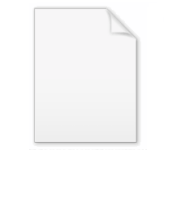
Strongly NP-complete
Encyclopedia
In computational complexity
, strong NP-completeness is a property of computational problems that is a special case of NP-completeness
. A general computational problem may have numerical parameters. For example, the input to the bin packing problem is a list of objects of specific sizes and a size for the bins that must contain the objects—these object sizes and bin size are numerical parameters.
A problem is said to be strongly NP-complete
(strongly NP-complete in the strong sense), if it remains so even when all of its numerical parameters are bounded by a polynomial in the length of the input. A problem is said to be strongly NP-hard
if a strongly NP-complete problem has a polynomial reduction to it; in combinatorial optimization, particularly, the phrase "strongly NP-hard" is reserved for problems that are not known to have a polynomial reduction to another strongly NP-complete problem.
Normally numerical parameters to a problem are given in binary notation, so a problem of input size n might contain parameters whose size is exponential
in n. If we redefine the problem to have the parameters given in unary notation
, then the parameters must be bounded by the input size. Thus strong NP-completeness or NP-hardness may also be defined as the NP-completeness or NP-hardness of this unary version of the problem.
For example, bin packing is strongly NP-complete while the 0-1 Knapsack problem is only weakly NP-complete
. Thus the version of bin packing where the object and bin sizes are integers bounded by a polynomial remains NP-complete, while the corresponding version of the Knapsack problem can be solved in polynomial time by dynamic programming
.
While weakly NP-complete problems may admit efficient solutions in practice as long as their inputs are of relatively small magnitude, strongly NP-complete problems do not admit efficient solutions in these cases. From a theoretical perspective any strongly NP-hard optimization problem with a polynomially bounded objective function cannot have an FPTAS unless P = NP. However, the converse fails: e.g. if P does not equal NP, knapsack with two constraints is not strongly NP-hard, but has no FPTAS even when the optimal objective is polynomially bounded.
Some strongly NP-complete problems may still be easy to solve on average, but it's more likely that difficult instances will be encountered in practice.
Unless P = NP, there is no fully polynomial-time approximation scheme (or FPTAS) for the strongly NP-complete problems.
Computational Complexity
Computational Complexity may refer to:*Computational complexity theory*Computational Complexity...
, strong NP-completeness is a property of computational problems that is a special case of NP-completeness
NP-complete
In computational complexity theory, the complexity class NP-complete is a class of decision problems. A decision problem L is NP-complete if it is in the set of NP problems so that any given solution to the decision problem can be verified in polynomial time, and also in the set of NP-hard...
. A general computational problem may have numerical parameters. For example, the input to the bin packing problem is a list of objects of specific sizes and a size for the bins that must contain the objects—these object sizes and bin size are numerical parameters.
A problem is said to be strongly NP-complete
NP-complete
In computational complexity theory, the complexity class NP-complete is a class of decision problems. A decision problem L is NP-complete if it is in the set of NP problems so that any given solution to the decision problem can be verified in polynomial time, and also in the set of NP-hard...
(strongly NP-complete in the strong sense), if it remains so even when all of its numerical parameters are bounded by a polynomial in the length of the input. A problem is said to be strongly NP-hard
NP-hard
NP-hard , in computational complexity theory, is a class of problems that are, informally, "at least as hard as the hardest problems in NP". A problem H is NP-hard if and only if there is an NP-complete problem L that is polynomial time Turing-reducible to H...
if a strongly NP-complete problem has a polynomial reduction to it; in combinatorial optimization, particularly, the phrase "strongly NP-hard" is reserved for problems that are not known to have a polynomial reduction to another strongly NP-complete problem.
Normally numerical parameters to a problem are given in binary notation, so a problem of input size n might contain parameters whose size is exponential
Exponential function
In mathematics, the exponential function is the function ex, where e is the number such that the function ex is its own derivative. The exponential function is used to model a relationship in which a constant change in the independent variable gives the same proportional change In mathematics,...
in n. If we redefine the problem to have the parameters given in unary notation
Unary numeral system
The unary numeral system is the bijective base-1 numeral system. It is the simplest numeral system to represent natural numbers: in order to represent a number N, an arbitrarily chosen symbol representing 1 is repeated N times. For example, using the symbol | , the number 6 is represented as ||||||...
, then the parameters must be bounded by the input size. Thus strong NP-completeness or NP-hardness may also be defined as the NP-completeness or NP-hardness of this unary version of the problem.
For example, bin packing is strongly NP-complete while the 0-1 Knapsack problem is only weakly NP-complete
Weakly NP-complete
In computational complexity, an NP-complete problem is weakly NP-complete , if there is an algorithm for the problem whose running time is polynomial in the dimension of the problem and the magnitudes of the data involved , rather than the base-two logarithms of their magnitudes...
. Thus the version of bin packing where the object and bin sizes are integers bounded by a polynomial remains NP-complete, while the corresponding version of the Knapsack problem can be solved in polynomial time by dynamic programming
Dynamic programming
In mathematics and computer science, dynamic programming is a method for solving complex problems by breaking them down into simpler subproblems. It is applicable to problems exhibiting the properties of overlapping subproblems which are only slightly smaller and optimal substructure...
.
While weakly NP-complete problems may admit efficient solutions in practice as long as their inputs are of relatively small magnitude, strongly NP-complete problems do not admit efficient solutions in these cases. From a theoretical perspective any strongly NP-hard optimization problem with a polynomially bounded objective function cannot have an FPTAS unless P = NP. However, the converse fails: e.g. if P does not equal NP, knapsack with two constraints is not strongly NP-hard, but has no FPTAS even when the optimal objective is polynomially bounded.
Some strongly NP-complete problems may still be easy to solve on average, but it's more likely that difficult instances will be encountered in practice.
Unless P = NP, there is no fully polynomial-time approximation scheme (or FPTAS) for the strongly NP-complete problems.