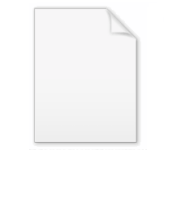
Marginal model
Encyclopedia
In statistics
, marginal models (Heagerty & Zeger, 2000) are a technique for obtaining regression estimates in multilevel model
ing, also called hierarchical linear models.
People often want to know the effect of a predictor/explanatory variable X, on a response variable Y. One way to get an estimate for such effects is through regression analysis
.
for the response variable (
). In a marginal model, we collapse over the level 1 & 2 residuals and thus marginalize (see also conditional probability
) the joint distribution into a univariate normal distribution. We then fit the marginal model to data.
For example, for the following hierarchical model,
Thus, the marginal model is,

This model is what is used to fit to data in order to get regression estimates.
Statistics
Statistics is the study of the collection, organization, analysis, and interpretation of data. It deals with all aspects of this, including the planning of data collection in terms of the design of surveys and experiments....
, marginal models (Heagerty & Zeger, 2000) are a technique for obtaining regression estimates in multilevel model
Multilevel model
Multilevel models are statistical models of parameters that vary at more than one level...
ing, also called hierarchical linear models.
People often want to know the effect of a predictor/explanatory variable X, on a response variable Y. One way to get an estimate for such effects is through regression analysis
Regression analysis
In statistics, regression analysis includes many techniques for modeling and analyzing several variables, when the focus is on the relationship between a dependent variable and one or more independent variables...
.
Why the name marginal model?
In a typical multilevel model, there are level 1 & 2 residuals (R and U variables). The two variables form a joint distributionJoint distribution
In the study of probability, given two random variables X and Y that are defined on the same probability space, the joint distribution for X and Y defines the probability of events defined in terms of both X and Y...
for the response variable (

Conditional probability
In probability theory, the "conditional probability of A given B" is the probability of A if B is known to occur. It is commonly notated P, and sometimes P_B. P can be visualised as the probability of event A when the sample space is restricted to event B...
) the joint distribution into a univariate normal distribution. We then fit the marginal model to data.
For example, for the following hierarchical model,
- level 1:
, the residual is
, and
- level 2:
, the residual is
, and
Thus, the marginal model is,

This model is what is used to fit to data in order to get regression estimates.