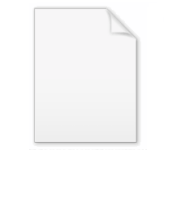
Distributed lag
Encyclopedia
In statistics
and econometrics
, a distributed lag model is a model for time series
data in which a regression
equation is used to predict current values of a dependent variable based on both the current values of an explanatory variable and the lagged (past period) values of this explanatory variable.
The starting point for a distributed lag model is an assumed structure of the form

or the form

where yt is the value at time period t of the dependent variable y, a is the intercept term to be estimated, and wi is called the lag weight (also to be estimated) placed on the value i periods previously of the explanatory variable x. In the first equation, the dependent variable is assumed to be affected by values of the independent variable arbitrarily far in the past, so the number of lag weights is infinite and the model is called an infinite distributed lag model. In the alternative, second, equation, there are only a finite number of lag weights, indicating an assumption that there is a maximum lag beyond which values of the independent variable do not affect the dependent variable; a model based on this assumption is called a finite distributed lag model.
In an infinite distributed lag model, an infinite number of lag weights need to be estimated; clearly this can be done only if some structure is assumed for the relation between the various lag weights, with the entire infinitude of them expressible in terms of a finite number of assumed underlying parameters. In a finite distributed lag model, the parameters could be directly estimated by ordinary least squares
(assuming the number of data points sufficiently exceeds the number of lag weights); nevertheless, such estimation may give very imprecise results due to extreme multicollinearity
among the various lagged values of the independent variable, so again it may be necessary to assume some structure for the relation between the various lag weights.
The concept of distributed lag models easily generalizes to the context of more than one right-side explanatory variable.
, assuming a fixed maximum lag
, assuming independent and identically distributed errors, and imposing no structure on the relationship of the coefficients of the lagged explanators with each other. However, multicollinearity
among the lagged explanators often arises, leading to high variance of the coefficient estimates.
lag model. This model allows the data to determine the shape of the lag structure, but the researcher must specify the maximum lag length; an incorrectly specified maximum lag length can distort the shape of the estimated lag structure as well as the cumulative effect of the independent variable. The Almon lag assumes that k+1 lag weights are related to n+1 linearly estimable underlying parameters (n) aj according to
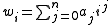
for

Here, if the model has been correctly specified, the value of the coefficient
will be greater than or equal to zero and strictly less than one. In this model, the short-run (same-period) effect of a unit change in the independent variable is the value of b, while the long-run (cumulative) effect of a sustained unit change in the independent variable can be shown to be 
Other infinite distributed lag models have been proposed to allow the data to determine the shape of the lag structure. The polynomial inverse lag assumes that the lag weights are related to underlying, linearly estimable parameters aj according to
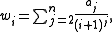
for
The geometric combination lag assumes that the lags weights are related to underlying, linearly estimable parameters aj according to either

for
or

for
The gamma lag and the rational lag are other infinite distributed lag structures.
Statistics
Statistics is the study of the collection, organization, analysis, and interpretation of data. It deals with all aspects of this, including the planning of data collection in terms of the design of surveys and experiments....
and econometrics
Econometrics
Econometrics has been defined as "the application of mathematics and statistical methods to economic data" and described as the branch of economics "that aims to give empirical content to economic relations." More precisely, it is "the quantitative analysis of actual economic phenomena based on...
, a distributed lag model is a model for time series
Time series
In statistics, signal processing, econometrics and mathematical finance, a time series is a sequence of data points, measured typically at successive times spaced at uniform time intervals. Examples of time series are the daily closing value of the Dow Jones index or the annual flow volume of the...
data in which a regression
Linear regression
In statistics, linear regression is an approach to modeling the relationship between a scalar variable y and one or more explanatory variables denoted X. The case of one explanatory variable is called simple regression...
equation is used to predict current values of a dependent variable based on both the current values of an explanatory variable and the lagged (past period) values of this explanatory variable.
The starting point for a distributed lag model is an assumed structure of the form

