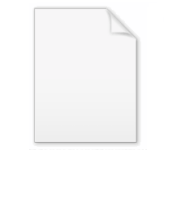
Version space
Encyclopedia
A version space in concept learning
or induction is the subset of all hypotheses that are consistent with the observed training examples. This set contains all hypotheses that have not been eliminated as a result of being in conflict with observed data.
The most specific hypotheses (i.e., the specific boundary SB) cover the observed positive training examples, and as little of the remaining feature space as possible. These hypotheses, if reduced any further, exclude a positive training example, and hence become inconsistent. These minimal hypotheses essentially constitute a (pessimistic) claim that the true concept is defined just by the positive data already observed: Thus, if a novel (never-before-seen) data point is observed, it should be assumed to be negative. (I.e., if data has not previously been ruled in, then it's ruled out.)
The most general hypotheses (i.e., the general boundary GB) cover the observed positive training examples, but also cover as much of the remaining feature space without including any negative training examples. These, if enlarged any further, include a negative training example, and hence become inconsistent. These maximal hypotheses essentially constitute a (optimistic) claim that the true concept is defined just by the negative data already observed: Thus, if a novel (never-before-seen) data point is observed, it should be assumed to be positive. (I.e., if data has not previously been ruled out, then it's ruled in.)
Thus, during the learning process, the version space (which itself is a set – possibly infinite – containing all consistent hypotheses) can be represented by just its lower and upper bounds (maximally general and maximally specific hypothesis sets), and learning operations can be performed just on these representative sets.
Concept learning
Concept learning, also known as category learning, concept attainment, and concept formation, is largely based on the works of the cognitive psychologist Jerome Bruner...
or induction is the subset of all hypotheses that are consistent with the observed training examples. This set contains all hypotheses that have not been eliminated as a result of being in conflict with observed data.
The Version Space algorithm
In settings where there is a generality-ordering on hypotheses, it is possible to represent the version space by two sets of hypotheses: (1) the most specific consistent hypotheses, and (2) the most general consistent hypotheses, where "consistent" indicates agreement with observed data.The most specific hypotheses (i.e., the specific boundary SB) cover the observed positive training examples, and as little of the remaining feature space as possible. These hypotheses, if reduced any further, exclude a positive training example, and hence become inconsistent. These minimal hypotheses essentially constitute a (pessimistic) claim that the true concept is defined just by the positive data already observed: Thus, if a novel (never-before-seen) data point is observed, it should be assumed to be negative. (I.e., if data has not previously been ruled in, then it's ruled out.)
The most general hypotheses (i.e., the general boundary GB) cover the observed positive training examples, but also cover as much of the remaining feature space without including any negative training examples. These, if enlarged any further, include a negative training example, and hence become inconsistent. These maximal hypotheses essentially constitute a (optimistic) claim that the true concept is defined just by the negative data already observed: Thus, if a novel (never-before-seen) data point is observed, it should be assumed to be positive. (I.e., if data has not previously been ruled out, then it's ruled in.)
Thus, during the learning process, the version space (which itself is a set – possibly infinite – containing all consistent hypotheses) can be represented by just its lower and upper bounds (maximally general and maximally specific hypothesis sets), and learning operations can be performed just on these representative sets.
Historical background
The notion of Version Spaces was introduced by Mitchell as a framework for understanding the basic problem of supervised learning within the context of solution search. Although the basic "candidate elimination" search method that accompanies the Version Space framework is not a popular learning algorithm (for various reasons, including the issue of noise (Russell & Norvig 2002)), there are some practical implementations that have been developed (e.g., Sverdlik & Reynolds 1992, Hong & Tsang 1997, Dubois & Quafafou 2002).See also
- Rough setRough setIn computer science, a rough set, first described by a Polish computer scientist Zdzisław I. Pawlak, is a formal approximation of a crisp set in terms of a pair of sets which give the lower and the upper approximation of the original set...
. [The rough set framework focuses on the case where ambiguity is introduced by an impoverished feature set. That is, the target concept cannot be decisively described because the available feature set fails to disambiguate objects belonging to different categories. The version space framework focuses on the (classical induction) case where the ambiguity is introduced by an impoverished data set. That is, the target concept cannot be decisively described because the available data fails to uniquely pick out a hypothesis. Naturally, both types of ambiguity can occur in the same learning problem.] provides an interesting discussion of the general problem of induction. - Mill (1843/2002) is the classic source on inductive logic.