may be approximated by t distribution
T distribution
The phrase "T distribution" may refer to* Student's t-test in univariate statistics,* Student's t-distribution in univariate probability theory,* Hotelling's T-square distribution in multivariate statistics.* Multivariate Student distribution....
s. With a heavy tail prior distribution on the kernel regression coefficients and a finite support on the kernel location parameter, BARK achieves sparse representations. The shape parameters in the kernel functions capture the non-linear interactions of the variables, which can be used for feature selection. A reversible-jump Markov chain Monte Carlo
Markov chain Monte Carlo
Markov chain Monte Carlo methods are a class of algorithms for sampling from probability distributions based on constructing a Markov chain that has the desired distribution as its equilibrium distribution. The state of the chain after a large number of steps is then used as a sample of the...
algorithm is developed to make posterior inference on the unknown mean function, and the R package is available on CRAN. For binary classification
Binary classification
Binary classification is the task of classifying the members of a given set of objects into two groups on the basis of whether they have some property or not. Some typical binary classification tasks are...
using a probit
Probit
In probability theory and statistics, the probit function is the inverse cumulative distribution function , or quantile function associated with the standard normal distribution...
link, the model can be augmented with latent normal variables and hence the same method for Gaussian noise applies in the classification problem.