or the form

where yt is the value at time period t of the dependent variable y, a is the intercept term to be estimated, and wi is called the lag weight (also to be estimated) placed on the value i periods previously of the explanatory variable x. In the first equation, the dependent variable is assumed to be affected by values of the independent variable arbitrarily far in the past, so the number of lag weights is infinite and the model is called an infinite distributed lag model. In the alternative, second, equation, there are only a finite number of lag weights, indicating an assumption that there is a maximum lag beyond which values of the independent variable do not affect the dependent variable; a model based on this assumption is called a finite distributed lag model.
In an infinite distributed lag model, an infinite number of lag weights need to be estimated; clearly this can be done only if some structure is assumed for the relation between the various lag weights, with the entire infinitude of them expressible in terms of a finite number of assumed underlying parameters. In a finite distributed lag model, the parameters could be directly estimated by ordinary least squares
Ordinary least squares
In statistics, ordinary least squares or linear least squares is a method for estimating the unknown parameters in a linear regression model. This method minimizes the sum of squared vertical distances between the observed responses in the dataset and the responses predicted by the linear...
(assuming the number of data points sufficiently exceeds the number of lag weights); nevertheless, such estimation may give very imprecise results due to extreme multicollinearity
Multicollinearity
Multicollinearity is a statistical phenomenon in which two or more predictor variables in a multiple regression model are highly correlated. In this situation the coefficient estimates may change erratically in response to small changes in the model or the data...
among the various lagged values of the independent variable, so again it may be necessary to assume some structure for the relation between the various lag weights.
The concept of distributed lag models easily generalizes to the context of more than one right-side explanatory variable.
Unstructured estimation
The simplest way to estimate parameters associated with distributed lags is by ordinary least squaresOrdinary least squares
In statistics, ordinary least squares or linear least squares is a method for estimating the unknown parameters in a linear regression model. This method minimizes the sum of squared vertical distances between the observed responses in the dataset and the responses predicted by the linear...
, assuming a fixed maximum lag

Multicollinearity
Multicollinearity is a statistical phenomenon in which two or more predictor variables in a multiple regression model are highly correlated. In this situation the coefficient estimates may change erratically in response to small changes in the model or the data...
among the lagged explanators often arises, leading to high variance of the coefficient estimates.
Structured estimation
Structured distributed lag models come in two types: finite and infinite. Infinite distributed lags allow the value of the independent variable at a particular time to influence the dependent variable infinitely far into the future, or to put it another way, they allow the current value of the dependent variable to be influenced by values of the independent variable that occurred infinitely long ago; but beyond some lag length the effects taper off toward zero. Finite distributed lags allow for the independent variable at a particular time to influence the dependent variable for only a finite number of periods.Finite distributed lags
The most important structured finite distributed lag model is the AlmonShirley Montag Almon
Shirley Montag Almon is an economist noted for the Almon Lag. She was educated at Goucher College Baltimore and then for her PhD at Harvard . A core element of her PhD was published in Econometrica and introduced the now famous technique for estimating distributed lags...
lag model. This model allows the data to determine the shape of the lag structure, but the researcher must specify the maximum lag length; an incorrectly specified maximum lag length can distort the shape of the estimated lag structure as well as the cumulative effect of the independent variable. The Almon lag assumes that k+1 lag weights are related to n+1 linearly estimable underlying parameters (n
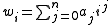
for

Infinite distributed lags
The most common type of structured infinite distributed lag model is the geometric lag, also known as the Koyck lag. In this lag structure, the weights (magnitudes of influence) of the lagged independent variable values decline exponentially with the length of the lag; while the shape of the lag structure is thus fully imposed by the choice of this technique, the rate of decline as well as the overall magnitude of effect are determined by the data. Specification of the regression equation is very straightforward: one includes as explanators (right-hand side variables in the regression) the one-period-lagged value of the dependent variable and the current value of the independent variable:
Here, if the model has been correctly specified, the value of the coefficient


Other infinite distributed lag models have been proposed to allow the data to determine the shape of the lag structure. The polynomial inverse lag assumes that the lag weights are related to underlying, linearly estimable parameters aj according to
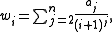
for

The geometric combination lag assumes that the lags weights are related to underlying, linearly estimable parameters aj according to either

for


for

The gamma lag and the rational lag are other infinite distributed lag structures.