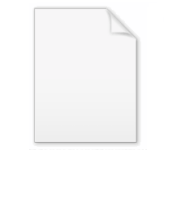
Propensity score matching
Encyclopedia
In the statistical
analysis of observational data
, propensity score matching (PSM) is a methodology attempting to provide unbiased
estimation of treatment-effects. The possibility of "bias" arises here because the effectiveness of a treatment may depend on characteristics that are associated with whether or not a participant in an observational study chooses, or is chosen, to receive a given treatment.
A treatment-effect is just jargon for the effect of something that is being studied -- like the consequences of smoking or the consequences of going to university. The people 'treated' are simply those -- the smokers, or the university graduates -- who undergo whatever it is that is being studied by the researcher. The language of 'treatment effects' comes originally from the medical literature where medical researchers have always hoped to isolate the true causal effects of different ways of dealing with disease. One way to do that is to run experiments.
In randomized experiment
s, the randomization enables unbiased estimation of treatment effects; for each covariate, randomization implies that treatment-groups will be balanced on average, by the law of large numbers
. Unfortunately, for observational studies, the assignment of treatments to research subjects has been haphazard and not randomized; lacking randomization, observational studies frequently provide biased estimation of treatment effects and have imbalance on covariates.
In observational studies, the "treatment"-groups (or "exposure" groups) often exhibit imbalance on covariates. This covariate imbalance is confounded with treatments: It is difficult to attribute differences in responses to the "treatment" or "exposure" because the covariates are also believed to influence the response. The propensity score matching attempts to reduce the confounding effects of covariates, and so allow differences of responses to be attributed to differences of treatments (exposures).
Researchers try to decide how the world works, and, in particular, what causes what. To do this properly, it is not enough to observe correlations. It is necessary to try to understand causality. In other words, even when it is seen that smoking and cancer tend to occur together in a sample of human beings, the basic questions remain:
Similarly, people with university degrees tend later in their lives to earn more money than others without degrees, but is that because the education is actually causing the higher earnings?
In normal Matching we match on single characteristics that distinguish treatment and control groups (to try to make them more alike). But If the two groups do not have substantial overlap, then substantial error may be introduced: E.g., if only the worst cases from the untreated “comparison” group are compared to only the best cases from the treatment group, the result may be regression toward the mean which may make the comparison group look better
or worse than reality.
PSM employs a predicted probability of group membership e.g., treatment vs. control group—based on observed predictors, usually obtained from logistic regression to create a counterfactual group. Also propensity score
s may be used for matching or as covariates—alone or with other matching variables or covariates.
published their seminal paper that first proposed this approach.
2.Match each participant to one or more nonparticipants on propensity score:
3.Multivariate analysis based on new sample
Michael Sosin, University of Chicago also identifies following problems with PSM:
Shadish, Cook, & Campbell (2002) identifies further shortcomings with PSM:
Pearl (2009) alerts to the following phenomena with PSM, as well as any matching method:
In general risks of PSM include:
Statistics
Statistics is the study of the collection, organization, analysis, and interpretation of data. It deals with all aspects of this, including the planning of data collection in terms of the design of surveys and experiments....
analysis of observational data
Observational study
In epidemiology and statistics, an observational study draws inferences about the possible effect of a treatment on subjects, where the assignment of subjects into a treated group versus a control group is outside the control of the investigator...
, propensity score matching (PSM) is a methodology attempting to provide unbiased
Bias (statistics)
A statistic is biased if it is calculated in such a way that it is systematically different from the population parameter of interest. The following lists some types of, or aspects of, bias which should not be considered mutually exclusive:...
estimation of treatment-effects. The possibility of "bias" arises here because the effectiveness of a treatment may depend on characteristics that are associated with whether or not a participant in an observational study chooses, or is chosen, to receive a given treatment.
A treatment-effect is just jargon for the effect of something that is being studied -- like the consequences of smoking or the consequences of going to university. The people 'treated' are simply those -- the smokers, or the university graduates -- who undergo whatever it is that is being studied by the researcher. The language of 'treatment effects' comes originally from the medical literature where medical researchers have always hoped to isolate the true causal effects of different ways of dealing with disease. One way to do that is to run experiments.
In randomized experiment
Randomized experiment
In science, randomized experiments are the experiments that allow the greatest reliability and validity of statistical estimates of treatment effects...
s, the randomization enables unbiased estimation of treatment effects; for each covariate, randomization implies that treatment-groups will be balanced on average, by the law of large numbers
Law of large numbers
In probability theory, the law of large numbers is a theorem that describes the result of performing the same experiment a large number of times...
. Unfortunately, for observational studies, the assignment of treatments to research subjects has been haphazard and not randomized; lacking randomization, observational studies frequently provide biased estimation of treatment effects and have imbalance on covariates.
