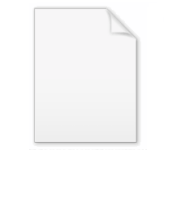
NETtalk (artificial neural network)
Encyclopedia
NETtalk is an artificial neural network
. It is the result of research carried out in the mid 1980s by Terrence Sejnowski and Charles Rosenberg. The intent behind NETtalk was to construct simplified models that might shed light on the complexity of learning human level cognitive tasks, and their implementation as a connectionist model that could also learn to perform a comparable task.
NETtalk is a program that learns to pronounce written English text by being shown text as input and matching phonetic transcriptions for comparison.
To those that do not rigorously study neural networks and their limitations, it would appear to be artificial intelligence in the truest sense of the word. Claims have been printed in the past by some misinformed authors of NETtalk learning to read at the level of a 4 year old human, in about 16 hours! Such a claim, while not an outright lie, is an example of misunderstanding what human brains do when they read, and what NETtalk is capable of learning. Being able to read and pronounce text is not the same as actually comprehending what is being read and understanding in terms of actual imagery and knowledge representation, and this is a key difference between a human child learning to read and an experimental neural network such as NETtalk. In other words, being able to pronounce "grandmother" is not the same as knowing who or what a grandmother is, and how she relates to your immediate family, or what she looks like. NETtalk does not specifically address human-level knowledge representation or its complexities.
NETtalk was created to explore the mechanisms of learning to correctly pronounce English text. The authors note that learning to read involves a complex mechanism involving many parts of the human brain. NETtalk does not specifically model the image processing stages and letter recognition of the visual cortex. Rather, it assumes that the letters have been pre-classified and recognized, and these letter sequences comprising words are then shown to the neural network during training and during performance testing. It is NETtalk's task to learn proper associations between the correct pronunciation with a given sequence of letters based on the context in which the letters appear. In other words NETtalk learns to use the letters around the currently pronounced phoneme that provide cues as to its intended phonemic mapping.
Artificial neural network
An artificial neural network , usually called neural network , is a mathematical model or computational model that is inspired by the structure and/or functional aspects of biological neural networks. A neural network consists of an interconnected group of artificial neurons, and it processes...
. It is the result of research carried out in the mid 1980s by Terrence Sejnowski and Charles Rosenberg. The intent behind NETtalk was to construct simplified models that might shed light on the complexity of learning human level cognitive tasks, and their implementation as a connectionist model that could also learn to perform a comparable task.
NETtalk is a program that learns to pronounce written English text by being shown text as input and matching phonetic transcriptions for comparison.
Achievements and limitations
It is a particularly fascinating neural network because hearing the audio examples of the neural network as it progresses through training seems to progress from a baby babbling to what sounds like a young child reading a kindergarten text, making the occasional mistake, but clearly demonstrating that it has learned the major rules of reading.To those that do not rigorously study neural networks and their limitations, it would appear to be artificial intelligence in the truest sense of the word. Claims have been printed in the past by some misinformed authors of NETtalk learning to read at the level of a 4 year old human, in about 16 hours! Such a claim, while not an outright lie, is an example of misunderstanding what human brains do when they read, and what NETtalk is capable of learning. Being able to read and pronounce text is not the same as actually comprehending what is being read and understanding in terms of actual imagery and knowledge representation, and this is a key difference between a human child learning to read and an experimental neural network such as NETtalk. In other words, being able to pronounce "grandmother" is not the same as knowing who or what a grandmother is, and how she relates to your immediate family, or what she looks like. NETtalk does not specifically address human-level knowledge representation or its complexities.
NETtalk was created to explore the mechanisms of learning to correctly pronounce English text. The authors note that learning to read involves a complex mechanism involving many parts of the human brain. NETtalk does not specifically model the image processing stages and letter recognition of the visual cortex. Rather, it assumes that the letters have been pre-classified and recognized, and these letter sequences comprising words are then shown to the neural network during training and during performance testing. It is NETtalk's task to learn proper associations between the correct pronunciation with a given sequence of letters based on the context in which the letters appear. In other words NETtalk learns to use the letters around the currently pronounced phoneme that provide cues as to its intended phonemic mapping.