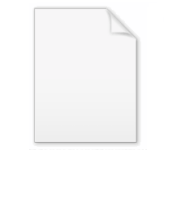
Hidden semi-Markov model
Encyclopedia
A hidden semi-Markov model (HSMM) is a statistical model with the same structure as a hidden Markov model
except that the unobservable process is semi-Markov
rather than Markov
. This means that the probability of there being a change in the hidden state depends on the amount of time that has elapsed since entry into the current state. This is in contrast to hidden Markov models where there is a constant probability of changing state given survival in the state up to that time. For instance Sansom et al. modelled daily rainfall using a hidden semi-Markov model. If the underlying process (e.g. weather system) does not have a geometrically distributed duration, an HSMM may be more appropriate. Statistical inference for hidden semi-Markov models is more difficult than in hidden Markov models, since algorithms like the Baum-Welch algorithm
are not directly applicable, and must be adapted requiring more resources.
Hidden Markov model
A hidden Markov model is a statistical Markov model in which the system being modeled is assumed to be a Markov process with unobserved states. An HMM can be considered as the simplest dynamic Bayesian network. The mathematics behind the HMM was developed by L. E...
except that the unobservable process is semi-Markov
Semi-Markov process
A continuous-time stochastic process is called a semi-Markov process or 'Markov renewal process' if the embedded jump chain is a Markov chain, and where the holding times are random variables with any distribution, whose distribution function may depend on the two states between which the move is...
rather than Markov
Markov process
In probability theory and statistics, a Markov process, named after the Russian mathematician Andrey Markov, is a time-varying random phenomenon for which a specific property holds...
. This means that the probability of there being a change in the hidden state depends on the amount of time that has elapsed since entry into the current state. This is in contrast to hidden Markov models where there is a constant probability of changing state given survival in the state up to that time. For instance Sansom et al. modelled daily rainfall using a hidden semi-Markov model. If the underlying process (e.g. weather system) does not have a geometrically distributed duration, an HSMM may be more appropriate. Statistical inference for hidden semi-Markov models is more difficult than in hidden Markov models, since algorithms like the Baum-Welch algorithm
Baum-Welch algorithm
In electrical engineering, computer science, statistical computing and bioinformatics, the Baum–Welch algorithm is used to find the unknown parameters of a hidden Markov model . It makes use of the forward-backward algorithm and is named for Leonard E. Baum and Lloyd R...
are not directly applicable, and must be adapted requiring more resources.