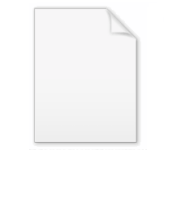
Backus–Gilbert method
Encyclopedia
In mathematics, the Backus–Gilbert method, also known as the optimally localized average (OLA) method is named for its discoverers, geophysicists George E. Backus and James Freeman Gilbert
. It is a regularization
method for obtaining meaningful solutions to ill-posed inverse problems
. Where other regularization methods, such as the frequently used Tikhonov regularization
method, seek to impose smoothness constraints on the solution, Backus–Gilbert instead seeks to impose stability constraints, so that the solution would vary as little as possible if the input data were resampled multiple times. In practice, and to the extent that is justified by the data, smoothness results from this.
James Freeman Gilbert
J. Freeman Gilbert is an American geophysicist, best known for his work with George E. Backus on inverting geophysical data, and also for his role in establishing an international network of long-period seismometers....
. It is a regularization
Regularization (mathematics)
In mathematics and statistics, particularly in the fields of machine learning and inverse problems, regularization involves introducing additional information in order to solve an ill-posed problem or to prevent overfitting...
method for obtaining meaningful solutions to ill-posed inverse problems
Inverse problem
An inverse problem is a general framework that is used to convert observed measurements into information about a physical object or system that we are interested in...
. Where other regularization methods, such as the frequently used Tikhonov regularization
Tikhonov regularization
Tikhonov regularization, named for Andrey Tikhonov, is the most commonly used method of regularization of ill-posed problems. In statistics, the method is known as ridge regression, and, with multiple independent discoveries, it is also variously known as the Tikhonov-Miller method, the...
method, seek to impose smoothness constraints on the solution, Backus–Gilbert instead seeks to impose stability constraints, so that the solution would vary as little as possible if the input data were resampled multiple times. In practice, and to the extent that is justified by the data, smoothness results from this.