In observational studies, the "treatment"-groups (or "exposure" groups) often exhibit imbalance on covariates. This covariate imbalance is confounded with treatments: It is difficult to attribute differences in responses to the "treatment" or "exposure" because the covariates are also believed to influence the response. The propensity score matching attempts to reduce the confounding effects of covariates, and so allow differences of responses to be attributed to differences of treatments (exposures).
Researchers try to decide how the world works, and, in particular, what causes what. To do this properly, it is not enough to observe correlations. It is necessary to try to understand causality. In other words, even when it is seen that smoking and cancer tend to occur together in a sample of human beings, the basic questions remain:
- is the smoking itself having a causal effect, or could it just be that cancer is caused by, say, poor diet and those who smoke tend not to eat healthy foods, or
- is some gene that leads to cancer and also by coincidence that that gene increases a person's enjoyment of cigarettes?
Similarly, people with university degrees tend later in their lives to earn more money than others without degrees, but is that because the education is actually causing the higher earnings?
Overview
PSM is for cases of causal inference and simple selection bias in non-experimental settings in which: (i) few units in the non-experimental comparison group are comparable to the treatment units; and (ii) selecting a subset of comparison units similar to the treatment unit is difficult because units must be compared across a high-dimensional set of pretreatment characteristics.In normal Matching we match on single characteristics that distinguish treatment and control groups (to try to make them more alike). But If the two groups do not have substantial overlap, then substantial error may be introduced: E.g., if only the worst cases from the untreated “comparison” group are compared to only the best cases from the treatment group, the result may be regression toward the mean which may make the comparison group look better
or worse than reality.
PSM employs a predicted probability of group membership e.g., treatment vs. control group—based on observed predictors, usually obtained from logistic regression to create a counterfactual group. Also propensity score
Propensity score
In the design of experiments, a propensity score is the probability of a unit being assigned to a particular condition in a study given a set of known covariates...
s may be used for matching or as covariates—alone or with other matching variables or covariates.
History
In 1983, Paul Rosenbaum and Donald RubinDonald Rubin
Donald Bruce Rubin is the John L. Loeb Professor of Statistics at Harvard University. He was hired by Harvard in 1984, and served as chair of the department from 1985-1994....
published their seminal paper that first proposed this approach.
General procedure
1.Run logistic regression:- Dependent variable: Y = 1, if participate; Y = 0, otherwise.
- Choose appropriate conditioning (instrumental) variables.
- Obtain propensity score: predicted probability (p) or log[p/(1 − p)].
2.Match each participant to one or more nonparticipants on propensity score:
- Nearest neighbor matchingNearest neighbor searchNearest neighbor search , also known as proximity search, similarity search or closest point search, is an optimization problem for finding closest points in metric spaces. The problem is: given a set S of points in a metric space M and a query point q ∈ M, find the closest point in S to q...
- Caliper matching
- Mahalanobis metricMahalanobis distanceIn statistics, Mahalanobis distance is a distance measure introduced by P. C. Mahalanobis in 1936. It is based on correlations between variables by which different patterns can be identified and analyzed. It gauges similarity of an unknown sample set to a known one. It differs from Euclidean...
matching in conjunction with PSM - Stratification matching
- Difference-in-differences matching (kernel and local linear weights)
3.Multivariate analysis based on new sample
- Use analyses appropriate for non-independent matched samples
Requirements for a good PSM
- Identify treatment and comparison groups with substantial overlap
- Use a composite variable—e.g., a propensity scorePropensity scoreIn the design of experiments, a propensity score is the probability of a unit being assigned to a particular condition in a study given a set of known covariates...
—which reduces group differences across the covariates.
Disadvantages
Howard Bloom, MDRC, sees PSM as a somewhat improved version of simple matching, but with many of the same limitations- Inclusion of propensity scores can help reduce large biases, but significant biases may remain
- Local comparison groups are best—PSM is no miracle maker (it cannot match unmeasured contextual variables)
- Short-term biases (2 years) are substantially less than medium term (3 to 5 year) biases—the value of comparison groups may deteriorate
Michael Sosin, University of Chicago also identifies following problems with PSM:
- Strong assumption that untreated cases were not treated at random
- Argues for using multiple methods and not relying on PSM
Shadish, Cook, & Campbell (2002) identifies further shortcomings with PSM:
- Large samples are required
- Group overlap must be substantial
- Hidden bias may remain because matching only controls for observed variables (to the extent that they are perfectly measured)
Pearl (2009) alerts to the following phenomena with PSM, as well as any matching method:
- Hidden bias may actually increase because matching on observed variables may unleash bias due to dormant unobserved confounders.
- Bias reduction can only be assured (asymptotically) by modeling the qualitative causal relationships between treatment, outcome, observed and unobserved covariates.
In general risks of PSM include:
- They may undermine the argument for experimental designs—an argument that is hard enough to make,
- They may be used to act “as if” a panel survey is an experimental design, overestimating the certainty of findings based on the PSM.
External links
- Introduction to Propensity Score Matching: A New Device for Program Evaluation. Lecture notes 2004
- Implementing Propensity Score Matching Estimators with STATA. Lecture notes 2001